Dimension reduction and outlier detection of 3-D shapes derived from multi-organ CT images
BMC Medical Informatics and Decision Making(2024)
摘要
Background Unsupervised clustering and outlier detection are important in medical research to understand the distributional composition of a collective of patients. A number of clustering methods exist, also for high-dimensional data after dimension reduction. Clustering and outlier detection may, however, become less robust or contradictory if multiple high-dimensional data sets per patient exist. Such a scenario is given when the focus is on 3-D data of multiple organs per patient, and a high-dimensional feature matrix per organ is extracted. Methods We use principal component analysis (PCA), t-distributed stochastic neighbor embedding ( t -SNE) and multiple co-inertia analysis (MCIA) combined with bagplots to study the distribution of multi-organ 3-D data taken by computed tomography scans. After point-set registration of multiple organs from two public data sets, multiple hundred shape features are extracted per organ. While PCA and t -SNE can only be applied to each organ individually, MCIA can project the data of all organs into the same low-dimensional space. Results MCIA is the only approach, here, with which data of all organs can be projected into the same low-dimensional space. We studied how frequently (i.e., by how many organs) a patient was classified to belong to the inner or outer 50% of the population, or as an outlier. Outliers could only be detected with MCIA and PCA. MCIA and t -SNE were more robust in judging the distributional location of a patient in contrast to PCA. Conclusions MCIA is more appropriate and robust in judging the distributional location of a patient in the case of multiple high-dimensional data sets per patient. It is still recommendable to apply PCA or t -SNE in parallel to MCIA to study the location of individual organs.
更多查看译文
关键词
CT scans,Outlier detection,Dimension reduction,Multiple co-inertia analysis,Bagplots
AI 理解论文
溯源树
样例
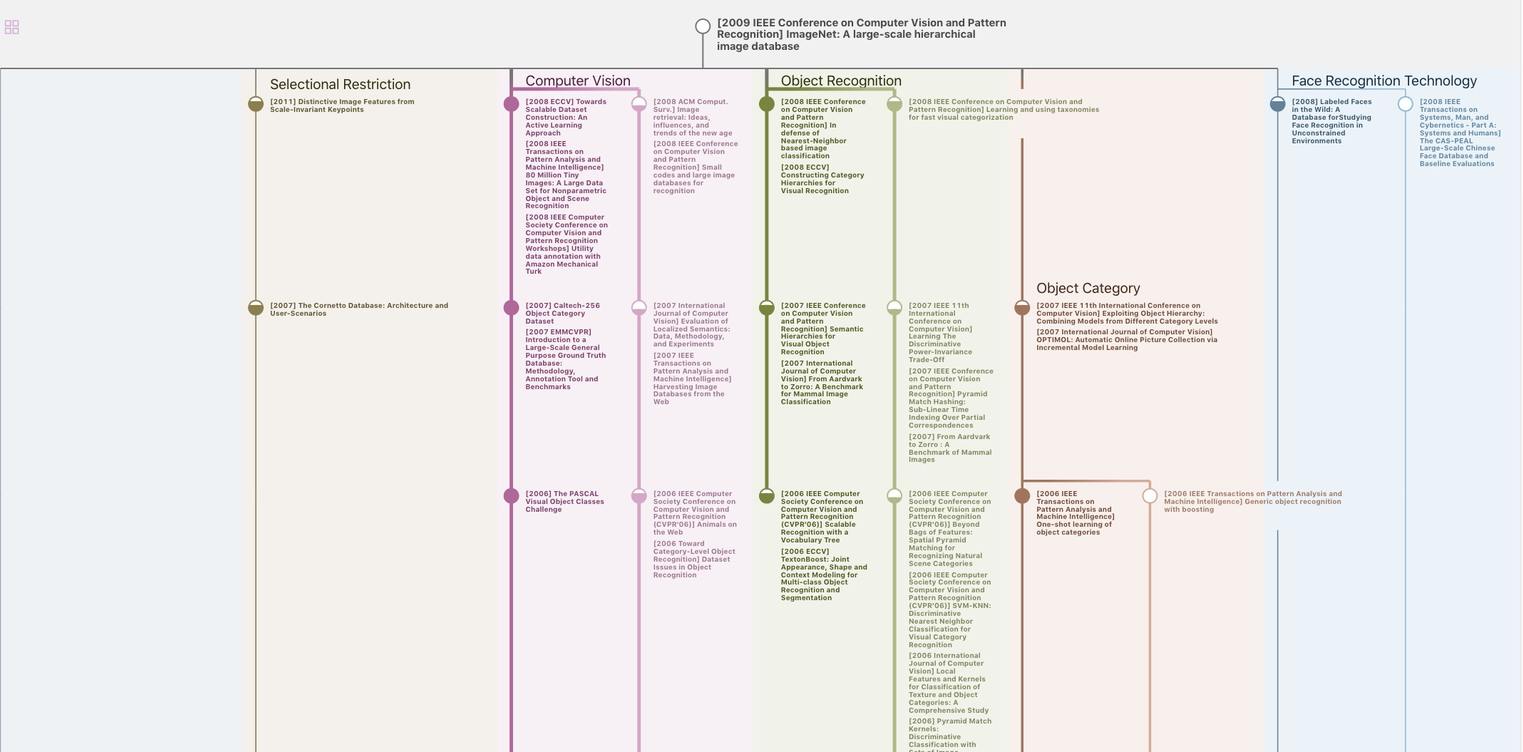
生成溯源树,研究论文发展脉络
Chat Paper
正在生成论文摘要