PLA-A Privacy-Embedded Lightweight and Efficient Automated Breast Cancer Accurate Diagnosis Framework for the Internet of Medical Things
ELECTRONICS(2023)
摘要
The Internet of Medical Things (IoMT) can automate breast tumor detection and classification with the potential of artificial intelligence. However, the leakage of sensitive data can cause harm to patients. To address this issue, this study proposed an intrauterine breast cancer diagnosis method, namely "Privacy-Embedded Lightweight and Efficient Automated (PLA)", for IoMT, which represents an approach that combines privacy-preserving techniques, efficiency, and automation to achieve our goals. Firstly, our model is designed to achieve lightweight classification prediction and global information processing of breast cancer by utilizing an advanced IoMT-friendly ViT backbone. Secondly, PLA protects patients' privacy by federated learning, taking the classification task of breast cancer as the main task and introducing the texture analysis task of breast cancer images as the auxiliary task to train the model. For our PLA framework, the classification accuracy is 0.953, the recall rate is 0.998 for the best, the F1 value is 0.969, the precision value is 0.988, and the classification time is 61.9 ms. The experimental results show that the PLA model performs better than all of the comparison methods in terms of accuracy, with an improvement of more than 0.5%. Furthermore, our proposed model demonstrates significant advantages over the comparison methods regarding time and memory.
更多查看译文
关键词
PLA,privacy-embedded,federated learning,breast cancer classification,Internet of Medical Things,lightweight
AI 理解论文
溯源树
样例
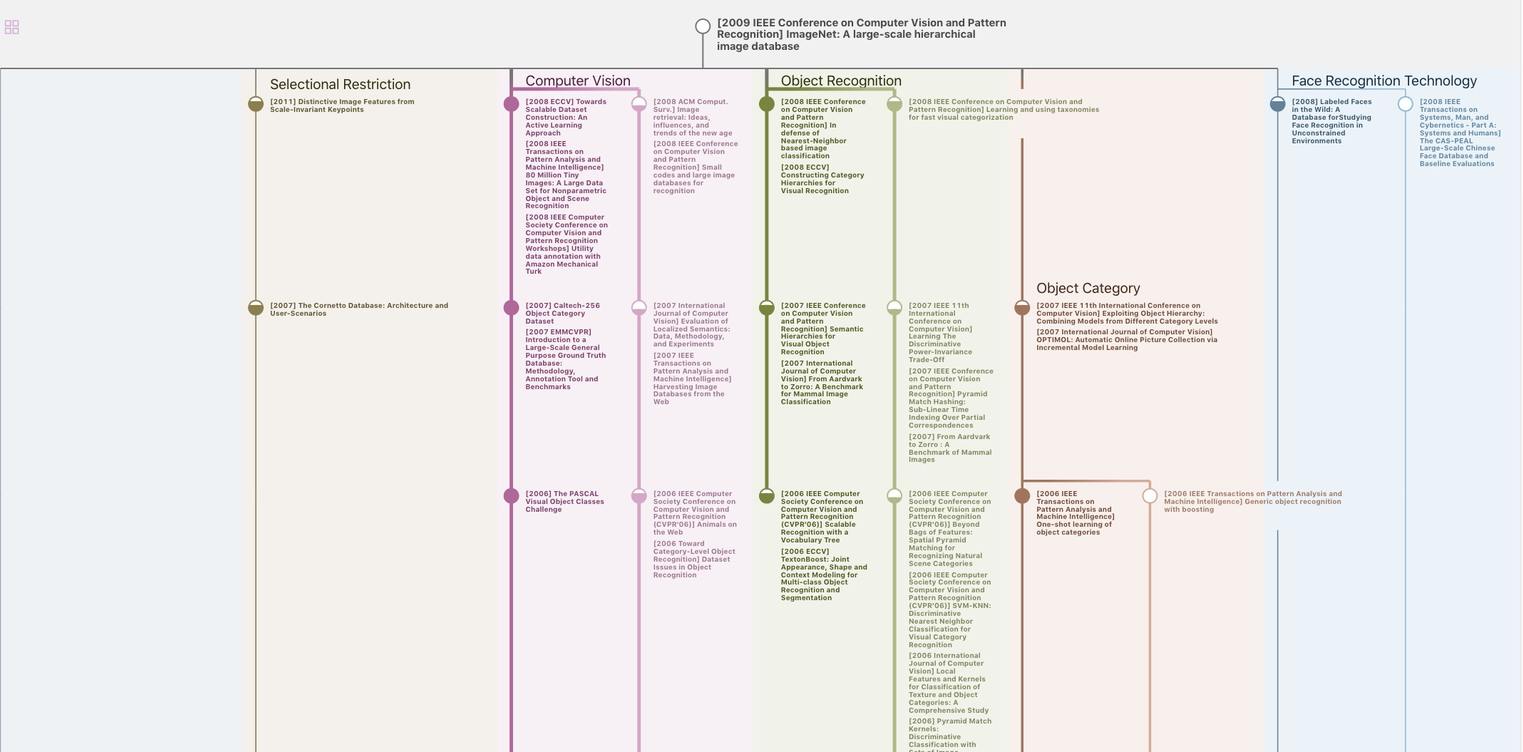
生成溯源树,研究论文发展脉络
Chat Paper
正在生成论文摘要