Grownbb: Gromov–Wasserstein learning of neural best buddies for cross-domain correspondence
The Visual Computer(2024)
摘要
Identifying pixel correspondences between two images is a fundamental task in computer vision, and has been widely used for 3D reconstruction, image morphing, and image retrieval. The neural best buddies (NBB) finds sparse correspondences between cross-domain images, which have semantically related local structures, though could be quite different in semantics as well as appearances. This paper presents a new method for cross-domain image correspondence, called GroWNBB, by incorporating the Gromov–Wasserstein learning into the NBB framework. Specifically, we utilize the NBB as the backbone to search feature matching from deep layer and propagate to low layer. While for each layer, we modify the strategy of NBB by further mapping the matching pairs obtained from the NBB within and across images into graphs, then formulate the matches as optimal transport between graphs, and use Gromov–Wasserstein learning to establish matches between these graphs. Consequently, our approach considers the relationships between images as well as the relationships within images, which makes the correspondence more stable. Our experiments demonstrate that GroWNBB achieves state-of-the-art performance on cross-domain correspondence and outperforms other popular methods in intra-class and same object correspondence estimation. Our code is available at https://github.com/NolanInLowland/GroWNBB .
更多查看译文
关键词
Cross-domain correspondence,Optimal transport,Neural best buddies,Gromov–Wasserstein distance
AI 理解论文
溯源树
样例
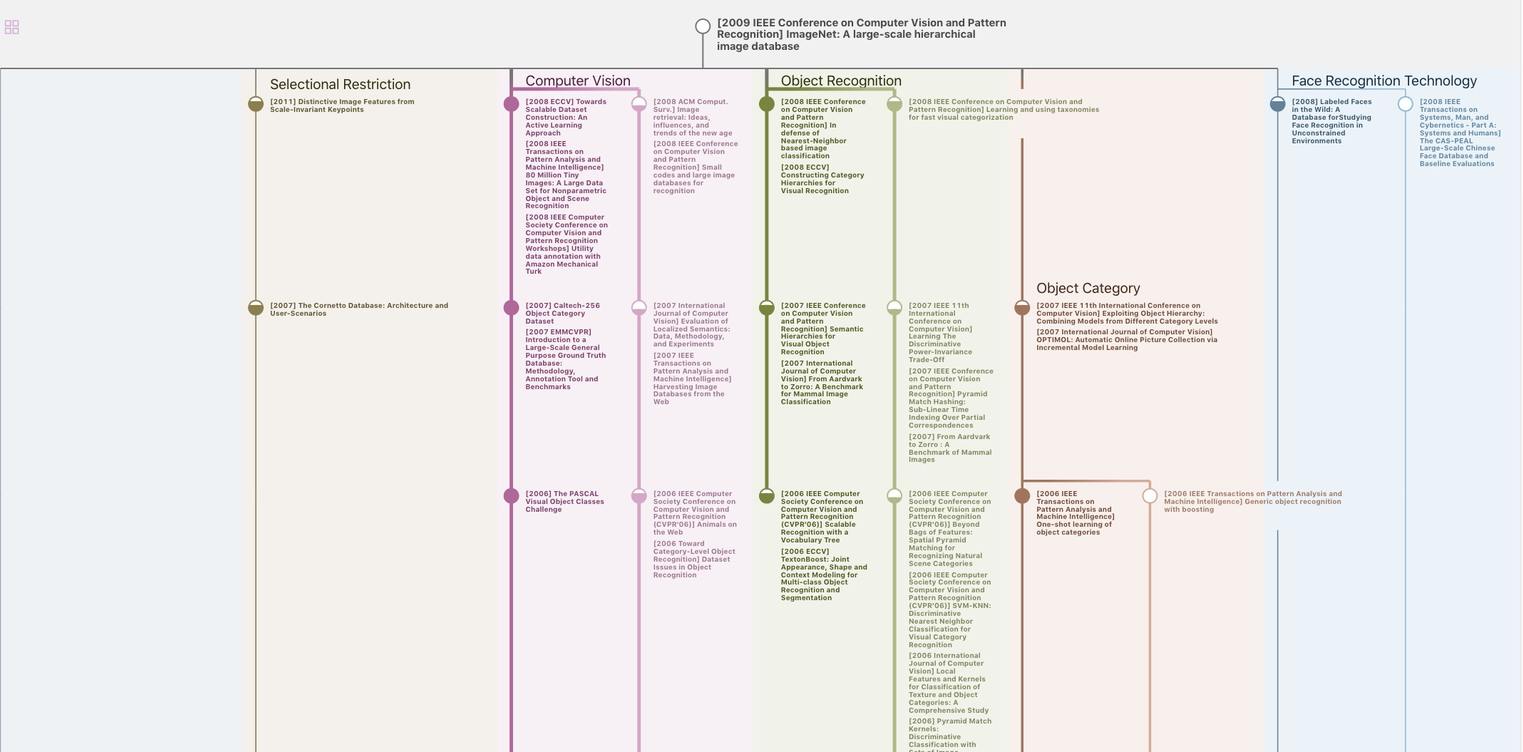
生成溯源树,研究论文发展脉络
Chat Paper
正在生成论文摘要