Unsupervised Traffic Sign Classification Relying on Explanatory Visible Factors.
2023 IEEE 26th International Conference on Intelligent Transportation Systems (ITSC)(2023)
摘要
Intelligent behavior of autonomous vehicles must rely on the understanding of explanatory factors of the environment in order to operate safely. To implement such intelligence, machine learning models such as CNNs are used to identify objects such as traffic signs. However, the process behind arriving at a certain result is hard to understand. Explainable artificial intelligence has the potential to overcome this limitation by unveiling explanatory factors learned by models and hence, increase the reliability of recognition systems. Recent progress in explainable artificial intelligence motivates research in various fields, and their application must become a core part of intelligent transport systems. We present in this paper an explainable and unsupervised methodology for traffic sign classification. The proposed pipeline combines methods for explainable feature extraction as well as out-of-distribution detection, which were previously applied in anomaly detection and evolutionary biology. This pipeline learns explanatory factors of a traffic sign class, and models a classification function without knowing other classes. Our method is evaluated using the GTSRB as well as the Tsinghua-Tencent-100k dataset and compared to a deep learning counterpart, namely GANs. The results show that the presented methodology is feasible to classify traffic sign images, is explainable and outperforms deep learning-based models.
更多查看译文
关键词
Explainable AI,Traffic Sign Classification,Perception
AI 理解论文
溯源树
样例
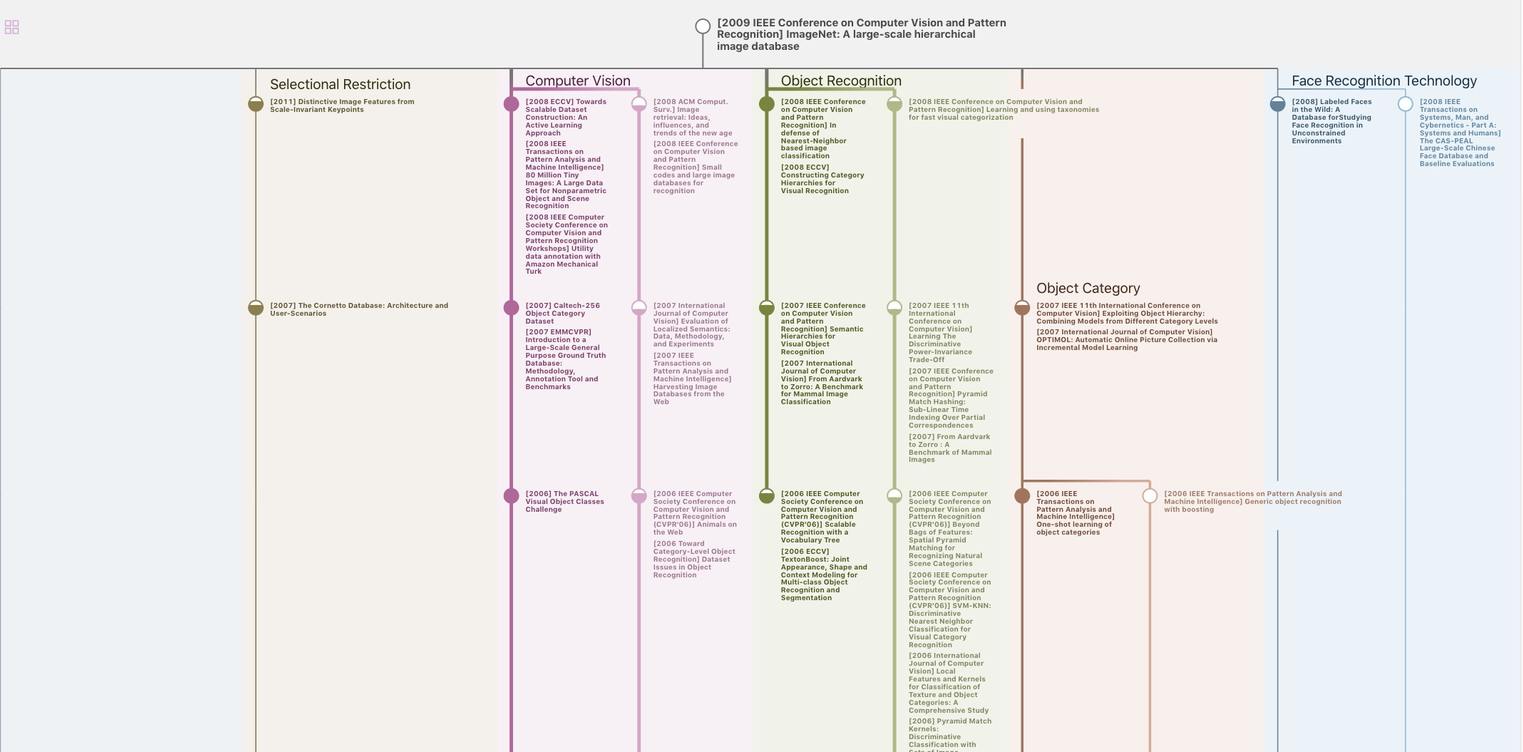
生成溯源树,研究论文发展脉络
Chat Paper
正在生成论文摘要