TAI-GAN: A Temporally and Anatomically Informed Generative Adversarial Network for early-to-late frame conversion in dynamic cardiac PET inter-frame motion correction
Medical Image Analysis(2024)
摘要
Inter-frame motion in dynamic cardiac positron emission tomography (PET) using rubidium-82 (82Rb) myocardial perfusion imaging impacts myocardial blood flow (MBF) quantification and the diagnosis accuracy of coronary artery diseases. However, the high cross-frame distribution variation due to rapid tracer kinetics poses a considerable challenge for inter-frame motion correction, especially for early frames where intensity-based image registration techniques often fail. To address this issue, we propose a novel method called Temporally and Anatomically Informed Generative Adversarial Network (TAI-GAN) that utilizes an all-to-one mapping to convert early frames into those with tracer distribution similar to the last reference frame. The TAI-GAN consists of a feature-wise linear modulation layer that encodes channel-wise parameters generated from temporal information and rough cardiac segmentation masks with local shifts that serve as anatomical information. Our proposed method was evaluated on a clinical 82Rb PET dataset, and the results show that our TAI-GAN can produce converted early frames with high image quality, comparable to the real reference frames. After TAI-GAN conversion, the motion estimation accuracy and subsequent myocardial blood flow (MBF) quantification with both conventional and deep learning-based motion correction methods were improved compared to using the original frames. The code is available at https://github.com/gxq1998/TAI-GAN.
更多查看译文
关键词
Early-to-late frame conversion,Dynamic cardiac PET,Inter-frame motion correction
AI 理解论文
溯源树
样例
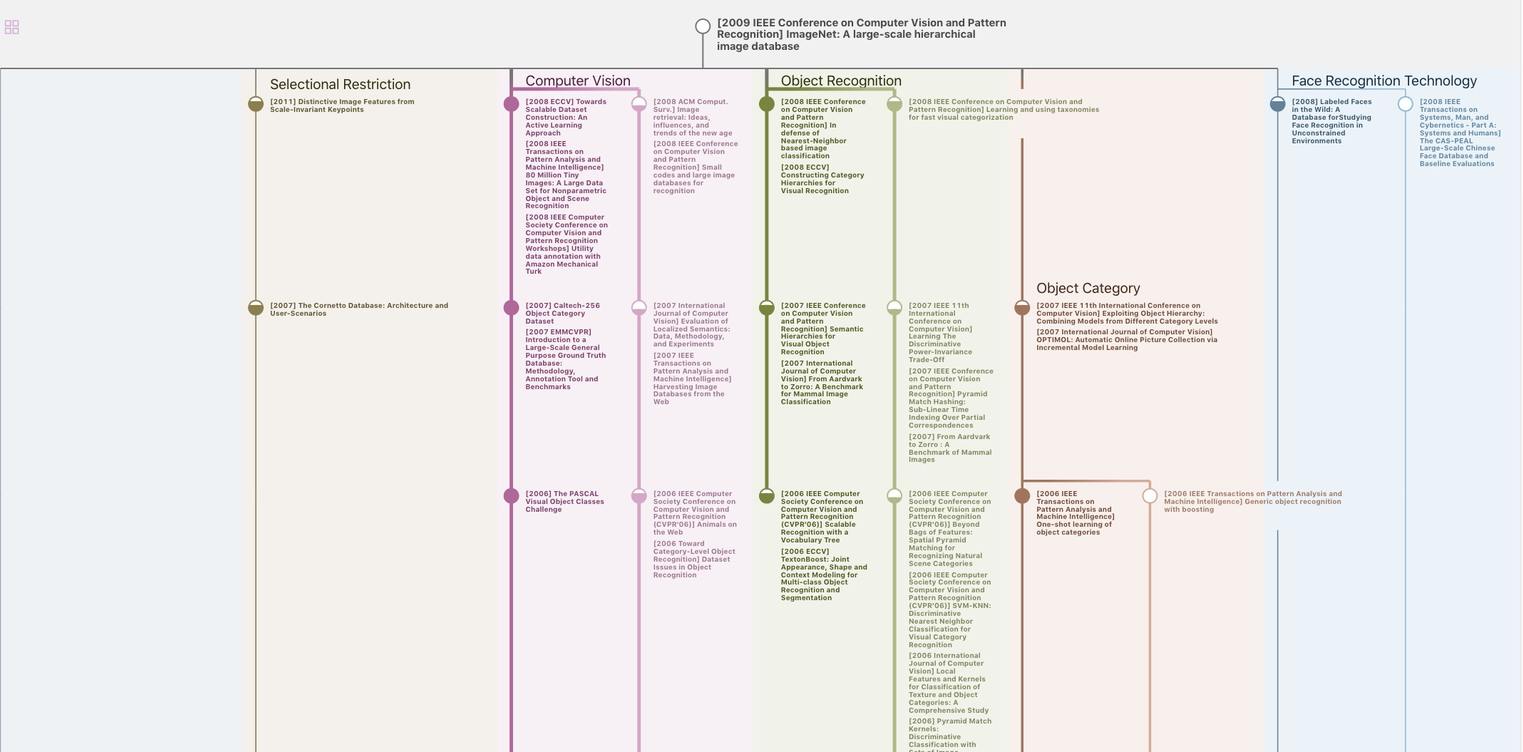
生成溯源树,研究论文发展脉络
Chat Paper
正在生成论文摘要