Augmented Doppler Filter Bank for Enhancing Targets Detection Based on Machine Learning
PRZEGLAD ELEKTROTECHNICZNY(2023)
摘要
Radar Target Detection (RTD) is a critical aspect of modern radar systems that have widespread use in both civil and military fields. However, detecting targets in clutter and unfavorable conditions is challenging with conventional signal processing approaches such as Constant False Alarm Rate (CFAR). The harsh and complex environments in radar measurements make the target detection problem even more challenging when using traditional methods. Therefore, developing a reliable and robust RTD technique is crucial. This paper proposes an approach that incorporates Machine Learning (ML) with conventional methods to detect, separate, and classify real targets from noisy backgrounds in a real radar dataset by employing Fuzzy C-means (FCM) clustering to segment the Range Doppler Map (RDM) image into targets and background, then a feature extraction technique based on gray-level co-occurrence matrix (GLCM) and classify the targets using a support vector machine (SVM). The approach is based on an augmented Doppler Filter Bank (DFB) with RDM images and has been tested on a Frequency Modulated Continuous Wave (FMCW) radar mounted on an Unmanned Aerial Vehicle (UAV) for detecting ground targets. A flight was conducted in a challenging environment to evaluate the proposed system's performance. The experimental results demonstrate that the proposed approach outperforms existing methods in terms of classification accuracy. The proposed approach is also computationally efficient and can be easily implemented in real-time systems and has great potential in improving RTD performance in various applications.
更多查看译文
关键词
doppler filter bank,moving target detector,radar signal processing,fuzzy c-means cluster,support vector machine
AI 理解论文
溯源树
样例
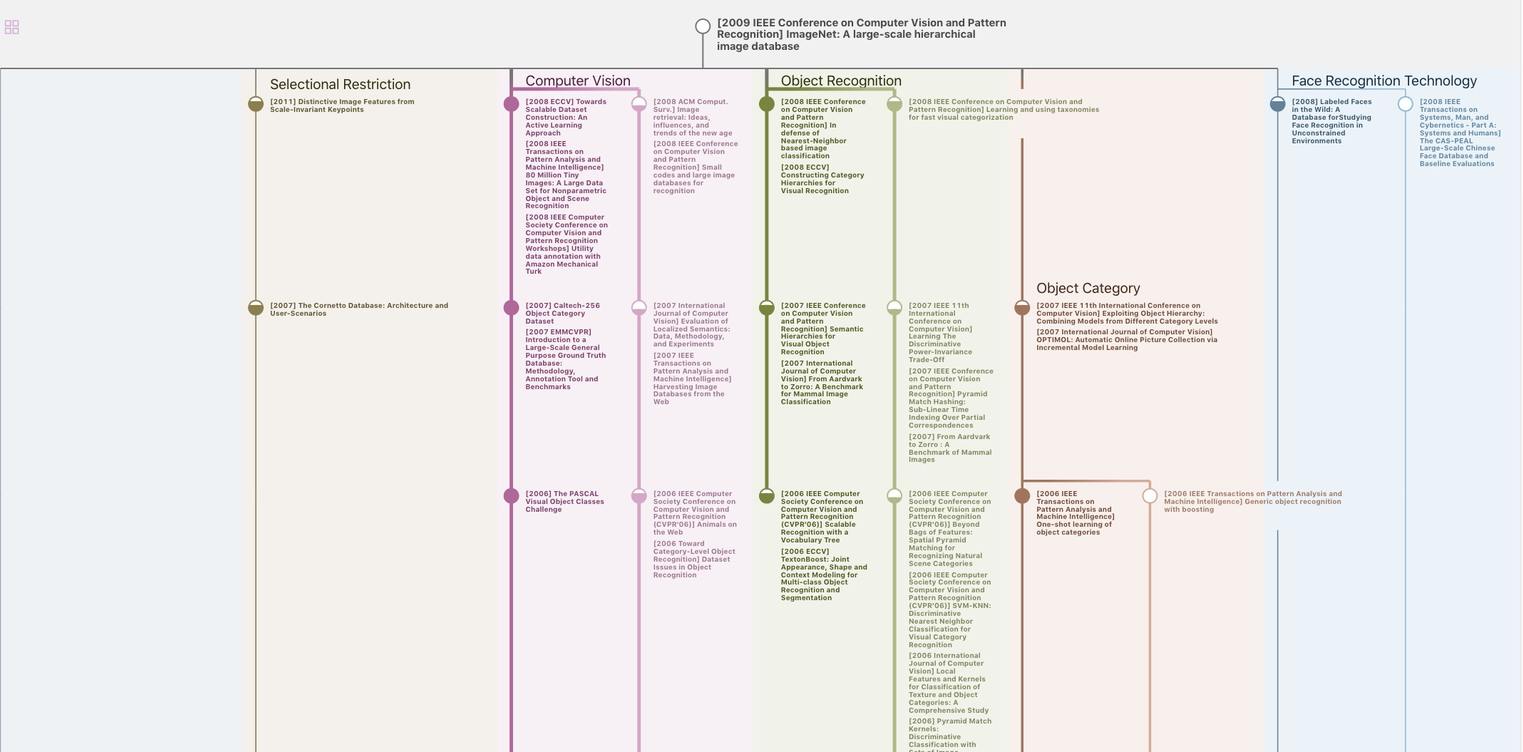
生成溯源树,研究论文发展脉络
Chat Paper
正在生成论文摘要