MLM-Net: Streamlined Multi-Lane Detection Network with Spatio-temporal Memory for Video Instance Lane Detection.
2023 IEEE 26th International Conference on Intelligent Transportation Systems (ITSC)(2023)
摘要
Lane detection is a crucial task in autonomous driving as it helps vehicles maintain stable and efficient driving in complex traffic environments. Image-based and video-based lane detection are two common methods, with video-based lane detection being more effective in handling complex scenarios such as lane occlusion and wear. However, most existing video-based lane detection methods suffer from information redundancy, where the same key frames of the same lane are repeatedly calculated during the detection of multiple lanes. Additionally, the spatio-temporal sequence information of video-based lanes is not fully utilized. To address these challenges, MLM-Net is proposed as a streamlined multilane network that facilitates the sharing of spatiotemporal information. The Global Memory Feature (GMF) module is redesigned to mitigate information redundancy across multiple lanes, while the Local Spatio-Temporal Feature (LSTF) module is introduced to enhance the utilization of spatiotemporal consistency information. Additionally, the Enhanced Global Memory Feature (EGMF) module is introduced to extract enhanced features and handle challenging detection scenarios. Experimental results demonstrate that our method achieves state-of-the-art performance for video instance lane detection on the VIL-100 dataset.
更多查看译文
关键词
Lane Detection,Spatiotemporal Memory,Global Features,Redundant Information,Spatiotemporal Characteristics,Memory Characteristics,Spatiotemporal Information,Feature Module,Multiple Lanes,Loss Function,Deep Learning,Decoding,Feature Maps,Recurrent Network,Improvement In Accuracy,Learning Network,Similar Mean,Temporal Information,Mean Accuracy,Similarity Matrix,Memory Reading,Contour Region,Global Feature Extraction,Affinity Matrix,Current Frame,Backbone Network,Video Frames,Key-value Pairs
AI 理解论文
溯源树
样例
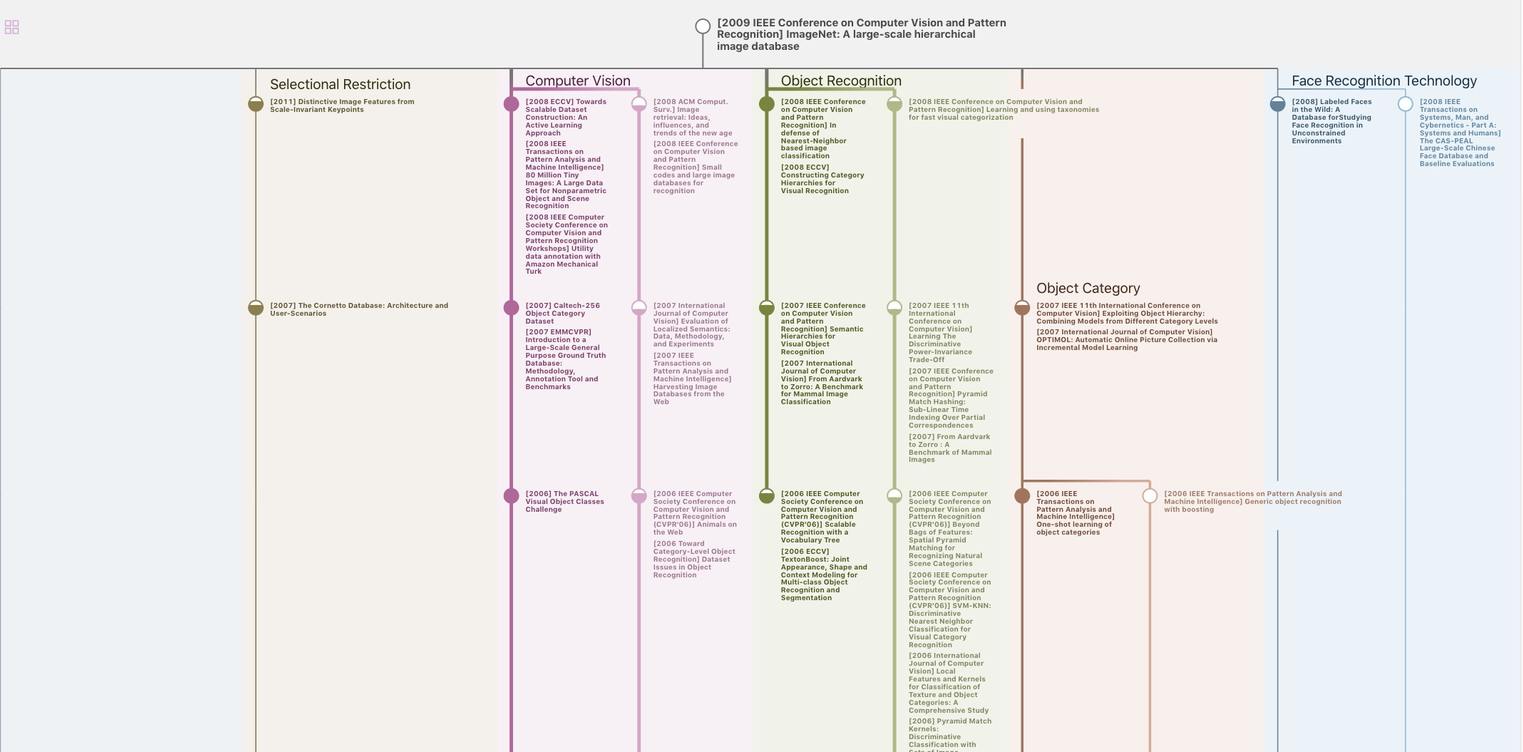
生成溯源树,研究论文发展脉络
Chat Paper
正在生成论文摘要