Ensemble synthetic oversampling with pixel pair for class-imbalanced and small-sized hyperspectral data classification
International Journal of Applied Earth Observation and Geoinformation(2024)
摘要
Hyperspectral images (HSI) suffer from limited labeled data and the curse of dimensionality, which makes it difficult to classify imbalanced and small-sized HSI data. To address the mentioned issues, pixel pair features is designed to handle small-size problem. However, the augmented data inherits the class imbalance and even exhibits a larger imbalance ratio. Therefore, the ensemble-based Synthetic Minority Oversampling Technique (SMOTE) is integrated into pixel pair features to deal with imbalanced datasets and strengthen the quality of the augmented datasets. The proposed method is evaluated on five groups of HSI datasets and compared against seven state-of-the-art contrast algorithms, including ensemble-CNN (E-CNN), CNN with pixel pair features, ensemble CNN with pixel pair features, spatial–spectral E-ResNet, E-Transfering-VGGNet, ContextualNet, and CNN with K-means SMOTE. The proposed method demonstrates superior performance across three distinct difficulty levels, as determined by its average performance rank on five HSI datasets. This underscores its effectiveness in mitigating small size and class imbalance challenges.
更多查看译文
关键词
Hyperspectral image classification,Imbalanced data,Small-sized data,Ensemble learning
AI 理解论文
溯源树
样例
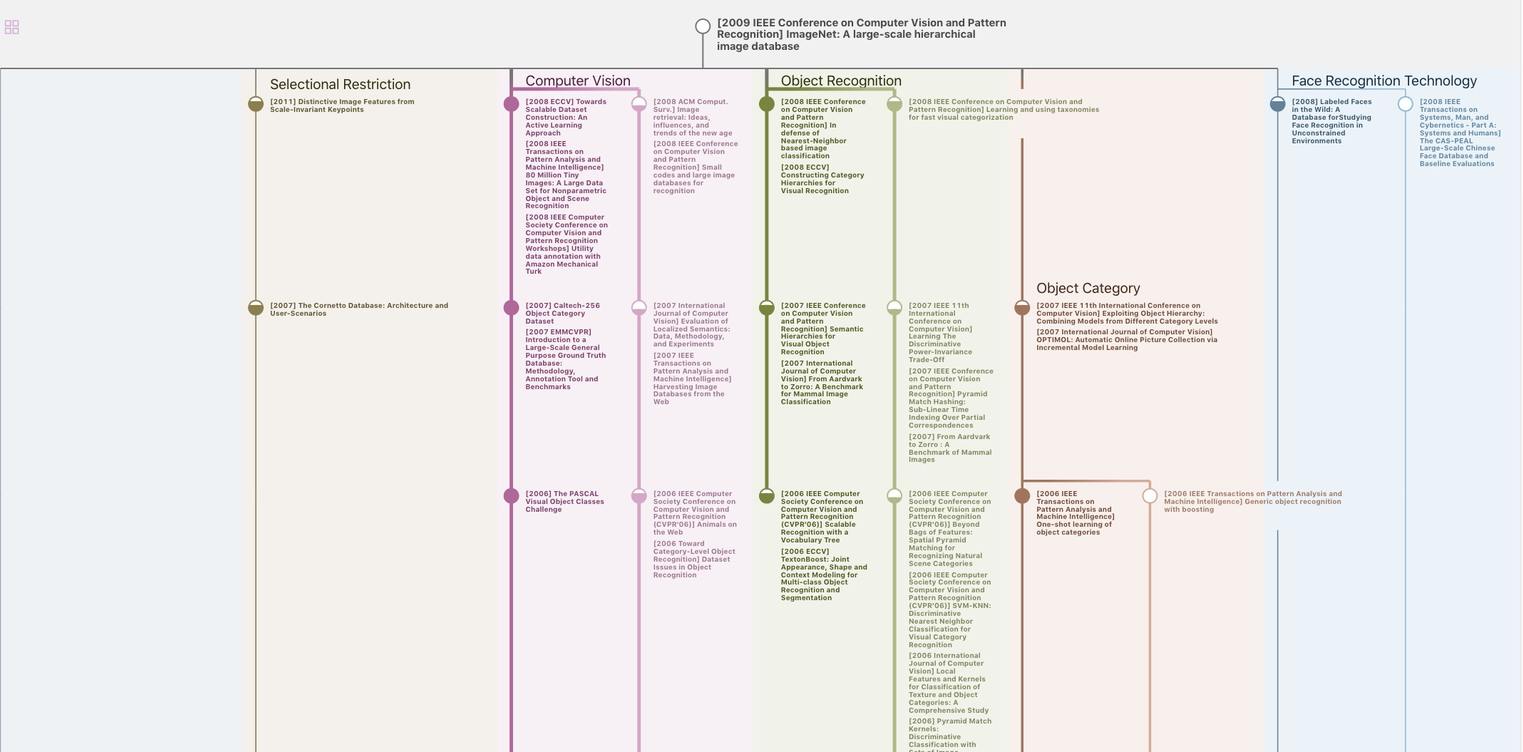
生成溯源树,研究论文发展脉络
Chat Paper
正在生成论文摘要