Multi-Modal Sensor Framework with Learnable Uncertainty Estimator for Pedestrian Trajectory Prediction.
2023 IEEE 26th International Conference on Intelligent Transportation Systems (ITSC)(2023)
摘要
This paper presents a novel pedestrian trajectory prediction framework for autonomous driving systems in multimodality sensor settings where the set of sensors (i.e., camera, LiDAR, etc.) can be used for prediction task. While achiveving promising results, the existing camera-based prediction models often fail to capture more complex human motion in realworld. Our work mitigate this issue by proposing a multimodal sensor framework for pedestrian trajectory prediction (xMTP), consisting of two branches of predictors. On the first branch, we leverage the promising performances of the existing camera-based prediction models, such as BiTrap [1] and PIE [2], to model the human intentions. We refer to the existing camera-based prediction models as native predictors, that can potentially be any off-the-shelf prediction model. On the other branch, we propose a novel LiDARbased predictor (P3D) model the pedestrians'
$\mathbf{3D}$
movements as LiDAR data provides rich depth information that help reason about pedestrian motions in real world. We then develop a novel learnable prediction sharing with uncertainty estimation (PSUE) to quantify the uncertainties of predictions from the two branches relative to their past performances on observed pedestrian motions to select the best prediction for the given scenarios. Through extensive experiments on commonly-used datasets: KITTI [3] and SHIFT [4], consisting of both camera and LiDAR data, we demonstrate the effectiveness of our framework in incorporating two different state-of-the-art native predictors, BiTrap [1] and PIE [2], under different pedestrian movement scenarios.
更多查看译文
关键词
Uncertainty Estimation,Trajectory Prediction,Multimodal Sensor,Pedestrian Trajectory Prediction,Walking,Prediction Model,Prediction Task,Prediction Uncertainty,Lidar Data,Camera Data,Pedestrian Movement,Prediction Accuracy,Time Step,Prediction Error,Object Detection,Visual Features,Recurrent Neural Network,Bounding Box,Image Sensor,Multimodal Approach,KITTI Dataset,3D Movement,Autoencoder Framework,Prediction Module,3D Bounding Box,Multimodal Dataset,Predicted Bounding Box,Human Pose,Scene Context,Dynamic Scenes
AI 理解论文
溯源树
样例
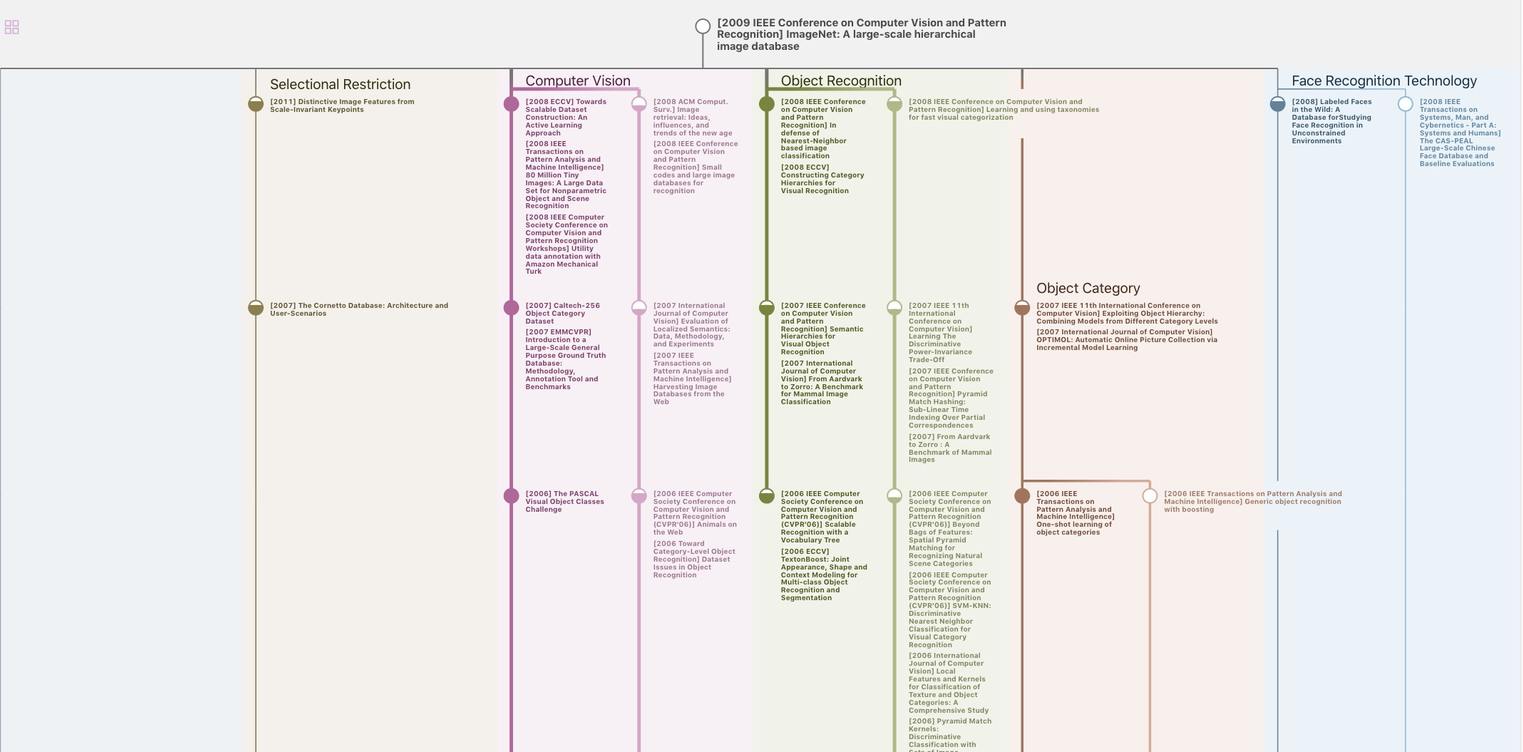
生成溯源树,研究论文发展脉络
Chat Paper
正在生成论文摘要