An Accelerated Gradient Method for Simple Bilevel Optimization with Convex Lower-level Problem
CoRR(2024)
摘要
In this paper, we focus on simple bilevel optimization problems, where we
minimize a convex smooth objective function over the optimal solution set of
another convex smooth constrained optimization problem. We present a novel
bilevel optimization method that locally approximates the solution set of the
lower-level problem using a cutting plane approach and employs an accelerated
gradient-based update to reduce the upper-level objective function over the
approximated solution set. We measure the performance of our method in terms of
suboptimality and infeasibility errors and provide non-asymptotic convergence
guarantees for both error criteria. Specifically, when the feasible set is
compact, we show that our method requires at most
𝒪(max{1/√(ϵ_f), 1/ϵ_g}) iterations to find a
solution that is ϵ_f-suboptimal and ϵ_g-infeasible. Moreover,
under the additional assumption that the lower-level objective satisfies the
r-th Hölderian error bound, we show that our method achieves an iteration
complexity of
𝒪(max{ϵ_f^-2r-1/2r,ϵ_g^-2r-1/2r}),
which matches the optimal complexity of single-level convex constrained
optimization when r=1.
更多查看译文
AI 理解论文
溯源树
样例
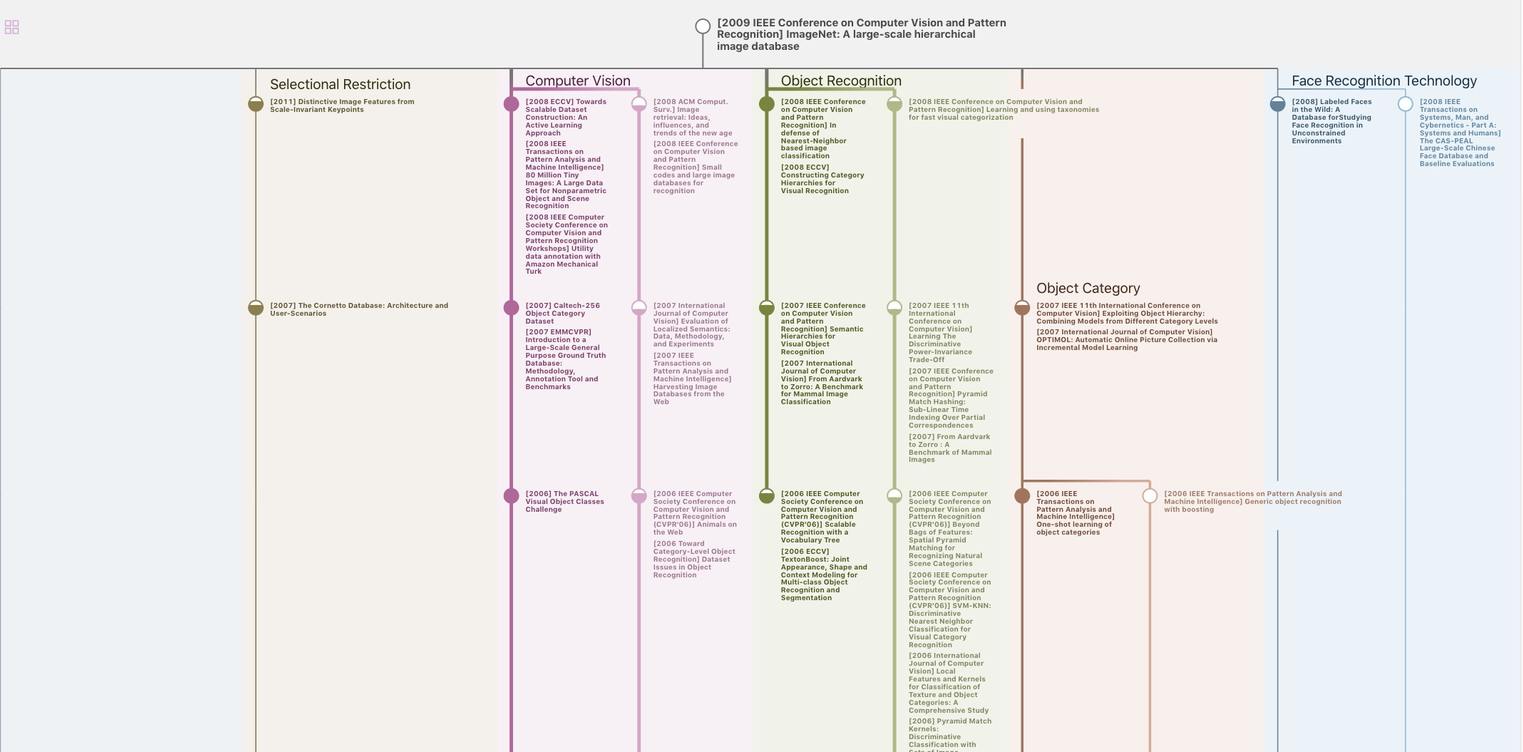
生成溯源树,研究论文发展脉络
Chat Paper
正在生成论文摘要