Searching for massive black hole binaries with a transfer learning algorithm
PHYSICAL REVIEW D(2023)
摘要
Hierarchical mergers in a dense environment are one of the primary formation channels of the intermediate-mass black hole (IMBH) binary system. We expect that the resulting massive binary system will exhibit mass asymmetry. The emitted gravitational wave (GW) carries significant contributions from higher-order modes and hence complex waveform morphology due to the superposition of different modes. Further, IMBH binaries exhibit lower merger frequency and shorter signal duration in the LIGO detector, increasing the risk of them being misclassified as short-duration noisy glitches. Deep learning algorithms can be trained to discriminate noisy glitches from short GW transients. We present THAMES-a deeplearning-based end-to-end signal-detection algorithm for GW signals from quasicircular nearly edge-on, mass asymmetric IMBH binaries in advanced GW detectors. Our study shows that it outperforms matchedfilter based PyCBC searches for higher mass asymmetric, nearly edge-on IMBH binaries. The maximum gain in the sensitive volume-time product for mass ratio q is an element of (5, 10) is by a factor of 5.24 (2.92) against the PyCBC-IMBH (PyCBC-HM) search at a false alarm rate of 1 in 100 years. Compared to the broad PyCBC search, this factor is -100 for the q is an element of (10, 18). One of the reasons for this leap in volumetric sensitivity is its ability to discriminate between signals with complex waveform morphology and noisy transients, clearly demonstrating the potential of deep learning algorithms in probing complex signal morphology in the field of gravitational-wave astronomy. With the current training set, THAMES slightly underperforms with respect to PyCBC-based searches targeting intermediate-mass black hole binaries with mass ratio q is an element of (5, 10) and detector frame total mass MT(1 thorn z) is an element of (100, 200)M circle dot. Because of the independent nature of the search, THAMES offers a follow-up targeted method for asymmetric IMBH systems with higher inclination. This can help verify and potentially enhance the event's statistical significance.
更多查看译文
AI 理解论文
溯源树
样例
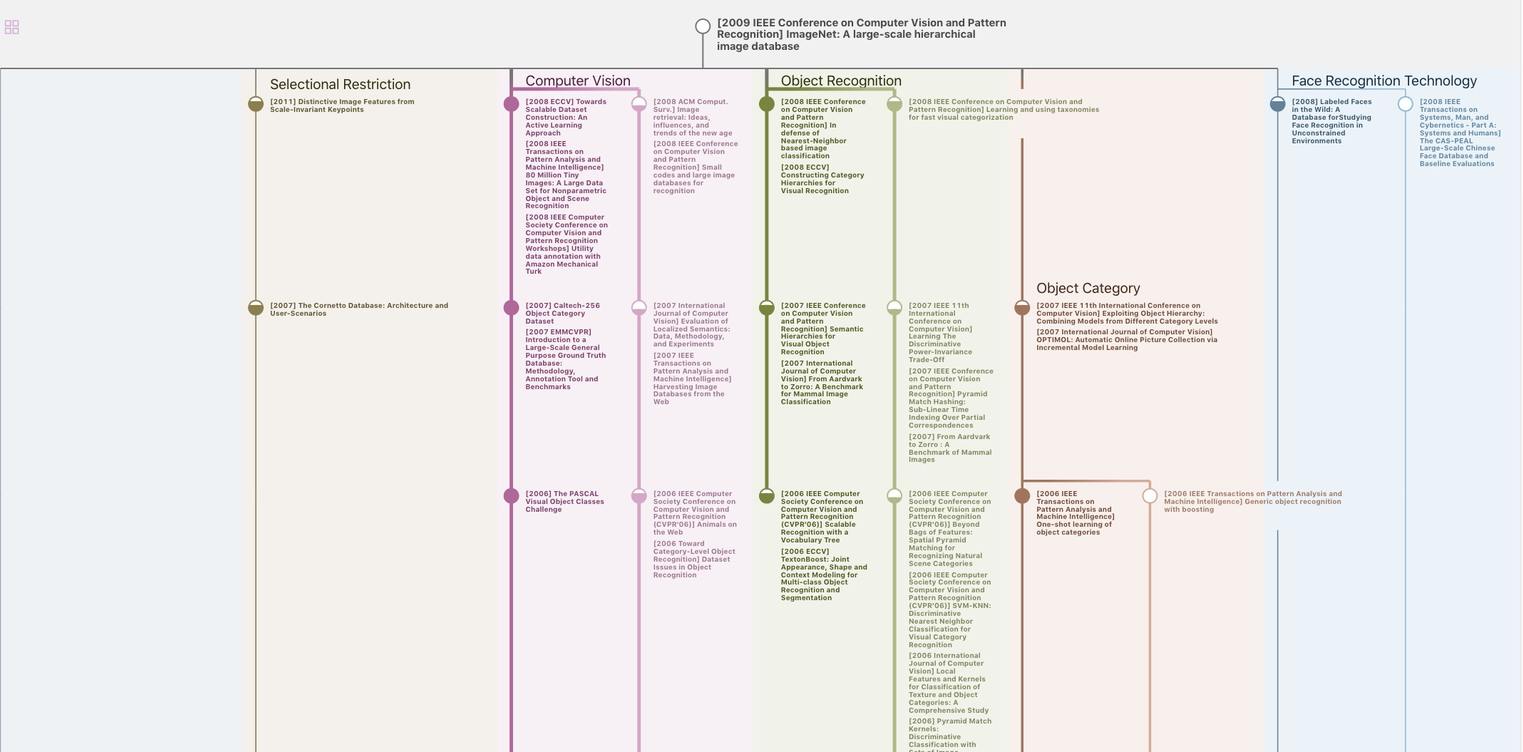
生成溯源树,研究论文发展脉络
Chat Paper
正在生成论文摘要