U^2MRPD: Unsupervised undersampled MRI reconstruction by prompting a large latent diffusion model
arxiv(2024)
摘要
Implicit visual knowledge in a large latent diffusion model (LLDM)
pre-trained on natural images is rich and hypothetically universal to natural
and medical images. To test this hypothesis, we introduce a novel framework for
Unsupervised Undersampled MRI Reconstruction by Prompting a pre-trained large
latent Diffusion model ( U^2MRPD). Existing data-driven, supervised
undersampled MRI reconstruction networks are typically of limited
generalizability and adaptability toward diverse data acquisition scenarios;
yet U^2MRPD supports image-specific MRI reconstruction by prompting an LLDM
with an MRSampler tailored for complex-valued MRI images. With any
single-source or diverse-source MRI dataset, U^2MRPD's performance is further
boosted by an MRAdapter while keeping the generative image priors intact.
Experiments on multiple datasets show that U^2MRPD achieves comparable or
better performance than supervised and MRI diffusion methods on in-domain
datasets while demonstrating the best generalizability on out-of-domain
datasets. To the best of our knowledge, U^2MRPD is the first
unsupervised method that demonstrates the universal prowess of a LLDM,
on magnitude-only natural images in medical imaging, attaining the best
adaptability for both MRI database-free and database-available scenarios and
generalizability towards out-of-domain data.
更多查看译文
AI 理解论文
溯源树
样例
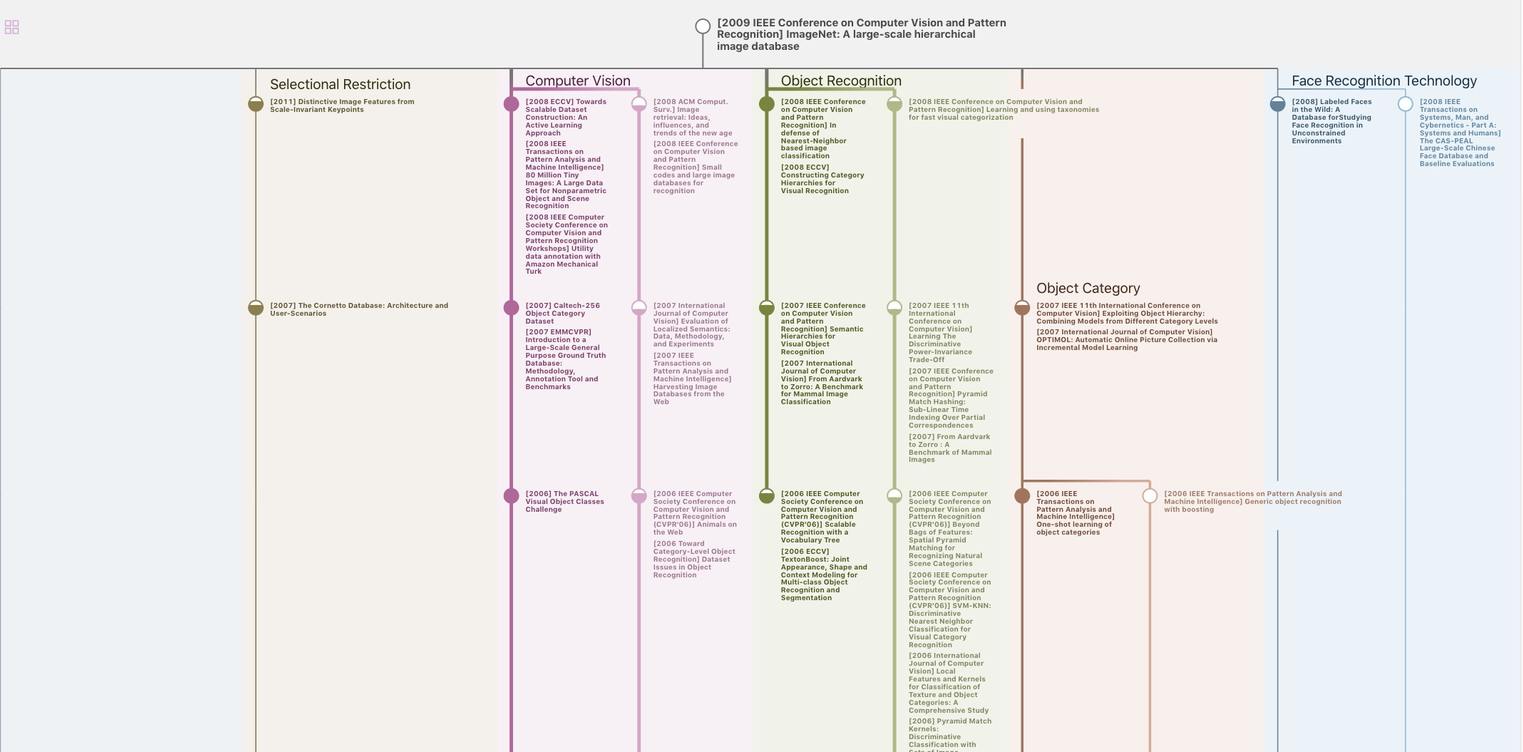
生成溯源树,研究论文发展脉络
Chat Paper
正在生成论文摘要