Accelerated discovery of high-performance 3D printing materials using multi-objective active optimization method
Journal of Materials Science(2024)
摘要
The inadequate performance of available materials substantially hinders the advancement of 3D printing in achieving complex structure manufacturing and realizing customized design. However, the traditional empirical trial-and-error approach is generally optimized for a single objective with high experimental costs. Therefore, we developed an active optimization workflow to accelerate the discovery of high-performance 3D printing materials with minimal experimental costs using the machine learning method. Employing the multi-objective optimization method, the subsequent experimental plan is recommended actively based on the previous experimental results and iterated until the algorithm converges. Within a mere 15 iterations of the algorithm, a set of high-performance materials was discovered, significantly reducing the experiments required to just 90 sets of samples. Furthermore, the discovered materials demonstrated remarkable improvements in various mechanical properties. The hardness increased from 78.3 to 84.5 HD, the bending strength increased from 53.7 to 89.2 MPa, the tensile strength increased from 30.4 to 45.6 MPa, and the elongation at break increased from 4.9 to 13.6%. Crucially, this highly efficient active optimization workflow can be seamlessly adapted for discovering a wide range of high-performance materials with minimal experimental costs.
更多查看译文
AI 理解论文
溯源树
样例
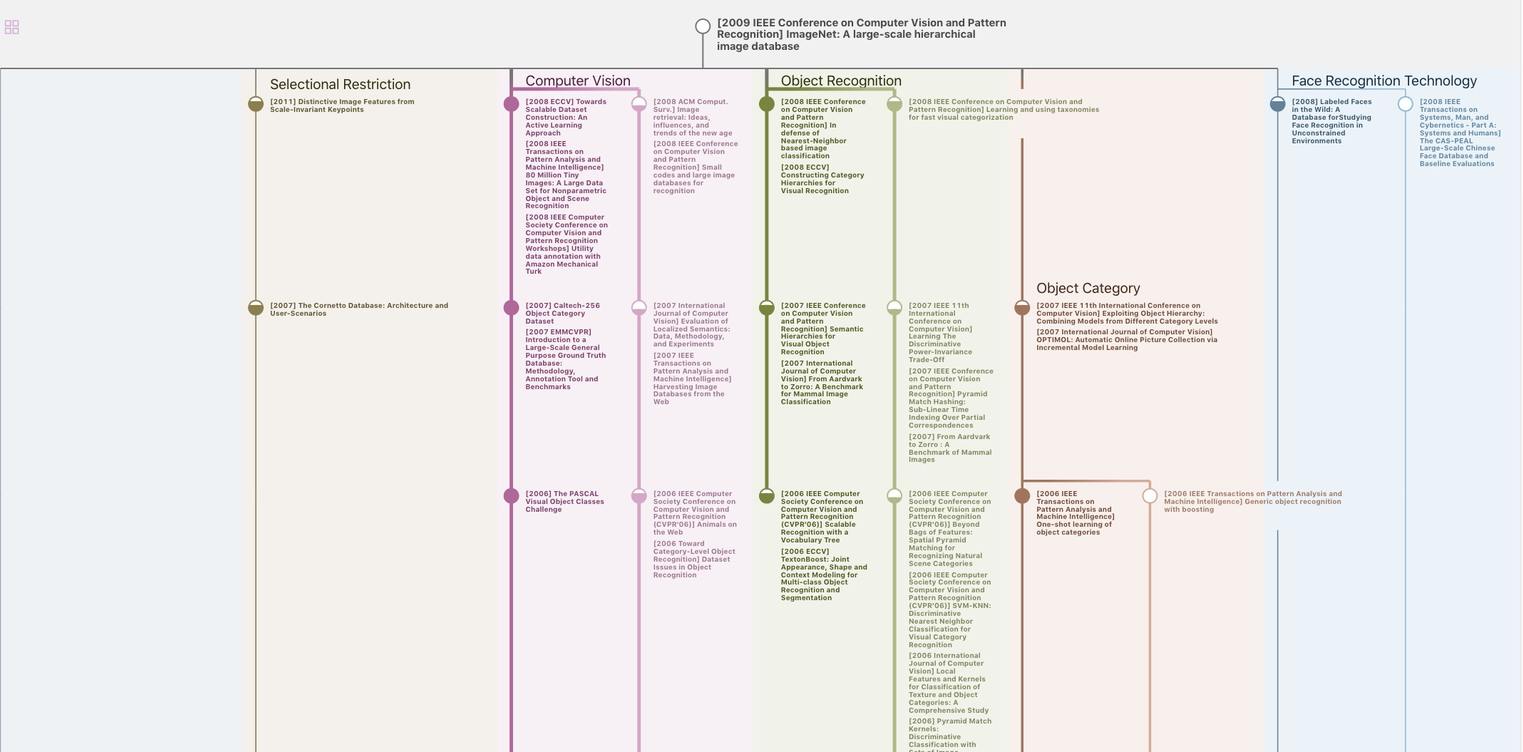
生成溯源树,研究论文发展脉络
Chat Paper
正在生成论文摘要