Regional Adaptive Metropolis Light Transport
CoRR(2024)
摘要
The design of the proposal distributions, and most notably the kernel
parameters, are crucial for the performance of Markov chain Monte Carlo (MCMC)
rendering. A poor selection of parameters can increase the correlation of the
Markov chain and result in bad rendering performance. We approach this problem
by a novel path perturbation strategy for online-learning of state-dependent
kernel parameters. We base our approach on the theoretical framework of
regional adaptive MCMC which enables the adaptation of parameters depending on
the region of the state space which contains the current sample, and on
information collected from previous samples. For this, we define a partitioning
of the path space on a low-dimensional canonical space to capture the
characteristics of paths, with a focus on path segments closer to the sensor.
Fast convergence is achieved by adaptive refinement of the partitions.
Exemplarily, we present two novel regional adaptive path perturbation
techniques akin to lens and multi-chain perturbations. Our approach can easily
be used on top of existing path space MLT methods to improve rendering
efficiency, while being agnostic to the initial choice of kernel parameters.
更多查看译文
AI 理解论文
溯源树
样例
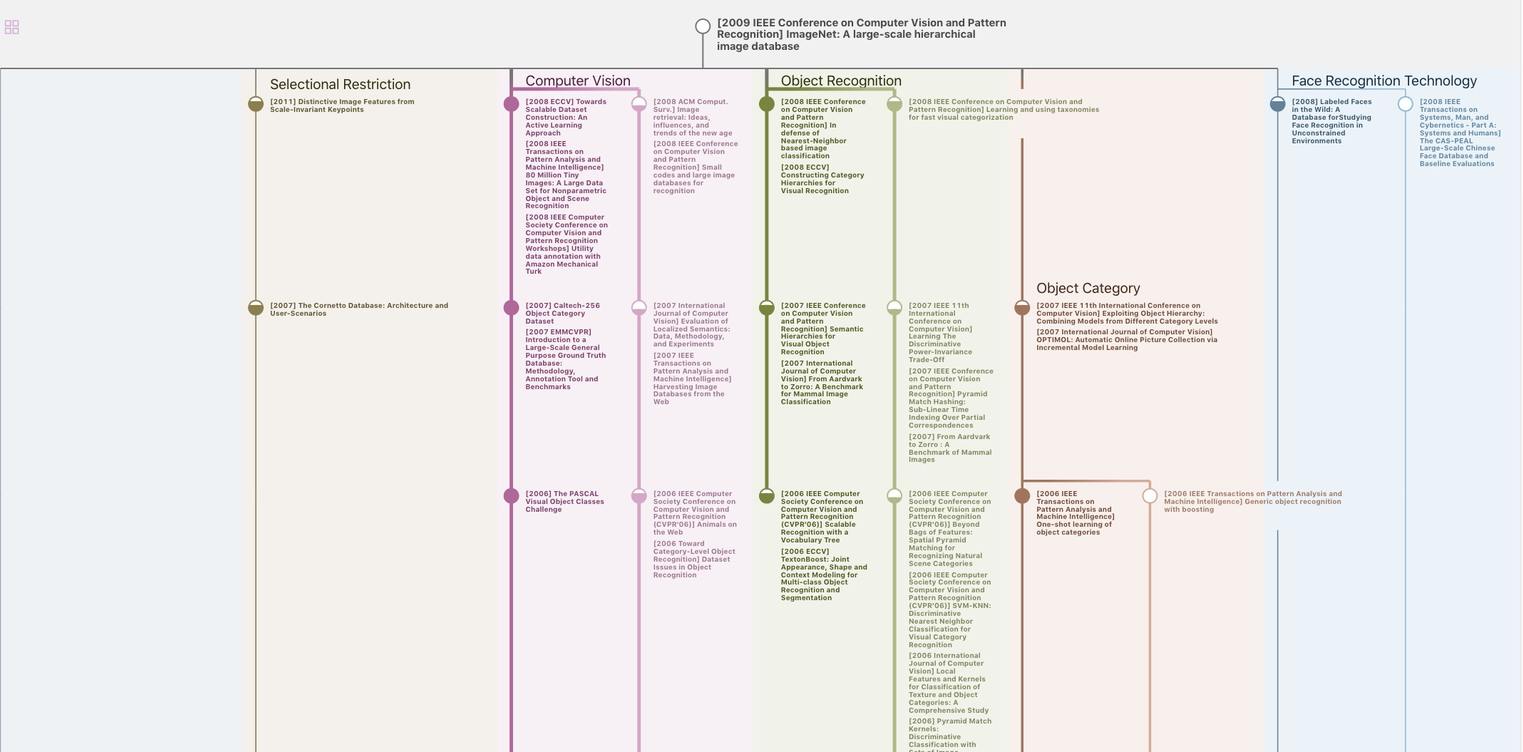
生成溯源树,研究论文发展脉络
Chat Paper
正在生成论文摘要