MarkerNet: A divide-and-conquer solution to motion capture solving from raw markers
COMPUTER ANIMATION AND VIRTUAL WORLDS(2024)
Abstract
Marker-based optical motion capture (MoCap) aims to localize 3D human motions from a sequence of input raw markers. It is widely used to produce physical movements for virtual characters in various games such as the role-playing game, the fighting game, and the action-adventure game. However, the conventional MoCap cleaning and solving process is extremely labor-intensive, time-consuming, and usually the most costly part of game animation production. Thus, there is a high demand for automated algorithms to replace costly manual operations and achieve accurate MoCap cleaning and solving in the game industry. In this article, we design a divide-and-conquer-based MoCap solving network, dubbed MarkerNet, to estimate human skeleton motions from sequential raw markers effectively. In a nutshell, our key idea is to decompose the task of direct solving of global motion from all markers into first modeling sub-motions of local parts from the corresponding marker subsets and then aggregating sub-motions into a global one. In this manner, our model can effectively capture local motion patterns w.r.t. different marker subsets, thus producing more accurate results compared to the existing methods. Extensive experiments on both real and synthetic data verify the effectiveness of the proposed method. The overall motion of a human body can be decomposed into several sub-motions of different local parts. Thus, we divide all sequential markers into different subsets and learn different local sub-motions from the corresponding marker subsets within local spatio-temporal ranges.image
MoreTranslated text
Key words
deep learning,MoCap solving,motion capture,virtual character animation
AI Read Science
Must-Reading Tree
Example
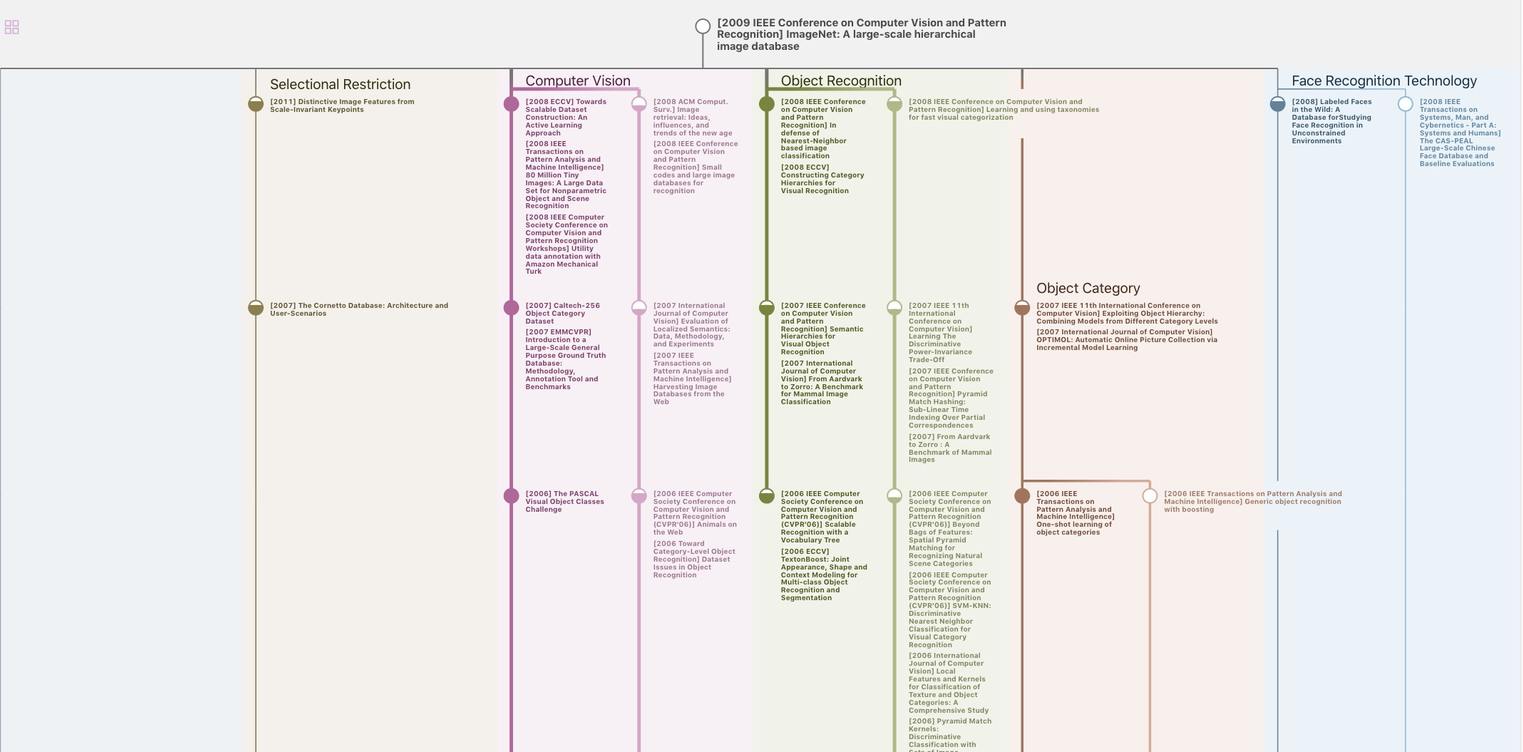
Generate MRT to find the research sequence of this paper
Chat Paper
Summary is being generated by the instructions you defined