Advanced modeling for predicting compressive strength in fly ash-modified recycled aggregate concrete: XGboost, MEP, MARS, and ANN approaches
Innovative Infrastructure Solutions(2024)
摘要
In recent years, there has been a global trend toward producing environmentally friendly construction materials. concrete, one of the most widely used construction materials worldwide, has received substantial attention from researchers in the pursuit of eco-friendliness. This is because approximately three-quarters of concrete is composed of natural aggregates, which harm the local environment during their extraction. This study employed soft computing models to predict the compressive strength of fly ash-modified concrete produced with recycled aggregate concrete (RCA) to replace natural fine and/or coarse aggregate. For that purpose, a database containing 295 data points was assembled from the literature and utilized to develop models for predicting CS of various RCA mixture mix proportions. The database included diverse concrete mixtures with varying input parameters: cement content (225–560 kg/m 3 ), water-to-binder ratio (0.29–0.66), natural coarse aggregate (0–1237 kg/m 3 ), natural fine aggregate (0–1050 kg/m 3 ), recycled coarse aggregate (0–1215 kg/m 3 ), recycled fine aggregate (0–1050 kg/m 3 ), fly ash (0–225.5 kg/m 3 ), superplasticizer (0–7.3 kg/m 3 ), and curing times (1–90 days). In the modeling process, machine learning approaches were used, specifically the Multi Expression Programming, Artificial Neural Network, Multi Adaptive Regression Spline , and Extreme Gradient Boosting models. Cross-validation was used to determine tuning the required training parameters. The generated models were assessed using statistical evaluation tools, including the correlation coefficient (R), Root Mean Squared Error (RMSE), Mean Absolute Error, and Scatter Index (SI). Based on the evaluation of the developed models, the XGboost model outperformed other models using fivefold cross-validation, demonstrating high R and low RMSE and SI. SHAP values were then utilized to identify the most influential parameters in predicting the compressive strength of RA concrete, with curing time ranking as the most important, followed by water-to-binder ratio and cement content.
更多查看译文
关键词
Compressive strength,Fine and coarse aggregate replacement,Recycled concrete aggregate,Modeling,SHAP sensitivity analysis
AI 理解论文
溯源树
样例
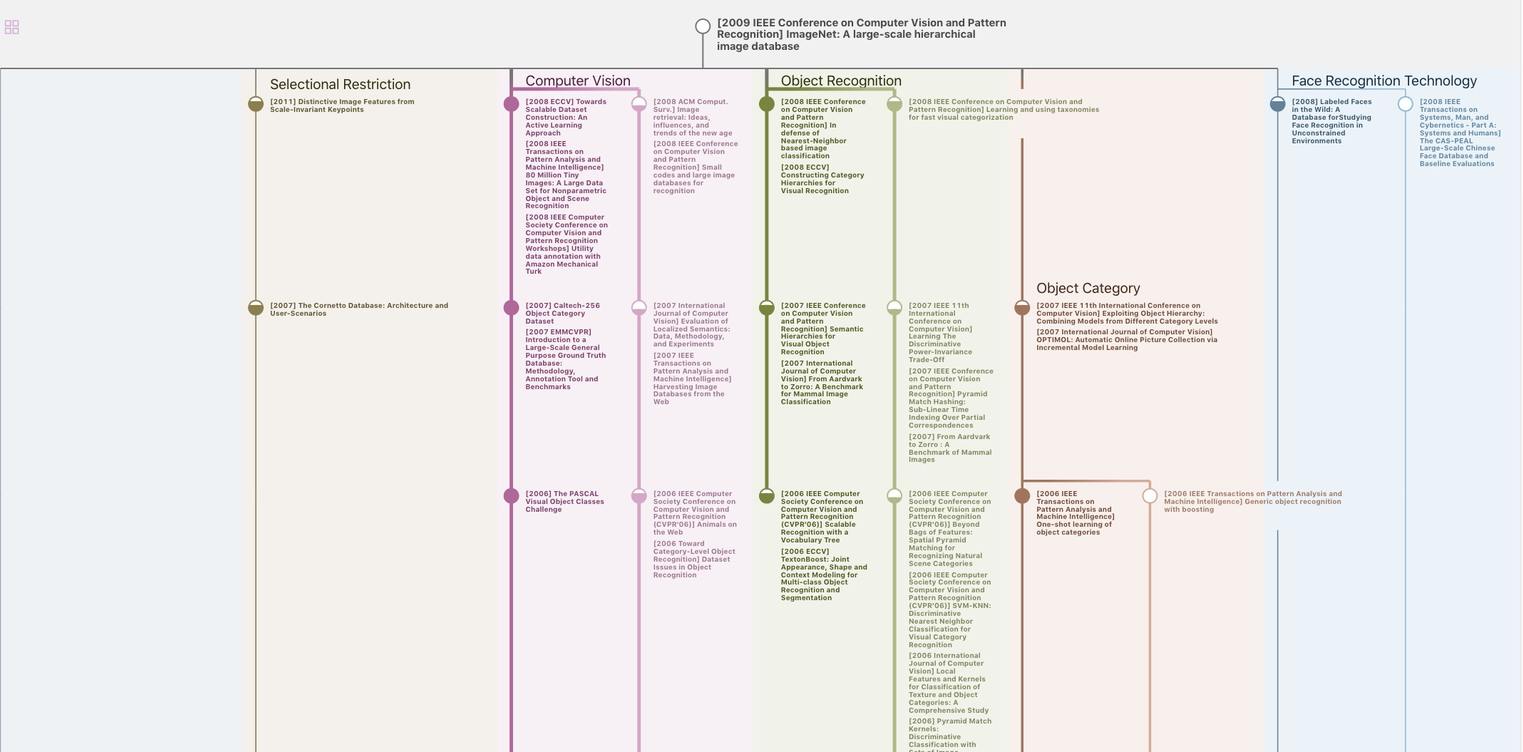
生成溯源树,研究论文发展脉络
Chat Paper
正在生成论文摘要