Vehicular communication using federated learning empowered chimp optimization (FLECO) algorithm
Multimedia Tools and Applications(2024)
摘要
Recently in the field of vehicular communication, there has been a concentration of research on the integration of a vehicle-to-vehicle (V2V) network. With vehicle-to-vehicle (V2V) communication, users can directly exchange significant information with nearby vehicles. Typically, automobiles tend to travel at higher speeds on highways compared to roads at intersections. As a result, it is necessary to have a reliable system in place that can effectively and securely facilitate communication. In recent times, scientists have developed different methods for distributing information. However, these systems have various issues such as latency, reliability, mobility, and communication cost. Consequently, this results in a lack of dependability for real-time communication. Therefore, this study introduces a novel approach to Federated Learning (FL) by including the Chimp Optimization Algorithm (ChOA). Federated Learning is an approach in the field of machine learning that enables multiple devices or nodes to collaboratively train a model without the need for data exchange. In the area of vehicular communication, utilization of Federated Learning can be employed to develop a predictive model that estimates the trajectory of nearby vehicles by utilizing collected data. The Chimp Optimization Algorithm (ChOA) is designed to improve the model's efficacy. The proposed method aims to enhance the accuracy of the model's predictions regarding the conduct of nearby vehicles, while also reducing the amount of data exchanged between vehicles, by combining Federated Learning and Chimp Optimization termed FLECO. This method has the potential to enhance vehicular communication effectiveness and security, while also improving road safety and traffic management. Federated Learning facilitates the group control of a machine learning (ML) system by vehicles through the adjustment of model parameters. To enhance the energy efficiency of the system, the implementation of resource allocation and an energy-efficient algorithm is employed for Federated Learning, which integrates power and time allocation methods. This paper conducts a comprehensive analysis of the impact of enabling re-routing capabilities on (i) the mobility of vehicles and (ii) Networks for predicting traffic. To achieve this, utilize the SUMO simulator for road traffic to generate vehicle trajectories. Subsequently, we evaluate the vehicular network's connectivity employing established graph metrics. The developed system is simulated using the Python tool and experimentally validated, demonstrating its effective accuracy in vehicular communication.
更多查看译文
关键词
Federated learning,Vehicle communication,Chimp optimization algorithm,SUMO,FLECO
AI 理解论文
溯源树
样例
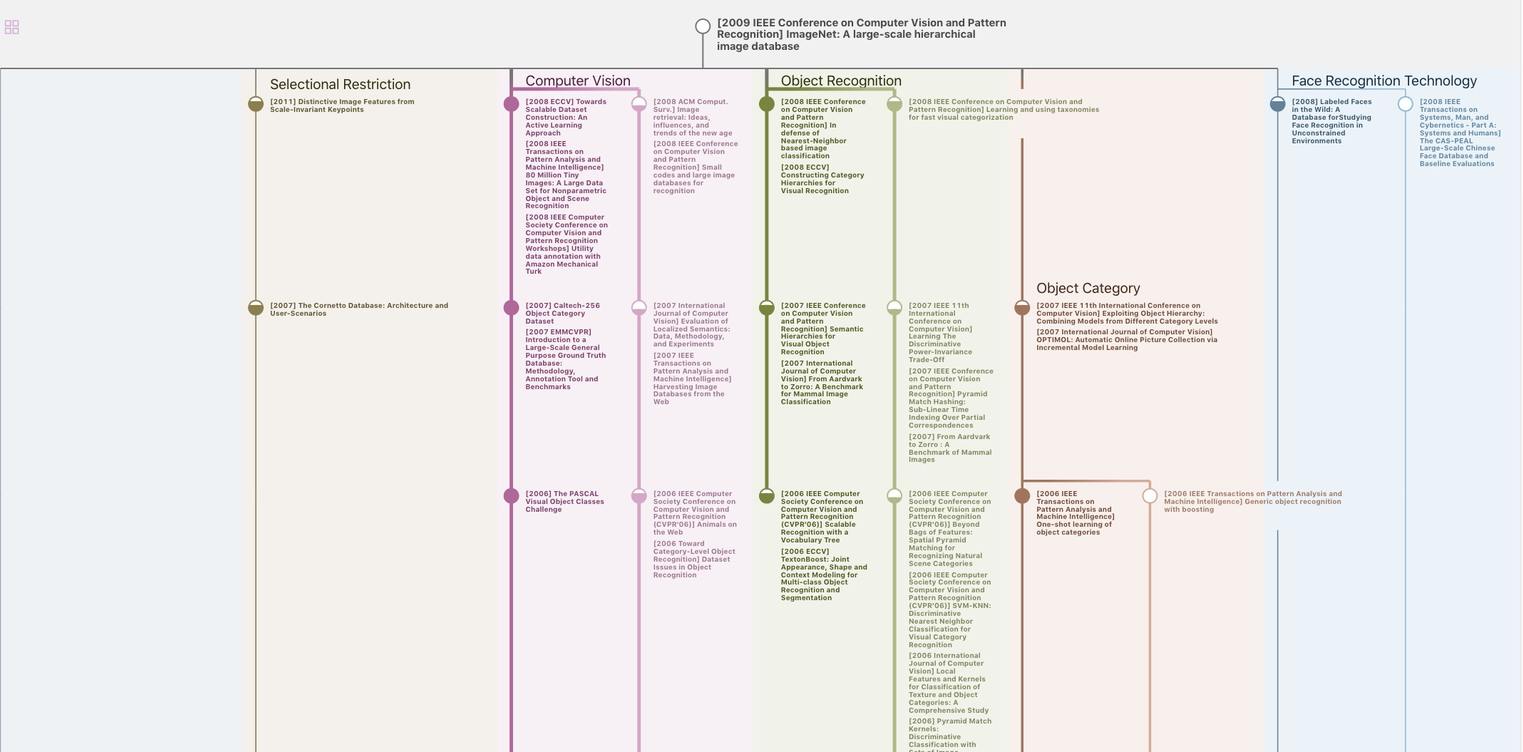
生成溯源树,研究论文发展脉络
Chat Paper
正在生成论文摘要