MaCo: efficient unsupervised low-light image enhancement via illumination-based magnitude control
The Visual Computer(2024)
摘要
This paper presents a novel low-light image enhancement (LLIE) method based on magnitude control (MaCo). Our method establishes a relationship between the low-light image and illumination, using pixel intensity and image brightness. Exploiting this relationship, MaCo enhances pixels with varying magnitudes, achieving pixel-wise LLIE. This yields high-quality enhanced images without local overexposure. We also introduce a set of carefully formulated unsupervised loss functions to enable training using only low-light images. Concretely, our method first trains a lightweight deep network, Low-res Coefficient Estimation Network (LCE-Net), to estimate the feature map in low-resolution space. Next, the High-res Illumination Estimation Module (HIE Module) is proposed to perform bilateral-grid-based upsampling for obtaining the high-res illumination. The illumination is finally utilized to light up the low-light image, yielding the enhanced image. MaCo is efficient and consumes fewer resources, as most computations occur in low resolution and the network is lightweight. Current LLIE datasets are mostly synthesized or generated through altered camera settings. These datasets are often limited in accurately representing real-world situations. To address this problem, we create a new dataset, IOLD, containing 572 images captured under real low- and normal-light conditions. In particular, the potential advantage of MaCo for face detection in the dark is also discussed. Extensive qualitative and quantitative experiments demonstrate that our method performs favorably against state-of-the-art methods in terms of effectiveness and efficiency. The IOLD dataset will be made publicly available at https://drive.google.com/drive/folders/1VAkuj9gheZ4aPEhBZ5MszHF6zpbrE_9_?usp=sharing .
更多查看译文
关键词
Low-light image enhancement (LLIE),Unsupervised learning,Magnitude control,High computational efficiency
AI 理解论文
溯源树
样例
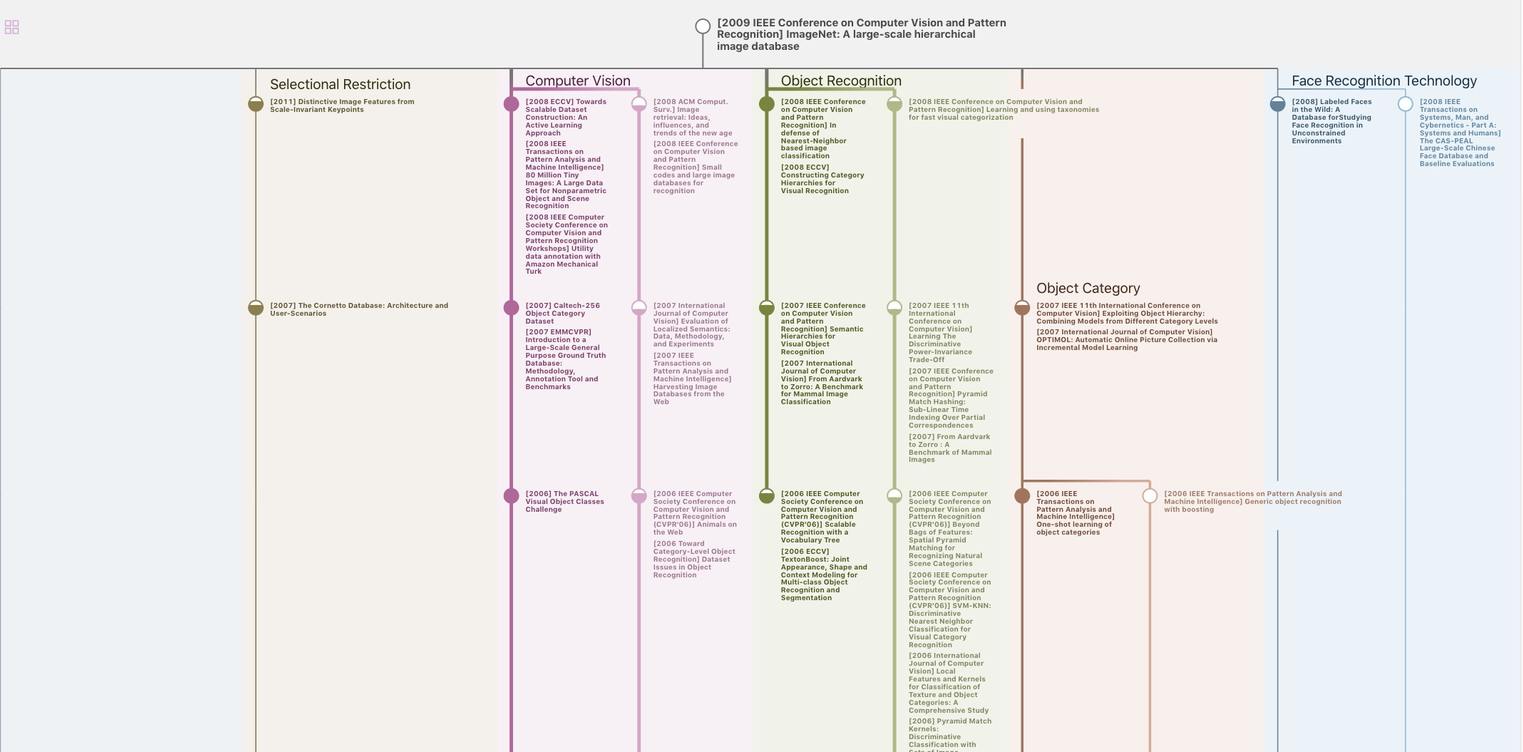
生成溯源树,研究论文发展脉络
Chat Paper
正在生成论文摘要