Motif Interactions Affect Post-Hoc Interpretability of Genomic Convolutional Neural Networks
biorxiv(2024)
摘要
Post-hoc interpretability methods are commonly used to understand decisions of genomic deep learning models and reveal new biological insights. However, interactions between sequence regions (e.g. regulatory elements) impact the learning process as well as interpretability methods that are sensitive to dependencies between features. Since deep learning models learn correlations between the data and output that do not necessarily represent a causal relationship, it is difficult to say how well interacting motif sets are fully captured. Here, we investigate how genomic motif interactions influence model learning and interpretability methods by formalizing possible scenarios where interaction effects appear. This includes the choice of negative data and non-additive effects on the outcome. We generate synthetic data containing interactions for those scenarios and evaluate how they affect the performance of motif detection. We show that post-hoc interpretability methods can miss motifs if interactions are present depending on how negative data is defined. Furthermore, we observe differences in interpretability between additive and non-additive effects as well as between post-hoc interpretability methods.
### Competing Interest Statement
The authors have declared no competing interest.
更多查看译文
AI 理解论文
溯源树
样例
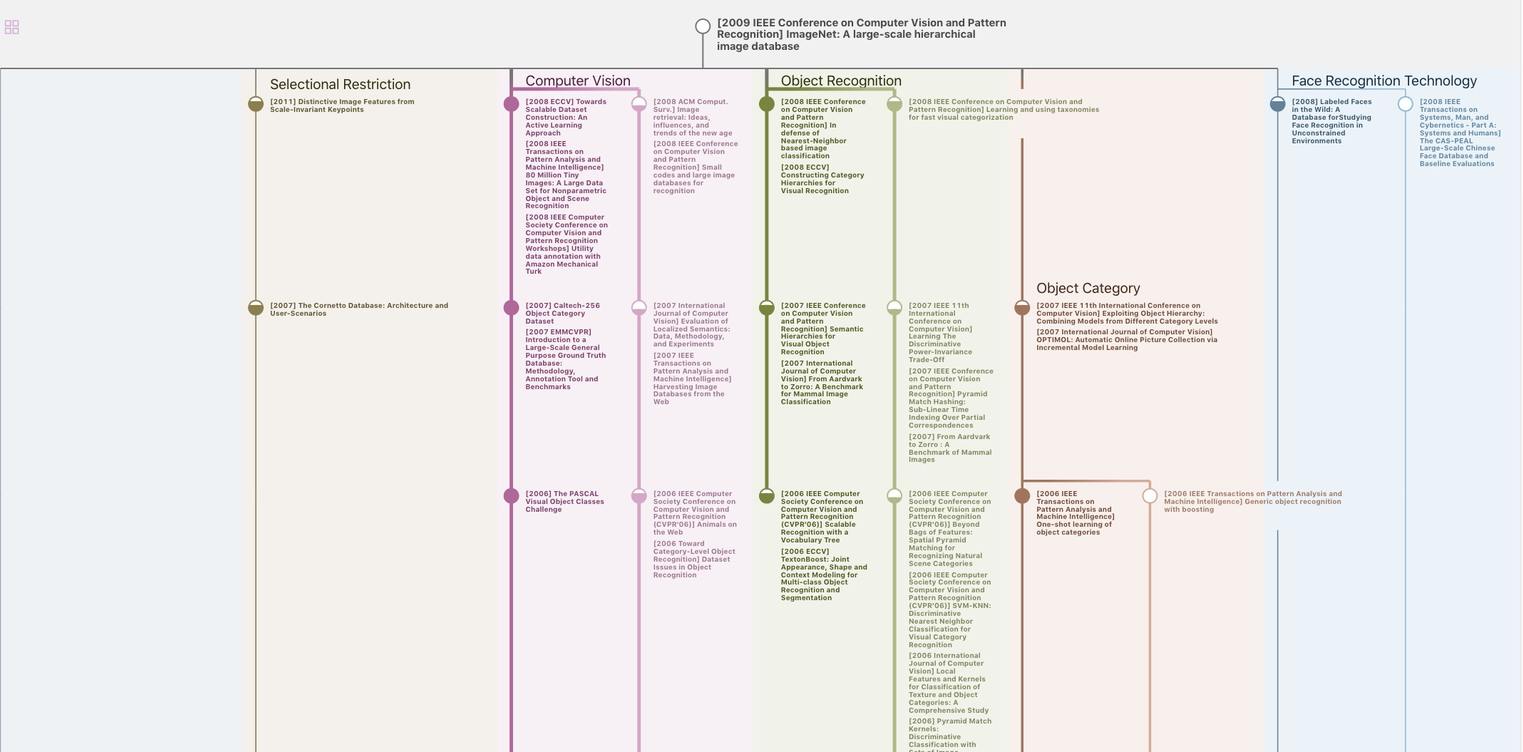
生成溯源树,研究论文发展脉络
Chat Paper
正在生成论文摘要