Mitigating subjectivity and bias in AI development indices: A robust approach to redefining country rankings
CoRR(2024)
摘要
Countries worldwide have been implementing different actions national
strategies for Artificial Intelligence (AI) to shape policy priorities and
guide their development concerning AI. Several AI indices have emerged to
assess countries' progress in AI development, aiding decision-making on
investments and policy choices. Typically, these indices combine multiple
indicators using linear additive methods such as weighted sums, although they
are limited in their ability to account for interactions among indicators.
Another limitation concerns the use of deterministic weights, which can be
perceived as subjective and vulnerable to debate and scrutiny, especially by
nations that feel disadvantaged. Aiming at mitigating these problems, we
conduct a methodological analysis to derive AI indices based on multiple
criteria decision analysis. Initially, we assess correlations between different
AI dimensions and employ the Choquet integral to model them. Thus, we apply the
Stochastic Multicriteria Acceptability Analysis (SMAA) to conduct a sensitivity
analysis using both weighted sum and Choquet integral in order to evaluate the
stability of the indices with regard the weights. Finally, we introduce a novel
ranking methodology based on SMAA, which considers several sets of weights to
derive the ranking of countries. As a result, instead of using predefined
weights, in the proposed approach, the ranking is achieved based on the
probabilities of countries in occupying a specific position. In the
computational analysis, we utilize the data employed in The Global AI Index
proposed by Tortoise. Results reveal correlations in the data, and our approach
effectively mitigates bias. In the sensitivity analysis, we scrutinize changes
in the ranking resulting from weight adjustments. We demonstrate that our
proposal rankings closely align with those derived from weight variations,
proving to be more robust.
更多查看译文
AI 理解论文
溯源树
样例
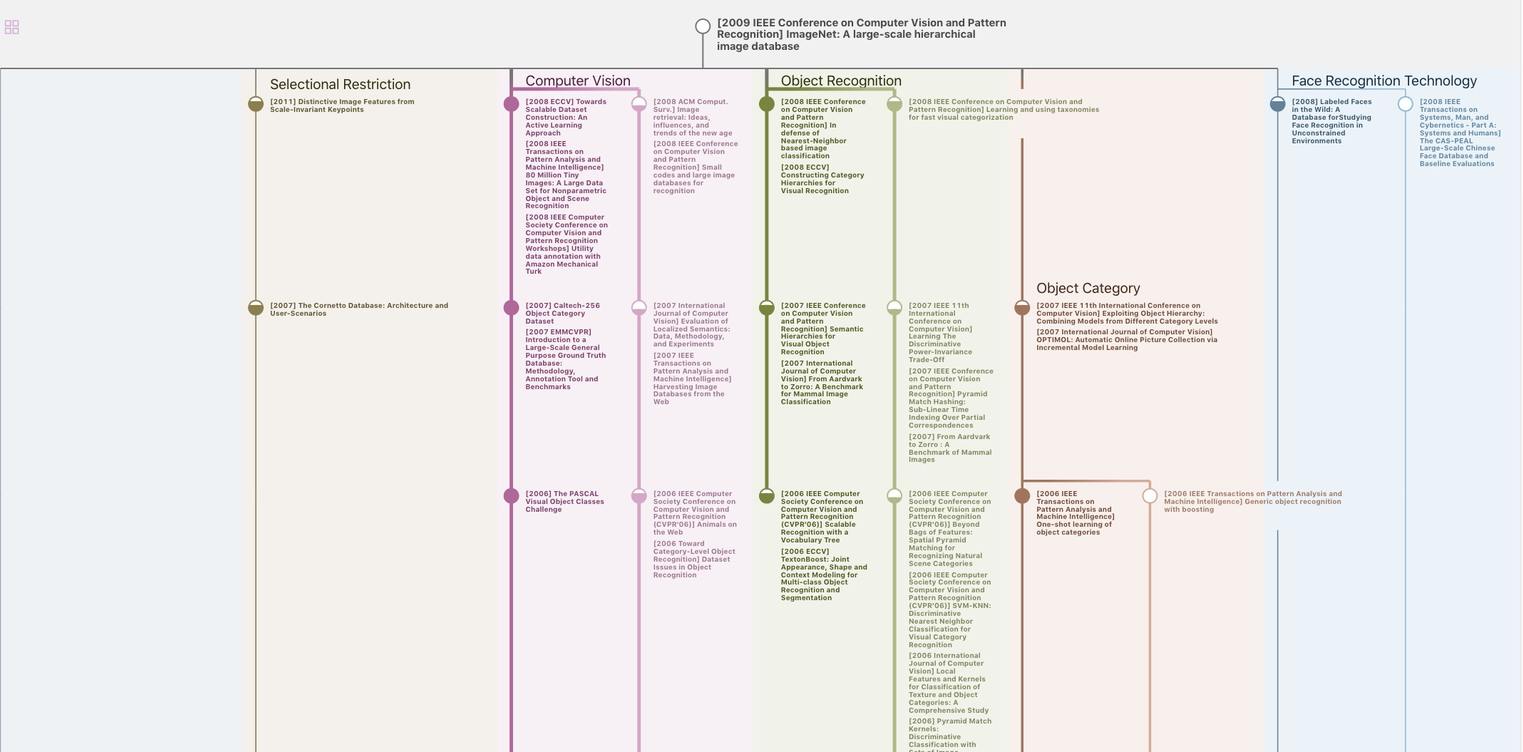
生成溯源树,研究论文发展脉络
Chat Paper
正在生成论文摘要