Human-Centered Explanations: Lessons Learned from Image Classification for Medical and Clinical Decision Making
KI - Künstliche Intelligenz(2024)
摘要
To date, there is no universal explanatory method for making decisions of an AI-based system transparent to human decision makers. This is because, depending on the application domain, data modality, and classification model, the requirements for the expressiveness of explanations vary. Explainees, whether experts or novices (e.g., in medical and clinical diagnosis) or developers, have different information needs. To address the explanation gap, we motivate human-centered explanations and demonstrate the need for combined and expressive approaches based on two image classification use cases: digital pathology and clinical pain detection using facial expressions. Various explanatory approaches that have emerged or been applied in the three-year research project “Transparent Medical Expert Companion” are shortly reviewed and categorized in expressiveness according to their modality and scope. Their suitability for different contexts of explanation is assessed with regard to the explainees’ need for information. The article highlights open challenges and suggests future directions for integrative explanation frameworks.
更多查看译文
关键词
Explainable Artificial Intelligence,Digital healthcare,Human-centered explanations,Explanation expressiveness,Explanation frameworks
AI 理解论文
溯源树
样例
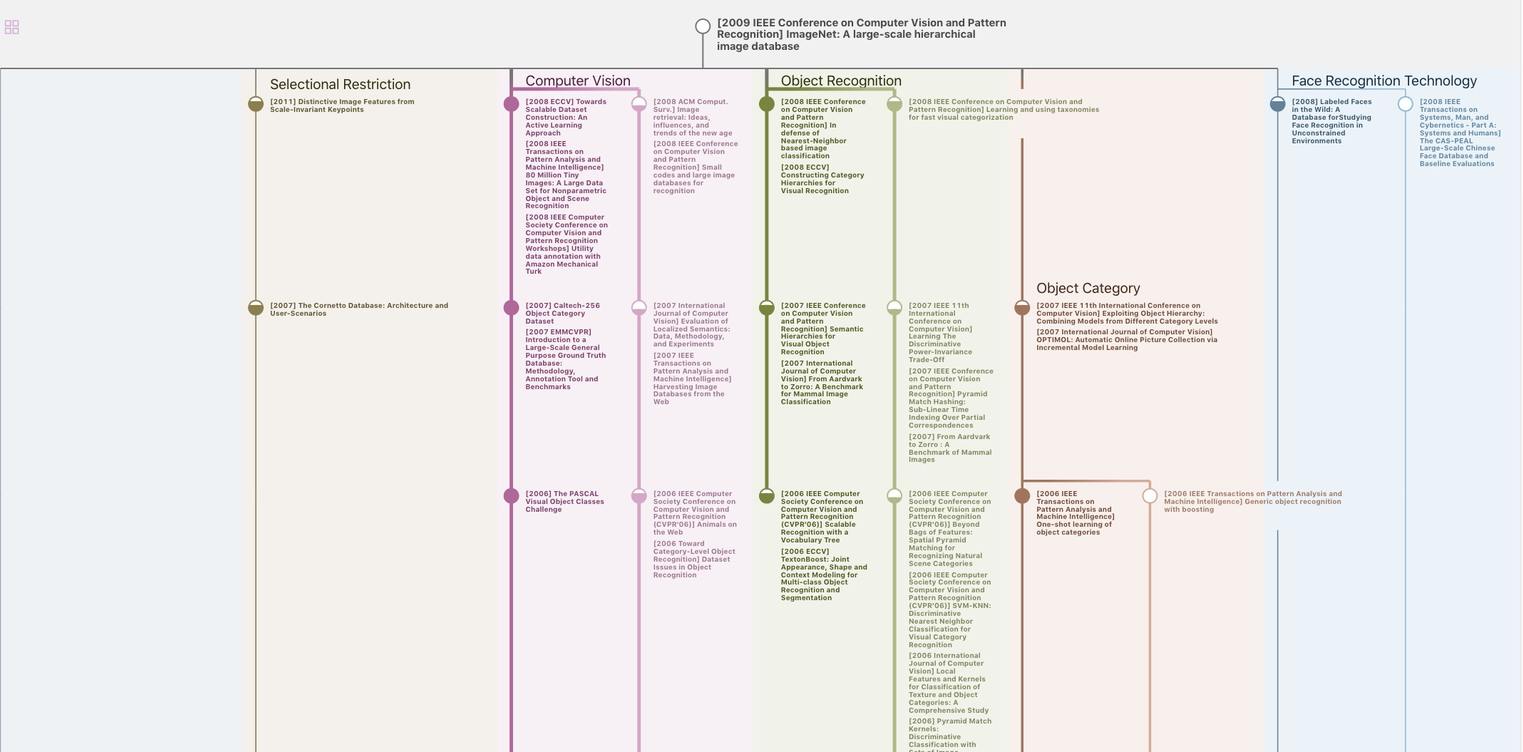
生成溯源树,研究论文发展脉络
Chat Paper
正在生成论文摘要