Understanding In-Context Learning with a Pelican Soup Framework
CoRR(2024)
摘要
Many existing theoretical analyses of in-context learning for natural
language processing are based on latent variable models that leaves gaps
between theory and practice. We aim to close these gaps by proposing a
theoretical framework, the Pelican Soup Framework. In this framework, we
introduce (1) the notion of a common sense knowledge base, (2) a general
formalism for natural language classification tasks, and the notion of (3)
meaning association. Under this framework, we can establish a
𝒪(1/T) loss bound for in-context learning, where T is the number
of example-label pairs in the demonstration. Compared with previous works, our
bound reflects the effect of the choice of verbalizers and the effect of
instruction tuning. An additional notion of atom concepts makes our
framework possible to explain the generalization to tasks unseen in the
language model training data. Finally, we propose a toy setup, Calcutec, and a
digit addition task that mimics types of distribution shifts a model needs to
overcome to perform in-context learning. We also experiment with GPT2-Large on
real-world NLP tasks. Our empirical results demonstrate the efficacy of our
framework to explain in-context learning.
更多查看译文
AI 理解论文
溯源树
样例
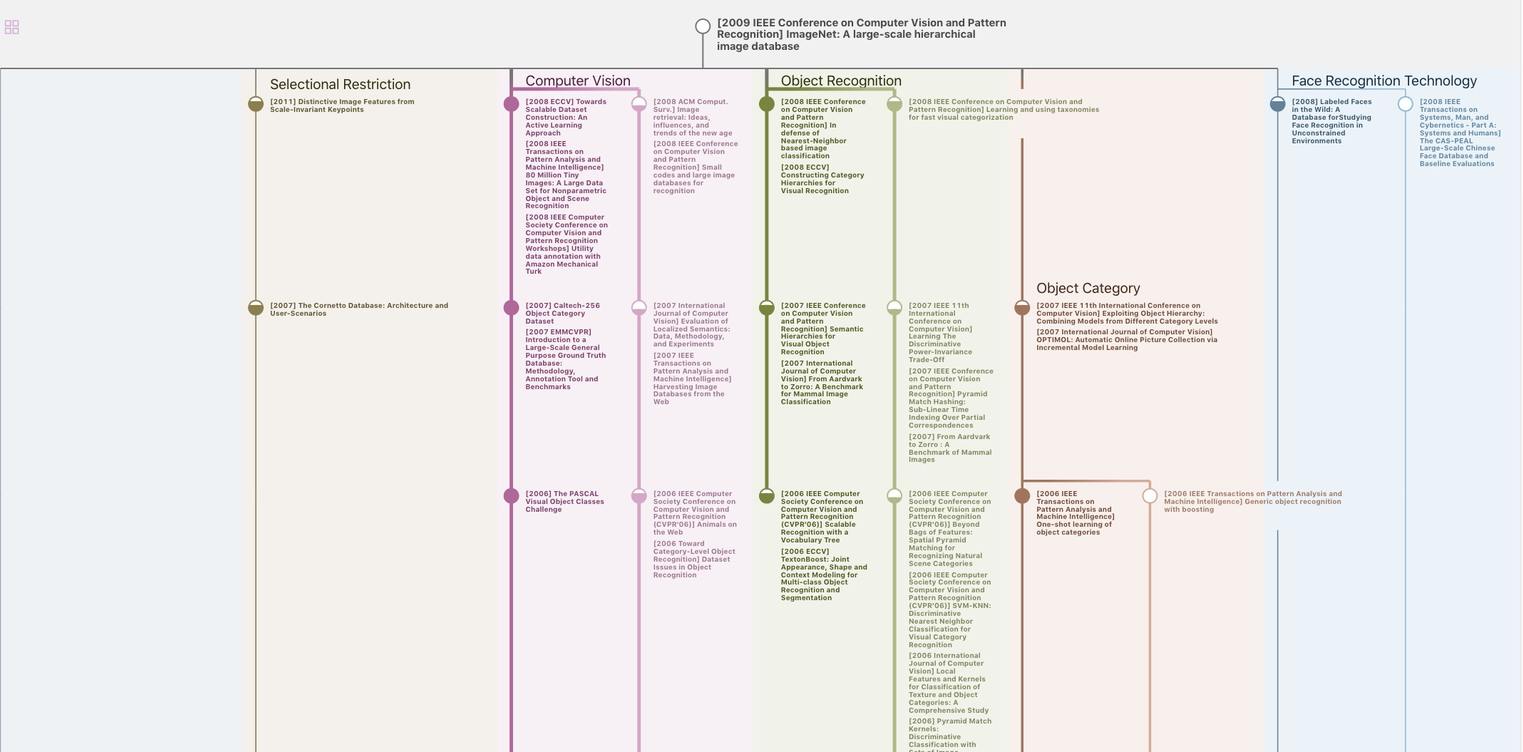
生成溯源树,研究论文发展脉络
Chat Paper
正在生成论文摘要