Construction of machine learning models based on transrectal ultrasound combined with contrast-enhanced ultrasound to predict preoperative regional lymph node metastasis of rectal cancer
HELIYON(2024)
摘要
Purpose: Constructing a machine learning model based on transrectal ultrasound (TRUS) combined with contrast-enhanced ultrasound (CEUS) to predict preoperative regional lymph node metastasis (RLNM) of rectal cancer and provide new references for decision-making. Materials and methods: 233 patients with rectal cancer were enrolled and underwent TRUS and CEUS prior to surgery. Clinicopathological and ultrasound data were collected to analyze the correlation of RLNM status, clinical features and ultrasound parameters. A 75% training set and 25% test set were utilized to construct seven machine learning algorithms. The DeLong test was used to assess the model's diagnostic performance, then chose the best one to predict RLNM of rectal cancer. Results: The diagnostic performance was most dependent on the following: MMT difference (36), length (30), location (29), AUC ratio (27), and PI ratio (24). The prediction accuracy, sensitivity, specificity, precision, and F1 score range of KNN, Bayes, MLP, LR, SVM, RF, and LightGBM were (0.553-0.857), (0.000-0.935), (0.600-1.000), (0.557-0.952), and (0.617-0.852), respectively. The LightGBM model exhibited the optimal accuracy (0.857) and F1 score (0.852). The AUC for machine learning analytics were (0.517-0.941, 95% CI: 0.380-0.986). The LightGBM model exhibited the highest AUC (0.941, 95% CI: 0.843-0.986), though no statistic significant showed in comparison with the SVM, LR, RF, and MLP models (P > 0.05), it was significantly higher than that of the KNN and Bayes models (P < 0.05). Conclusion: The LightGBM machine learning model based on TRUS combined with CEUS may help predict RLNM prior to surgery and provide new references for clinical treatment in rectal cancer.
更多查看译文
关键词
Rectal cancer,Regional lymph node metastasis,Transrectal ultrasound,Contrast-enhanced ultrasound,Machine learning,Predictive model
AI 理解论文
溯源树
样例
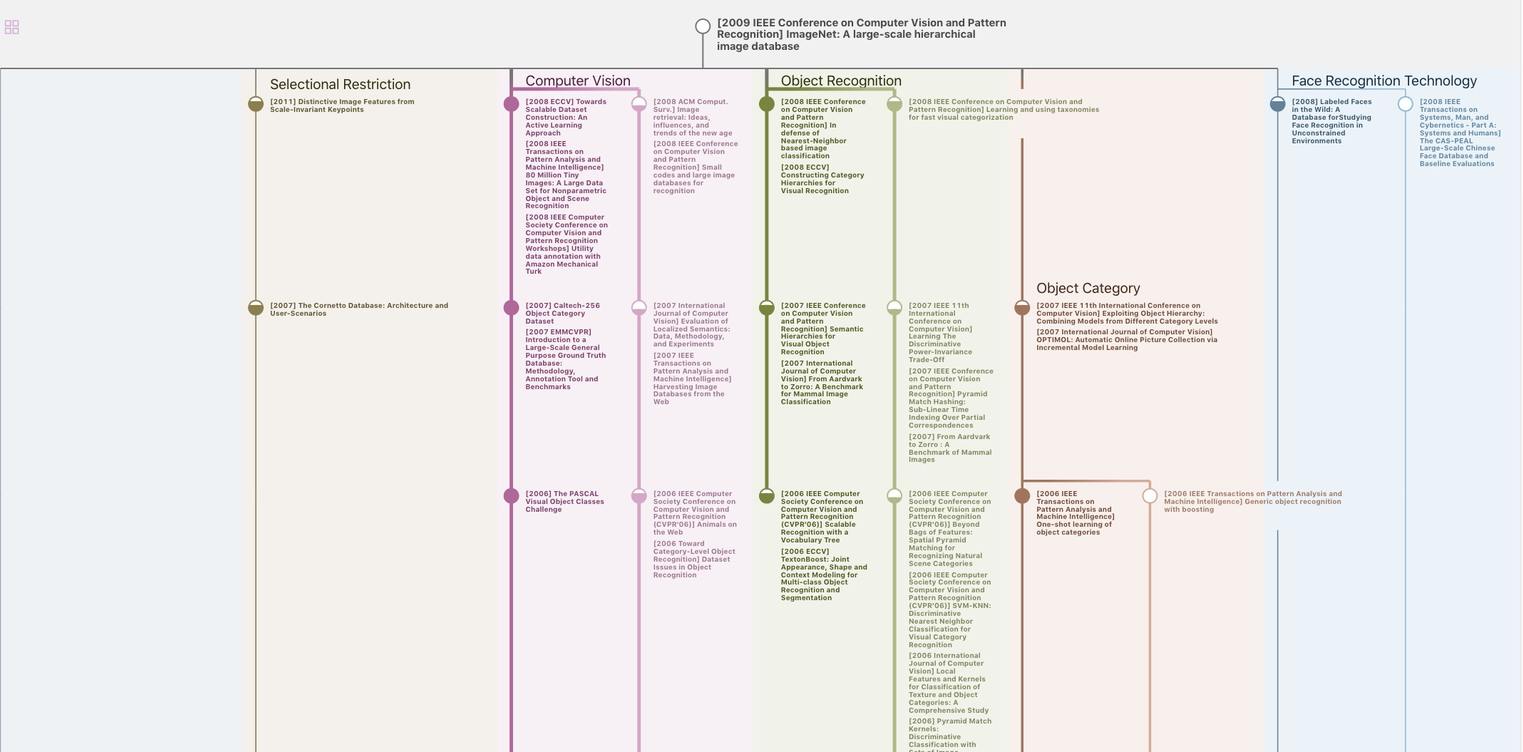
生成溯源树,研究论文发展脉络
Chat Paper
正在生成论文摘要