Comprehensive modelling of prostate cancer progression: integrating continuous and binary biomarkers with event time data and missing covariates
Discover Applied Sciences(2024)
摘要
Longitudinal studies involve recording observations at scheduled visits or time points for individuals until a predetermined event, like reaching satisfactory tumor shrinkage in cancer studies. Furthermore, dropout in longitudinal studies leads to incomplete data, which significantly increases the risk of bias. An amended joint shared-random effects model (SREM) is proposed for mixed continuous and binary longitudinal measurements and a time-to-event (TTE) outcome, incorporating missing covariates. In the proposed model, a conditional model is applied for the mixed continuous and binary longitudinal outcomes; a mixed effect model is considered for the continuous longitudinal outcome. For the binary longitudinal outcome, given the continuous longitudinal outcome, a logistic mixed effect model is considered. These models share common random effects with the model for the event time outcome. The model formulation is based on Bayesian statistical thinking via Markov Chain Monte Carlo (MCMC). The proposed joint modelling is applied to contribute to the understanding of the progression of prostate cancer (PCa) by considering a generalized linear mixed effects model for time-varying covariates that incorporate ignorable missingness. The association between prostate-specific antigen (PSA) with alkaline phosphatase (ALP) and tumor status has been studied with mixed conclusions.
更多查看译文
关键词
Conditional models,Generalized linear mixed model,Joint modelling,Missing-at-random,Shared random effects model
AI 理解论文
溯源树
样例
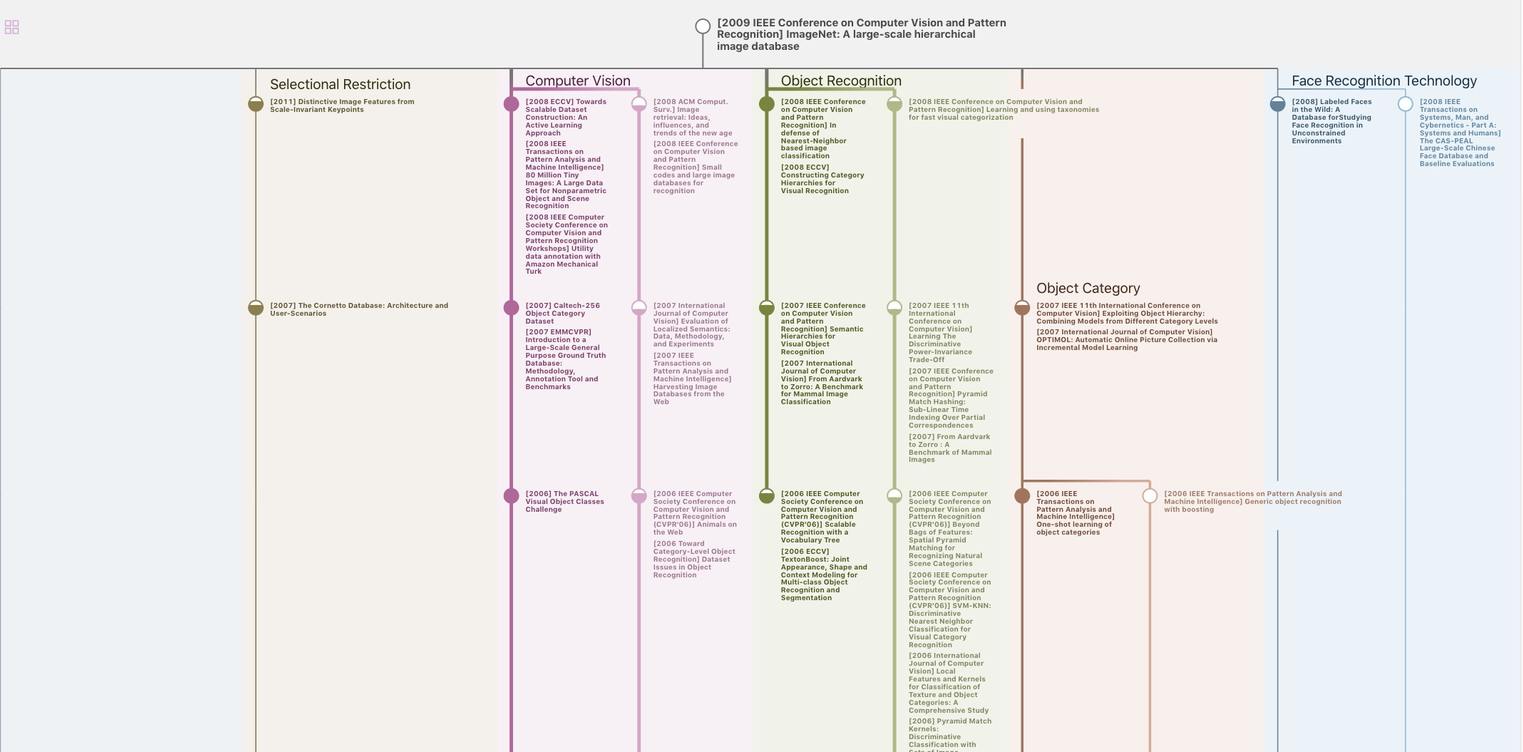
生成溯源树,研究论文发展脉络
Chat Paper
正在生成论文摘要