Informativeness of Reward Functions in Reinforcement Learning
CoRR(2024)
摘要
Reward functions are central in specifying the task we want a reinforcement
learning agent to perform. Given a task and desired optimal behavior, we study
the problem of designing informative reward functions so that the designed
rewards speed up the agent's convergence. In particular, we consider
expert-driven reward design settings where an expert or teacher seeks to
provide informative and interpretable rewards to a learning agent. Existing
works have considered several different reward design formulations; however,
the key challenge is formulating a reward informativeness criterion that adapts
w.r.t. the agent's current policy and can be optimized under specified
structural constraints to obtain interpretable rewards. In this paper, we
propose a novel reward informativeness criterion, a quantitative measure that
captures how the agent's current policy will improve if it receives rewards
from a specific reward function. We theoretically showcase the utility of the
proposed informativeness criterion for adaptively designing rewards for an
agent. Experimental results on two navigation tasks demonstrate the
effectiveness of our adaptive reward informativeness criterion.
更多查看译文
AI 理解论文
溯源树
样例
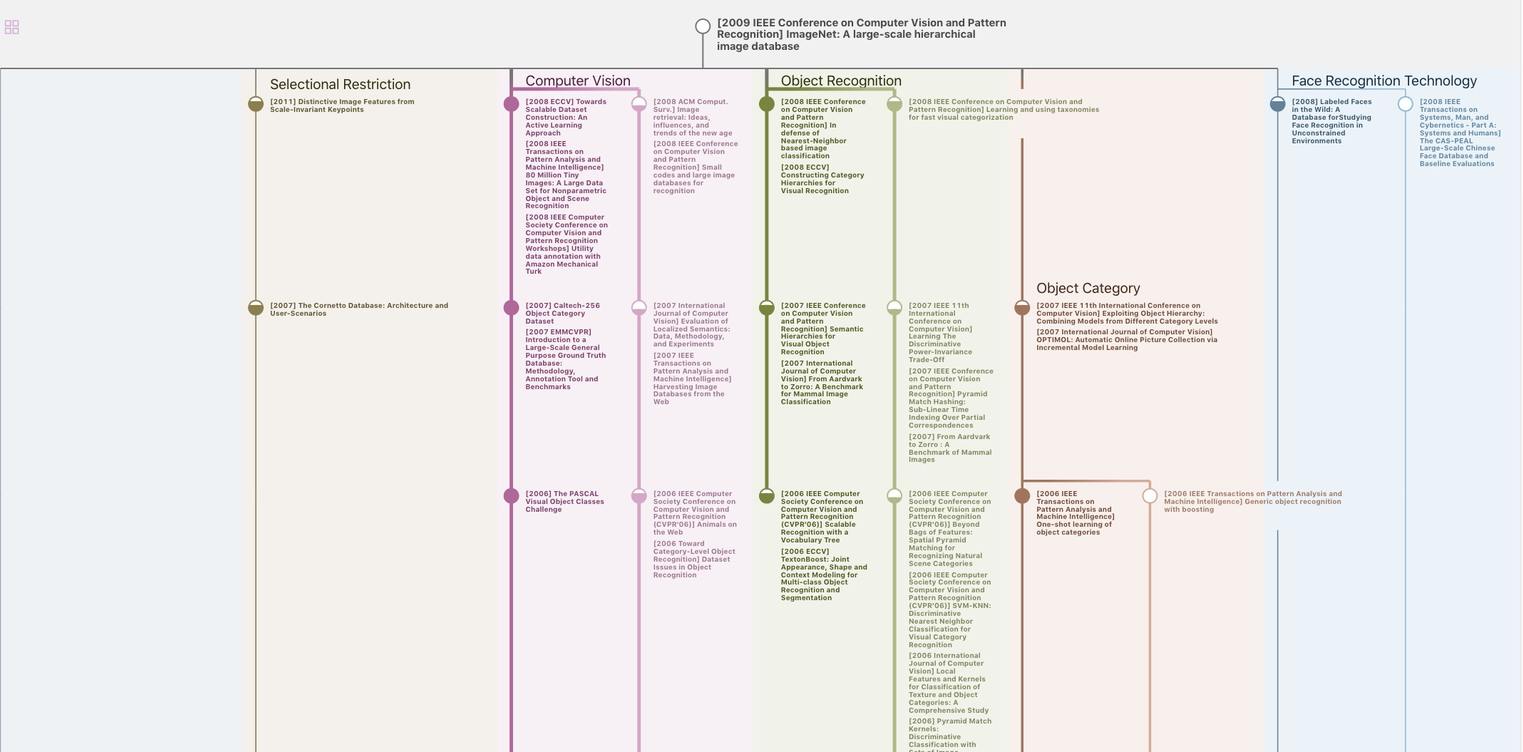
生成溯源树,研究论文发展脉络
Chat Paper
正在生成论文摘要