LSTM-Based GNSS Localization Using Satellite Measurement Features Jointly with Pseudorange Residuals
SENSORS(2024)
摘要
In the Global Navigation Satellite System (GNSS) context, the growing number of available satellites has led to many challenges when it comes to choosing the most-accurate pseudorange contributions, given the strong impact of biased measurements on positioning accuracy, particularly in single-epoch scenarios. This work leverages the potential of machine learning in predicting linkwise measurement quality factors and, hence, optimize measurement weighting. For this purpose, we used a customized matrix composed of heterogeneous features such as conditional pseudorange residuals and per-link satellite metrics (e.g., carrier-to-noise-power-density ratio and its empirical statistics, satellite elevation, carrier phase lock time). This matrix is then fed as an input to a long short-term memory (LSTM) deep neural network capable of exploiting the hidden correlations between these features relevant to positioning, leading to the predictions of efficient measurement weights. Our extensive experimental results on real data, obtained from extensive field measurements, demonstrate the high potential of our proposed solution, which is able to outperform traditional measurement weighting and selection strategies from the state-of-the-art. In addition, we included detailed illustrations based on representative sessions to provide a concrete understanding of the significant gains of our approach, particularly in strongly GNSS-challenged operating conditions.
更多查看译文
关键词
satellite selection,single-epoch positioning,machine (deep) learning,long short-term memory neural network,satellite measurement features
AI 理解论文
溯源树
样例
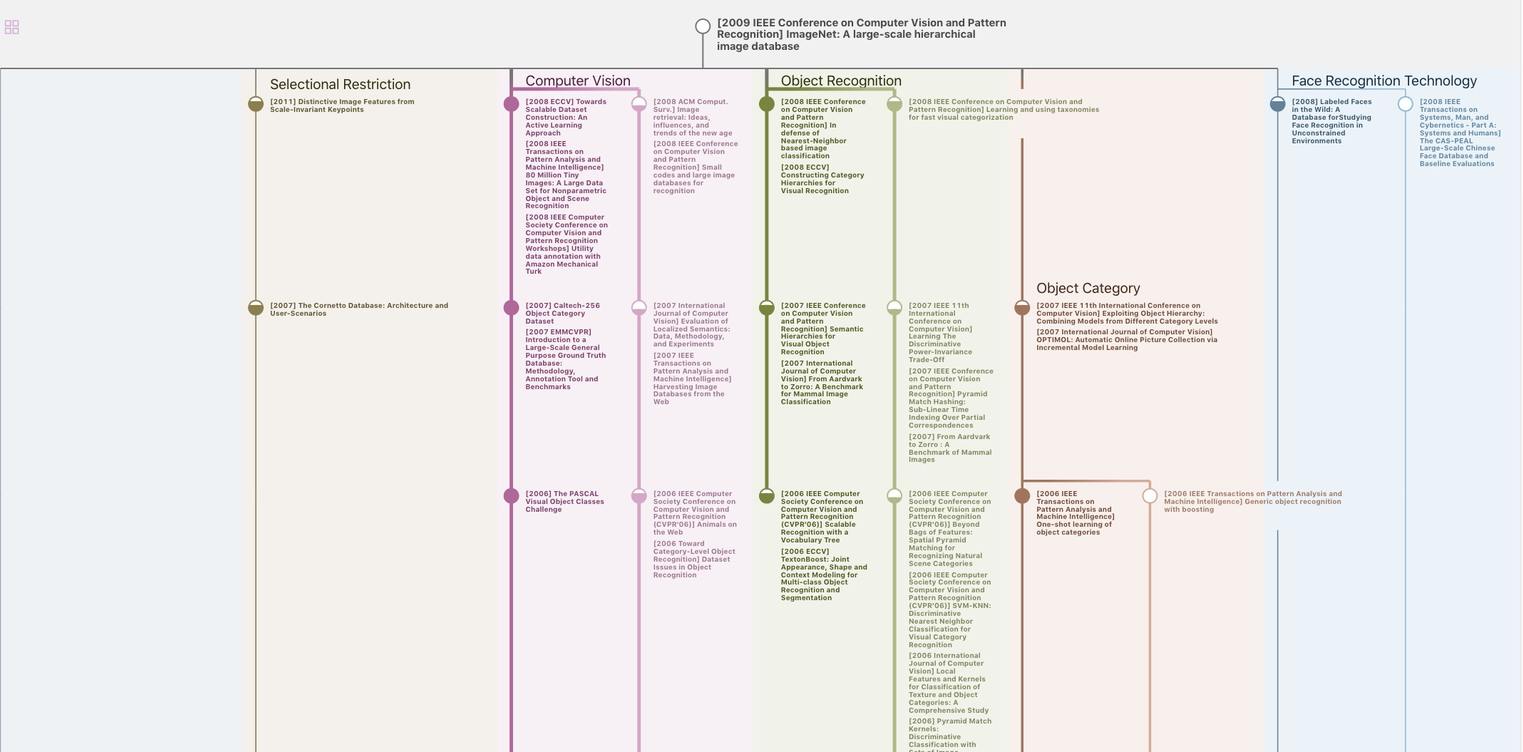
生成溯源树,研究论文发展脉络
Chat Paper
正在生成论文摘要