Towards an Efficient Federated Learning Framework with Selective Aggregation.
International Conference on Communication Systems and Networks(2024)
摘要
Federated Learning shows promise for collaborative, decentralized machine learning but faces efficiency challenges, primarily network straggler-induced latency bottlenecks and the need for complex aggregation techniques. To address these issues, ongoing research explores asynchronous FL, i.e., federated learning models, including an Asynchronous Parallel Federated Learning [5] framework. This study investigates the impact of varying worker node numbers on key metrics. More nodes offer faster convergence but may increase communication overhead and straggler vulnerability. We aim to quantify how the number of worker node variations for one global aggregation can affect convergence speed, communication efficiency, model accuracy, and system robustness, optimizing asynchronous FL system configurations. This work is crucial for practical and scalable FL applications, mitigating network stragglers, data distribution, and security challenges. This work analyses Asynchronous Parallel Federated Learning and showcases a paradigm shift in the approach by selectively aggregating early arriving worker node updates with a novel parameter ‘x’, improving efficiency and reshaping FL.
更多查看译文
关键词
Federated Learning,Federated Learning Framework,Machine Learning,Data Distribution,Worker Nodes,Federated Learning Model,Accurate Identification,Global Model,Visual Representation,Data Privacy,Real-world Applications,Real-world Scenarios,Training Efficiency,Central Server,Local Training,Edge Devices,Node Selection,Local Updates,Aggregation Operators,Heterogeneous Devices,Collaborative Training
AI 理解论文
溯源树
样例
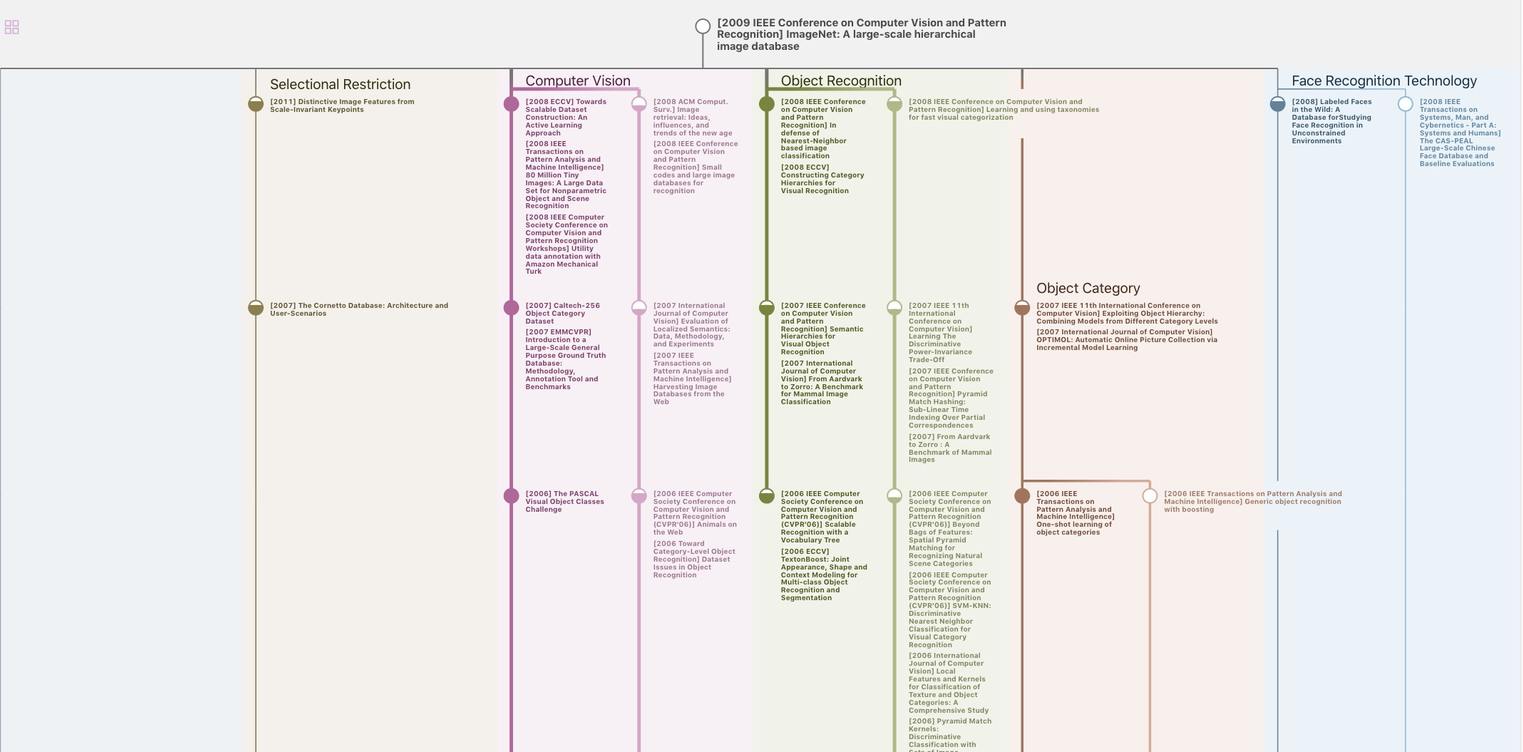
生成溯源树,研究论文发展脉络
Chat Paper
正在生成论文摘要