Masked feature regeneration based asymmetric student–teacher network for anomaly detection
Multimedia Tools and Applications(2024)
摘要
Anomaly detection (AD), also known as one-class classification and localization, has become a challenging task in the field of industrial defect detection where anomalous samples can hardly be collected to train a network based on conventional computer vision tasks. Knowledge distillation based on a student-teacher (S-T) framework has proved its effectiveness in solving such problems in an unsupervised fashion. In this paper, we propose a novel asymmetric S-T framework with masked feature regeneration called AST-MFR. First, to ensure better feature alignment during the training period, we introduce a masked feature regeneration (MFR) module to mask multi-level features of the student network randomly and regenerate the corresponding features under the guidance of the teacher network’s features. Second, to enlarge the feature diversity of unseen anomalous samples during the test period, we adopt an asymmetric S-T network structure that is sensitive to detecting and locating anomalous parts. We conduct experiments on the industrial anomaly detection benchmark dataset MVTec AD and the results demonstrate the proposed model achieves competitive performance compared to the state-of-the-art methods on both anomaly detection and anomaly localization .
更多查看译文
关键词
Anomaly detection,Knowledge distillation,Unsupervised learning,Masked feature regeneration,Asymmetric student-teacher framework
AI 理解论文
溯源树
样例
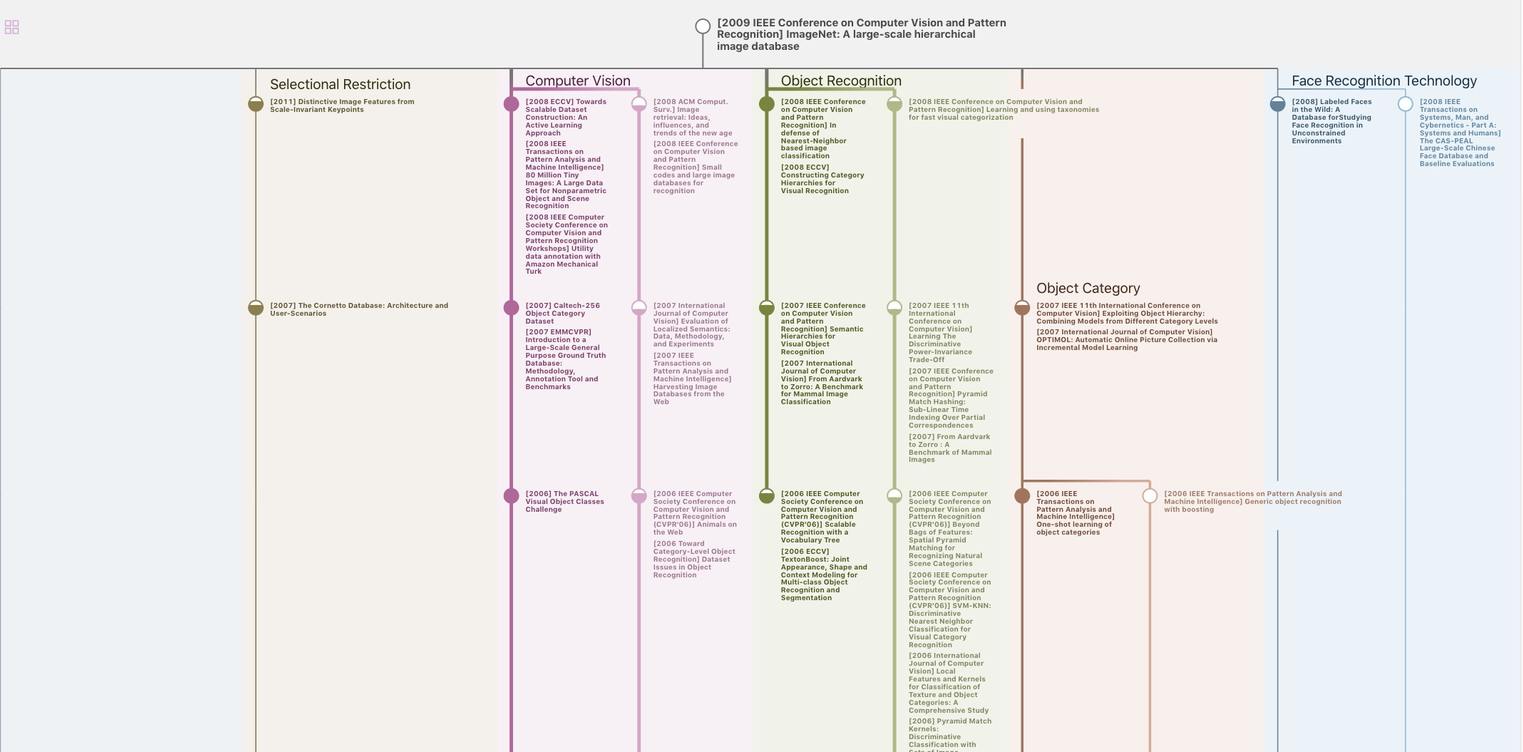
生成溯源树,研究论文发展脉络
Chat Paper
正在生成论文摘要