Neural MPC-Based Decision-Making Framework for Autonomous Driving in Multi-Lane Roundabout.
2023 IEEE 26th International Conference on Intelligent Transportation Systems (ITSC)(2023)
摘要
The multi-lane roundabout poses significant challenges for autonomous driving due to its complex road structure and traffic conditions. To address these challenges, this paper proposes a novel Neural Model Predictive Control (NMPC)-based decision-making framework that integrates prediction, planning, and control for autonomous vehicles to navigate multi-lane roundabouts. The proposed NMPC framework learns a dynamical model, incorporating interaction data, to accurately predict the behavior of surrounding traffic participants. Multiple candidate static paths are then generated based on the road structure, and the decision-making problem is formulated as a series of parallel static path tracking control problems subject to safety constraints. The static path with minimal tracking cost is selected as the target reference path, and the tracking control is generated simultaneously. To enhance computational efficiency, NMPC utilizes a critic network and an actor network to approximate the tracking cost and the control policy, respectively. Experimental evaluation on a multi-lane roundabout simulator, based on a real roundabout in Beijing, demonstrates that the proposed method performs better in terms of driving safety and efficiency compared to several baseline algorithms across various traffic densities.
更多查看译文
关键词
Autonomous Vehicles,Roundabout,Decision-making Framework,Neural Framework,Prediction Accuracy,Dynamical,Computational Efficiency,Control Problem,Minimum Cost,Traffic Congestion,Actor Network,Model Predictive Control,Tracking Control,Traffic Conditions,Neural Control,Decision-making Problems,Static Problem,Critic Network,Parallel Paths,Parallel Tracks,Steering Angle,Lane Change,Rule-based Methods,Target Path,Reference Trajectory,Dynamic Tracking,Optimal Control Problem,Candidate Paths,Traffic Flow,Adjacent Lane
AI 理解论文
溯源树
样例
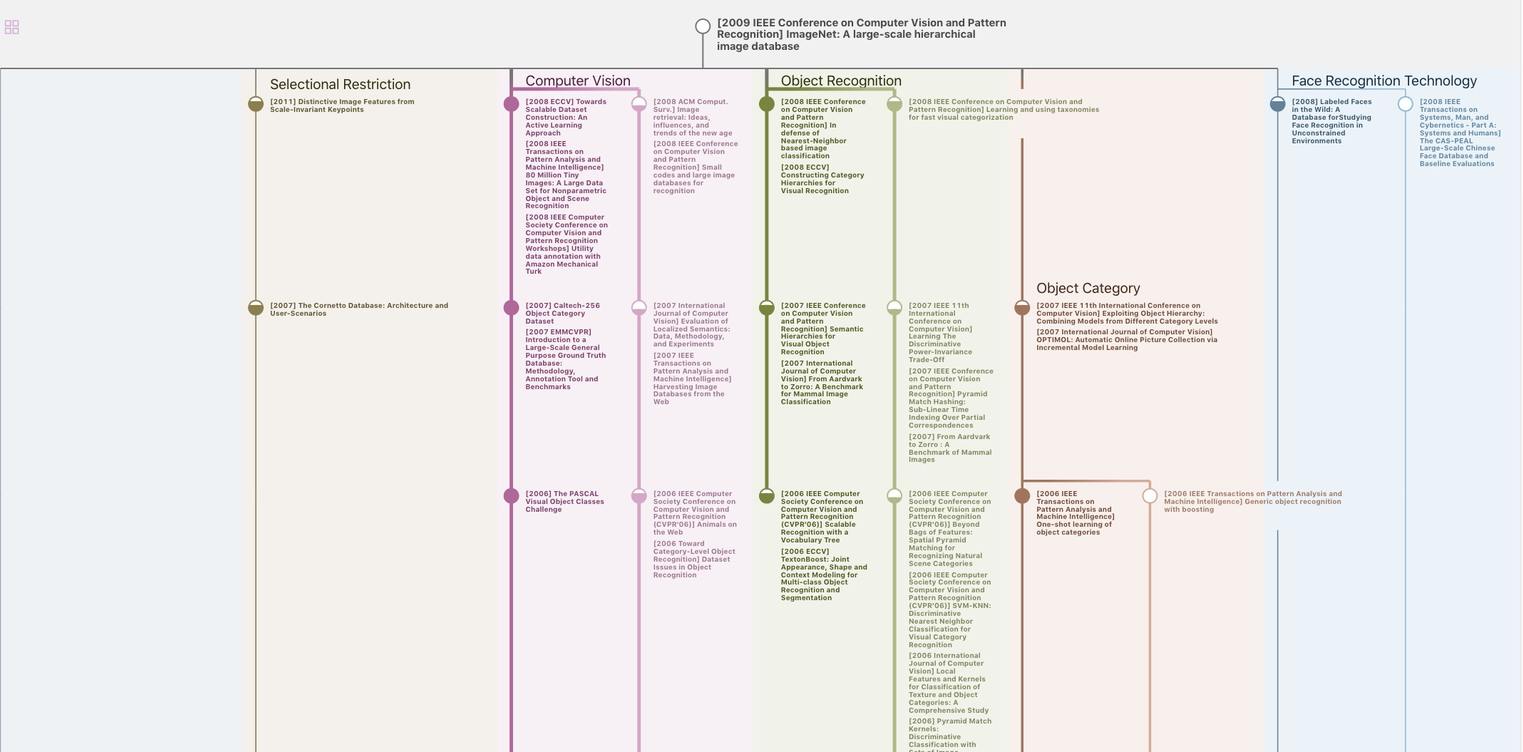
生成溯源树,研究论文发展脉络
Chat Paper
正在生成论文摘要