Universal and Scalable Weakly-Supervised Domain Adaptation
IEEE TRANSACTIONS ON IMAGE PROCESSING(2024)
摘要
Domain adaptation leverages labeled data from a source domain to learn an accurate classifier for an unlabeled target domain. Since the data collected in practical applications usually contain noise, the weakly-supervised domain adaptation algorithm has attracted widespread attention from researchers that tolerates the source domain with label noises or/and features noises. Several weakly-supervised domain adaptation methods have been proposed to mitigate the difficulty of obtaining the high-quality source domains that are highly related to the target domain. However, these methods assume to obtain the accurate noise rate in advance to reduce the negative transfer caused by noises in source domain, which limits the application of these methods in the real world where the noise rate is unknown. Meanwhile, since source data usually comes from multiple domains, the naive application of single-source domain adaptation algorithms may lead to sub-optimal results. We hence propose a universal and scalable weakly-supervised domain adaptation method called PDCAS to ease restraints of such assumptions and make it more general. Specifically, PDCAS includes two stages: progressive distillation and domain alignment. In progressive distillation stage, we iteratively distill out potentially clean samples whose annotated labels are highly consistent with the prediction of model and correct labels for noisy source samples. This process is non-supervision by exploiting intrinsic similarity to measure and extract initial corrected samples. In domain alignment stage, we consider Class-Aligned Sampling which balances the samples for both source and target domains along with the global feature distributions to alleviate the shift of label distributions. Finally, we apply PDCAS in multi-source noisy scenario and propose a novel multi-source weakly-supervised domain adaptation method called MSPDCAS, which shows the scalability of our framework. Extensive experiments on Office-31 and Office-Home datasets demonstrate the effectiveness and robustness of our method compared to state-of-the-art methods.
更多查看译文
关键词
Weakly-supervised domain adaptation,noises,adversarial learning,pseudo-labels
AI 理解论文
溯源树
样例
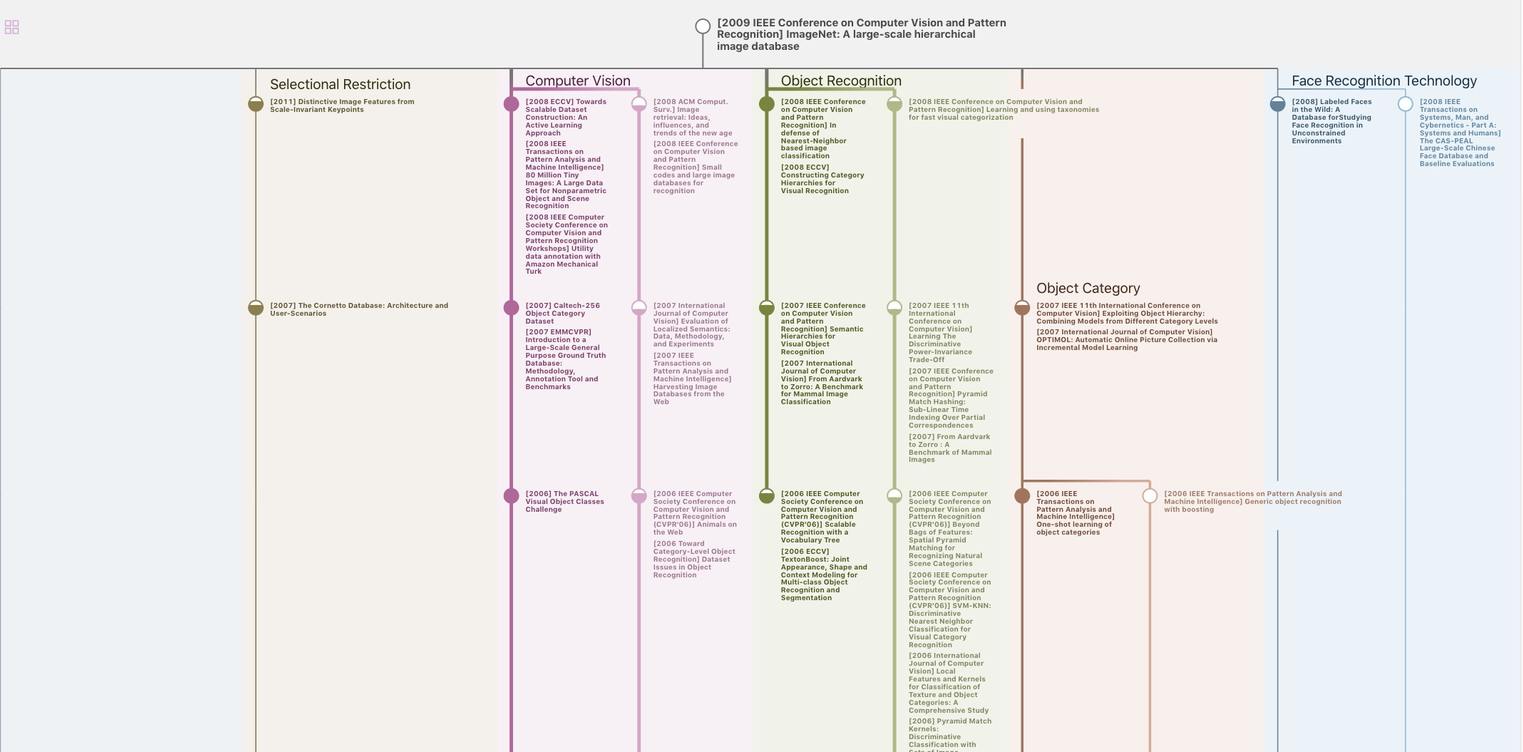
生成溯源树,研究论文发展脉络
Chat Paper
正在生成论文摘要