Rethinking Graph Masked Autoencoders through Alignment and Uniformity
AAAI 2024(2024)
Abstract
Self-supervised learning on graphs can be bifurcated into contrastive and generative methods. Contrastive methods, also known as graph contrastive learning (GCL), have dominated graph self-supervised learning in the past few years, but the recent advent of graph masked autoencoder (GraphMAE) rekindles the momentum behind generative methods. Despite the empirical success of GraphMAE, there is still a dearth of theoretical understanding regarding its efficacy. Moreover, while both generative and contrastive methods have been shown to be effective, their connections and differences have yet to be thoroughly investigated. Therefore, we theoretically build a bridge between GraphMAE and GCL, and prove that the node-level reconstruction objective in GraphMAE implicitly performs context-level GCL. Based on our theoretical analysis, we further identify the limitations of the GraphMAE from the perspectives of alignment and uniformity, which have been considered as two key properties of high-quality representations in GCL. We point out that GraphMAE's alignment performance is restricted by the masking strategy, and the uniformity is not strictly guaranteed. To remedy the aforementioned limitations, we propose an Alignment-Uniformity enhanced Graph Masked AutoEncoder, named AUG-MAE. Specifically, we propose an easy-to-hard adversarial masking strategy to provide hard-to-align samples, which improves the alignment performance. Meanwhile, we introduce an explicit uniformity regularizer to ensure the uniformity of the learned representations. Experimental results on benchmark datasets demonstrate the superiority of our model over existing state-of-the-art methods. The code is available at: https://github.com/AzureLeon1/AUG-MAE.
MoreTranslated text
Key words
ML: Graph-based Machine Learning,DMKM: Graph Mining, Social Network Analysis & Community,ML: Unsupervised & Self-Supervised Learning
AI Read Science
Must-Reading Tree
Example
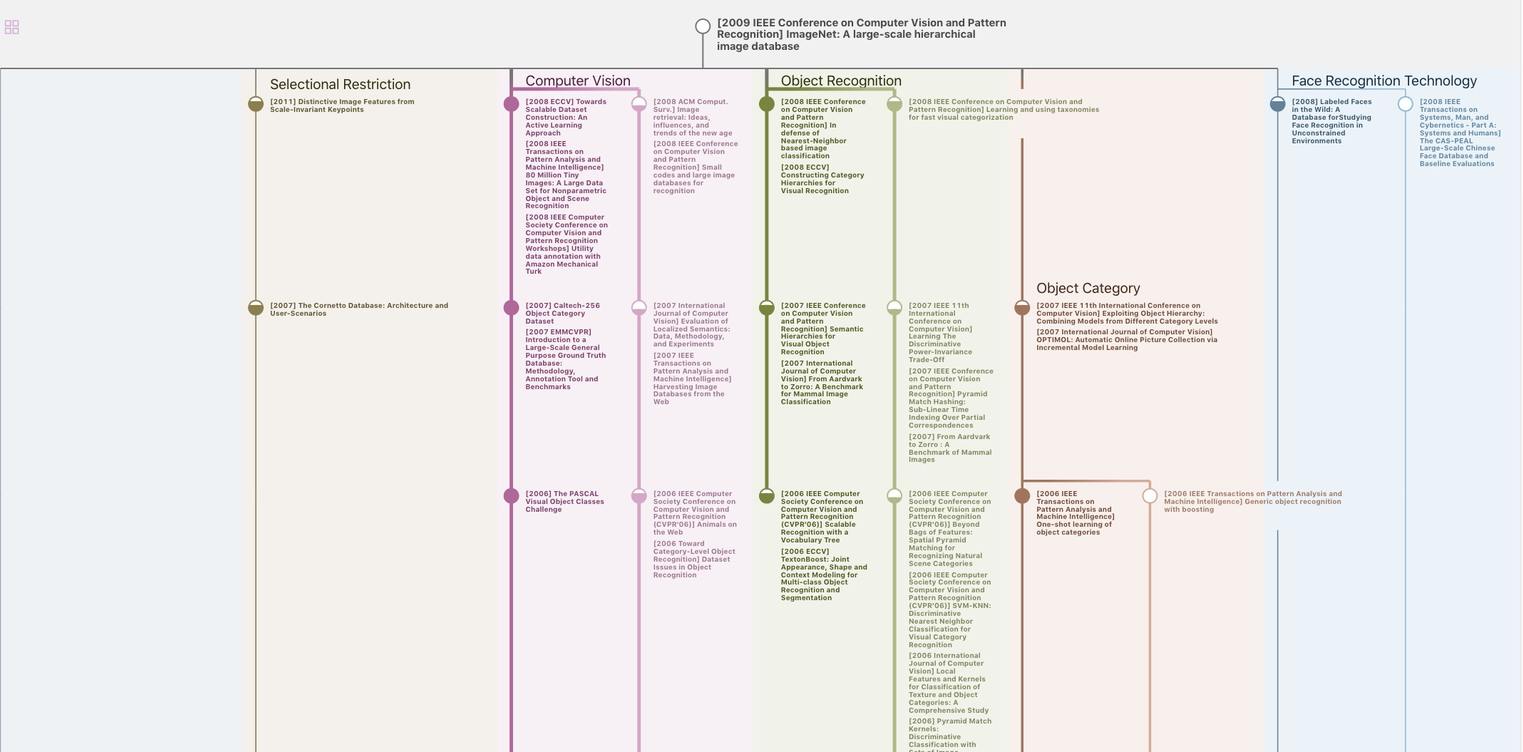
Generate MRT to find the research sequence of this paper
Chat Paper
Summary is being generated by the instructions you defined