Endowing Pre-trained Graph Models with Provable Fairness
CoRR(2024)
摘要
Pre-trained graph models (PGMs) aim to capture transferable inherent
structural properties and apply them to different downstream tasks. Similar to
pre-trained language models, PGMs also inherit biases from human society,
resulting in discriminatory behavior in downstream applications. The debiasing
process of existing fair methods is generally coupled with parameter
optimization of GNNs. However, different downstream tasks may be associated
with different sensitive attributes in reality, directly employing existing
methods to improve the fairness of PGMs is inflexible and inefficient.
Moreover, most of them lack a theoretical guarantee, i.e., provable lower
bounds on the fairness of model predictions, which directly provides assurance
in a practical scenario. To overcome these limitations, we propose a novel
adapter-tuning framework that endows pre-trained Graph models with
Provable fAiRness (called GraphPAR). GraphPAR
freezes the parameters of PGMs and trains a parameter-efficient adapter to
flexibly improve the fairness of PGMs in downstream tasks. Specifically, we
design a sensitive semantic augmenter on node representations, to extend the
node representations with different sensitive attribute semantics for each
node. The extended representations will be used to further train an adapter, to
prevent the propagation of sensitive attribute semantics from PGMs to task
predictions. Furthermore, with GraphPAR, we quantify whether the fairness of
each node is provable, i.e., predictions are always fair within a certain range
of sensitive attribute semantics. Experimental evaluations on real-world
datasets demonstrate that GraphPAR achieves state-of-the-art prediction
performance and fairness on node classification task. Furthermore, based on our
GraphPAR, around 90% nodes have provable fairness.
更多查看译文
AI 理解论文
溯源树
样例
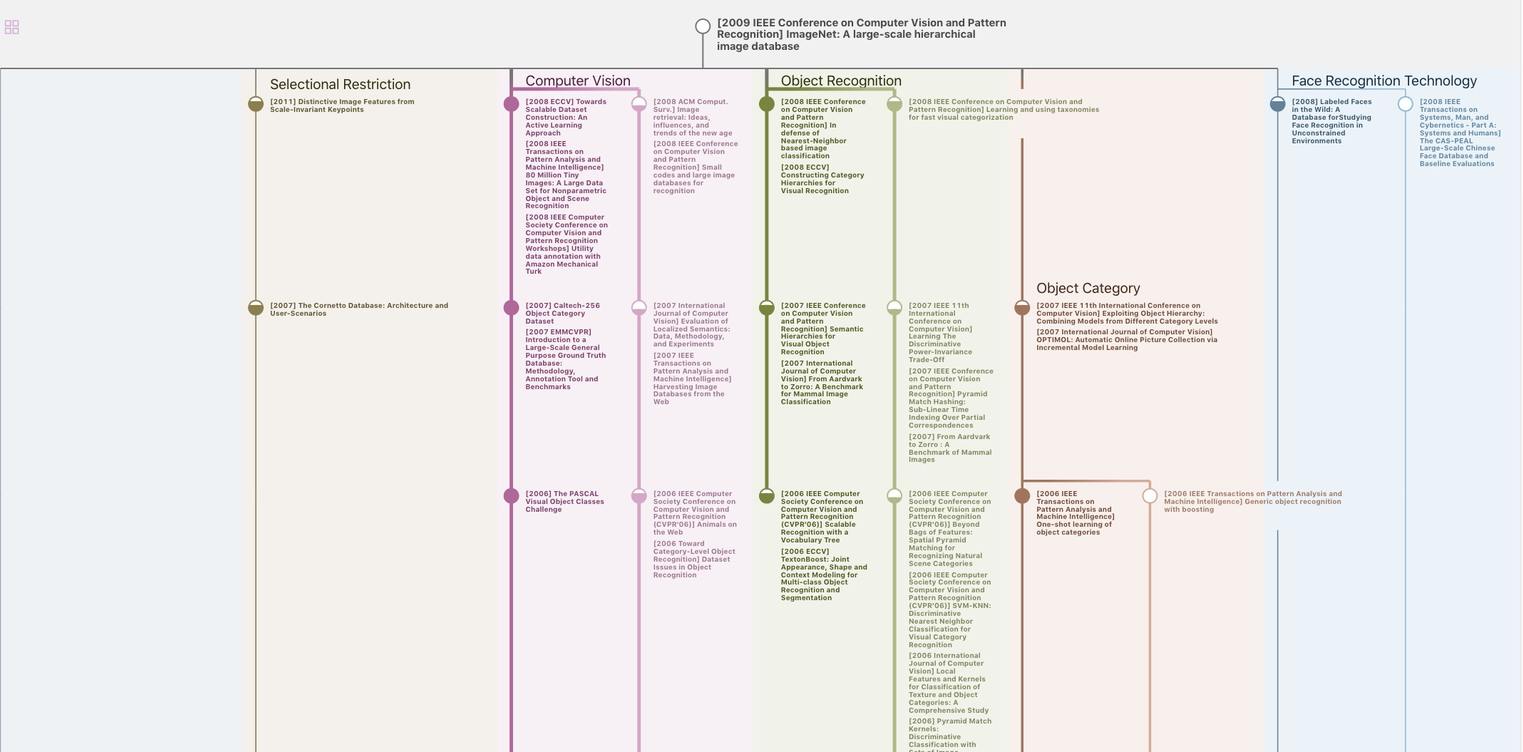
生成溯源树,研究论文发展脉络
Chat Paper
正在生成论文摘要