BCUIS-Net: A breast cancer ultrasound image segmentation network via boundary-aware and shape feature fusion
INTERNATIONAL JOURNAL OF IMAGING SYSTEMS AND TECHNOLOGY(2024)
摘要
Breast cancer is a highly lethal disease with the highest mortality rate among women worldwide. Breast tumor segmentation from ultrasound images plays a critical role in enabling early detection, leading to a reduction in mortality rates. However, the challenge of ultrasound breast cancer segmentation arises from factors such as indistinct lesion boundaries, noise artifacts, and inhomogeneous intensity distribution within the lesion region. To address the bottlenecks, a novel boundary-aware shape feature fusion network (BCUIS-Net) is proposed to segment breast lesion in ultrasound images. Firstly, a boundary-aware module (BAM) is put forward to accurately localize the ambiguous tumor regions and boundaries by embedding the horizontal and vertical position information into the channel attention. Subsequently, a shape feature fusion (SFF) module is presented to fuse shape features and segmentation features, in order to adaptively extract their complementary features by aggregating contextual information in an attention module. Specifically, the different levels of features from the encoder are up-sampled to the original image size and fed into the BAM to predict the boundary map. The boundary and decoder-generated feature maps are thereafter fused by the SFF module to exploit the complementarity between them to correct errors in segmentation and shape features, effectively eliminating false detections and noise in the features to achieve accurate segmentation of pathological regions. Finally, the shape fusion loss is derived from a combination of the binary cross-entropy loss and the distance map loss to intelligently penalize incorrect predictions and thus improve the attention to boundary locations. The performance of the network is evaluated in two public breast ultrasound datasets. Experimental results verify that the proposed method obtains superior segmentation results and outperforms the most recent state-of-the-art, in which IOU is increased by 2.15% and 2.59% on UDIAT and BUSI, respectively.
更多查看译文
关键词
boundary aware module,breast lesion segmentation,shape feature fusion module,shape fusion loss
AI 理解论文
溯源树
样例
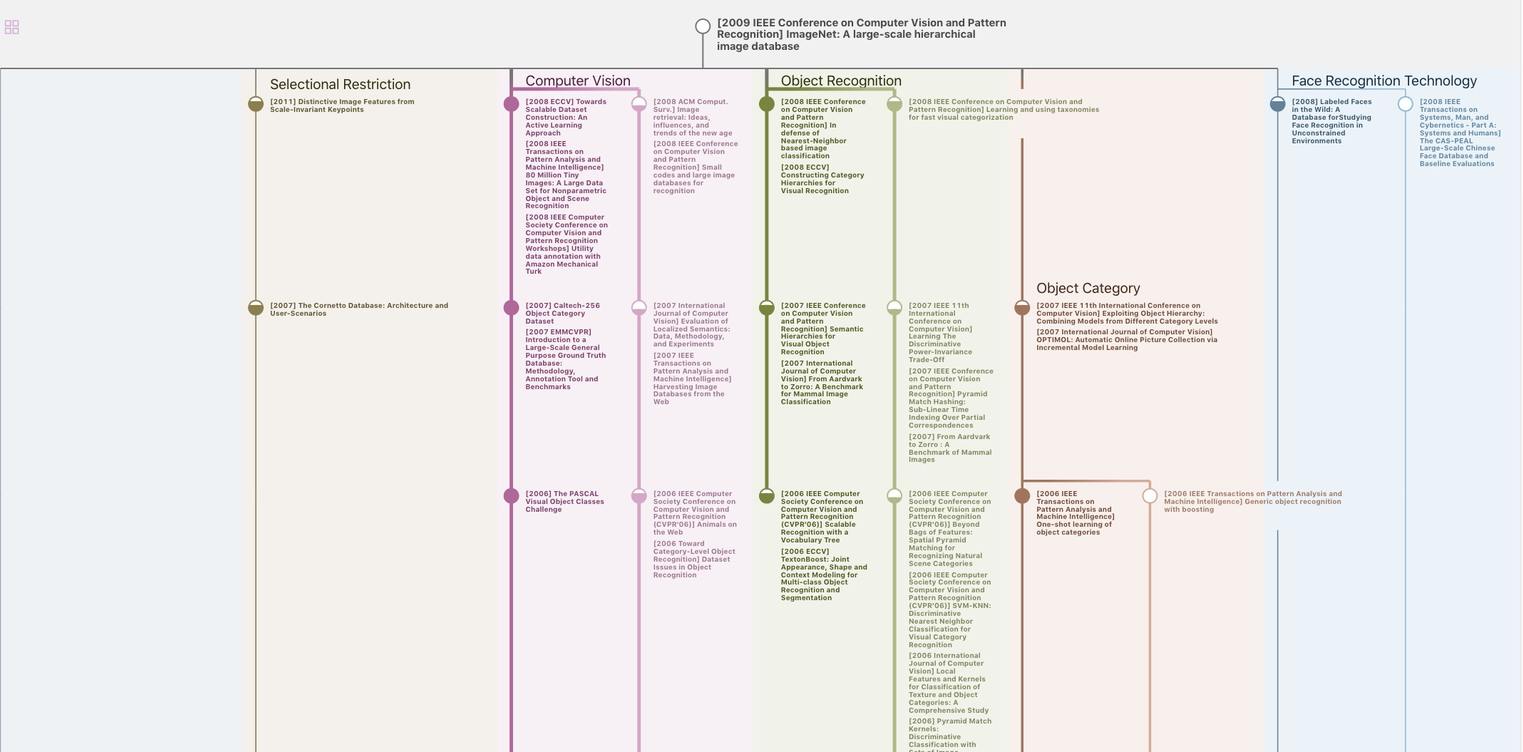
生成溯源树,研究论文发展脉络
Chat Paper
正在生成论文摘要