Traffic Flow Forecasting Based on Transformer with Diffusion Graph Attention Network
International Journal of Automotive Technology(2024)
摘要
Because of the high nonlinearity and complexity, it is still a challenge to forecast traffic flow accurately. Most of the existing methods, which lack the ability to model the dynamic spatio-temporal correlations of traffic flows, rely on a priori knowledge of the graph structure to capture static spatial correlations. However, spatial correlation can be dynamic and the physical structure does not reflect the true relationship between roads. To better capture complex spatio-temporal dependencies and predict traffic conditions on road networks, a new forecasting model based on Transformer and Diffusion Graph Attention Network (T-DGAN) is proposed. This model adopts the Transformer encoder-decoder architecture, where the encoder and decoder contain multiple Spatio-Temporal Convolutional Network Blocks (ST-CB) and Diffusion Graph Attention Blocks (DGA-B), which are utilized to capture the spatio-temporal dependency and the dynamic spatial correlation of traffic flow separately. In addition, an information auxiliary module is added to the decoder to summarize the traffic flow information between the encoder and the decoder. Experiments on different datasets verify that the T-DGAN model has achieved higher forecasting performance than the baseline methods. In PEMS03, the Mean Absolute Error (MAE) and Root Mean Square Error (RMSE) of T-DGAN to 60 min are improved by 4.28% and 3.66%, compared with the state-of-the-art model, respectively.
更多查看译文
关键词
Traffic forecasting,Transformer,Spatio-temporal correlation,Deep learning
AI 理解论文
溯源树
样例
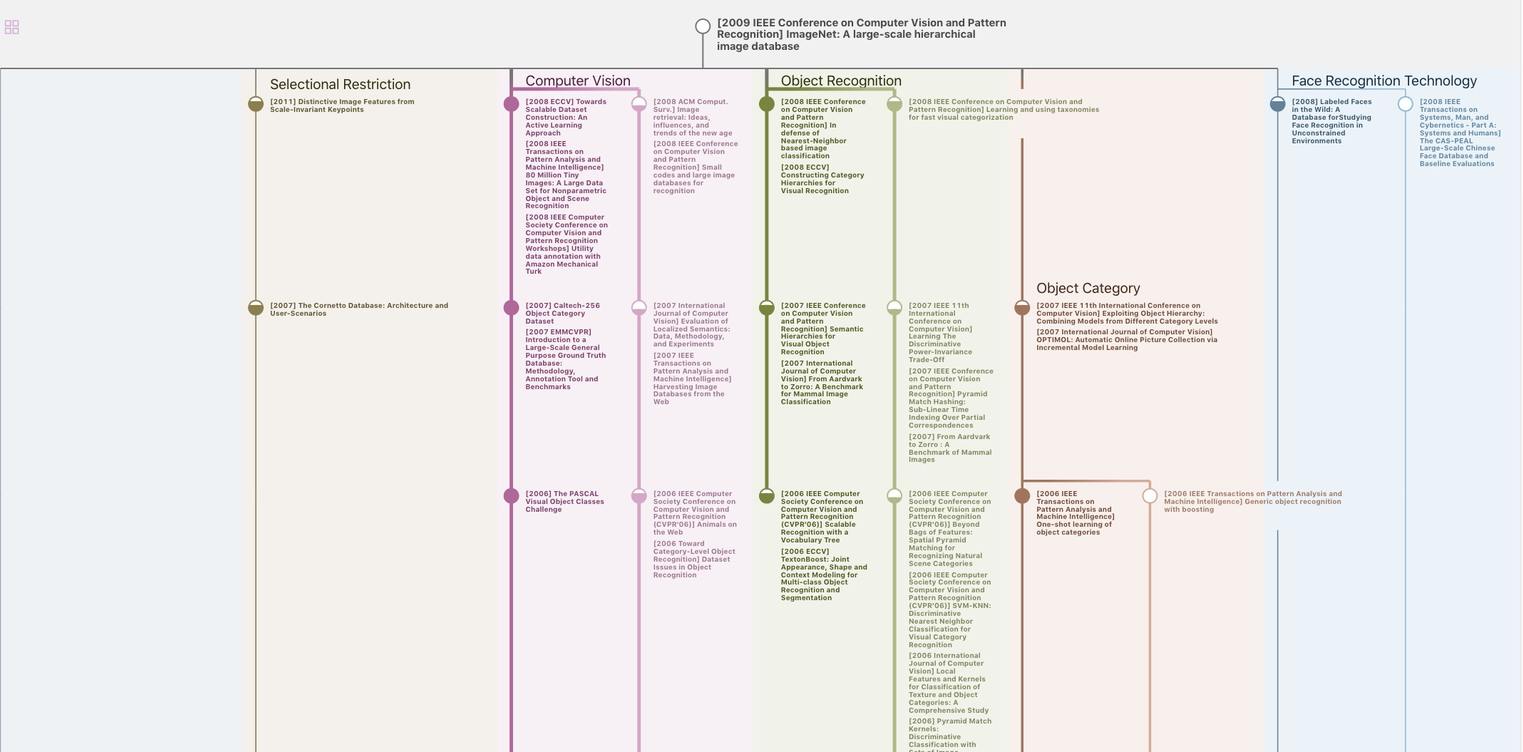
生成溯源树,研究论文发展脉络
Chat Paper
正在生成论文摘要