A bilateral heterogeneous graph model for interpretable job recommendation considering both reciprocity and competition
Frontiers of Engineering Management(2024)
摘要
Amidst the inefficiencies of traditional job-seeking approaches in the recruitment ecosystem, the importance of automated job recommendation systems has been magnified. However, existing models optimized to maximize user clicks for general product recommendations prove inept in addressing the unique challenges of job recommendation, namely reciprocity and competition. Moreover, sparse data on online recruitment platforms can further negatively impact the performance of existing job recommendation algorithms. To counteract these limitations, we propose a bilateral heterogeneous graph-based competition iteration model. This model comprises three integral components: 1) two bilateral heterogeneous graphs for capturing multi-source information from people and jobs and alleviating data sparsity, 2) fusion strategies for synthesizing attributes and preferences to produce mutually beneficial job matches, and 3) a competition-enhancing strategy for dispersing competition realized through a two-stage optimization algorithm. Augmented by granular attention mechanisms for enhanced interpretability, the model’s efficacy, competition dispersion, and interpretability are validated through rigorous empirical evaluations on a real-world recruitment platform.
更多查看译文
关键词
job recommendation,competition,reciprocity,interpretability
AI 理解论文
溯源树
样例
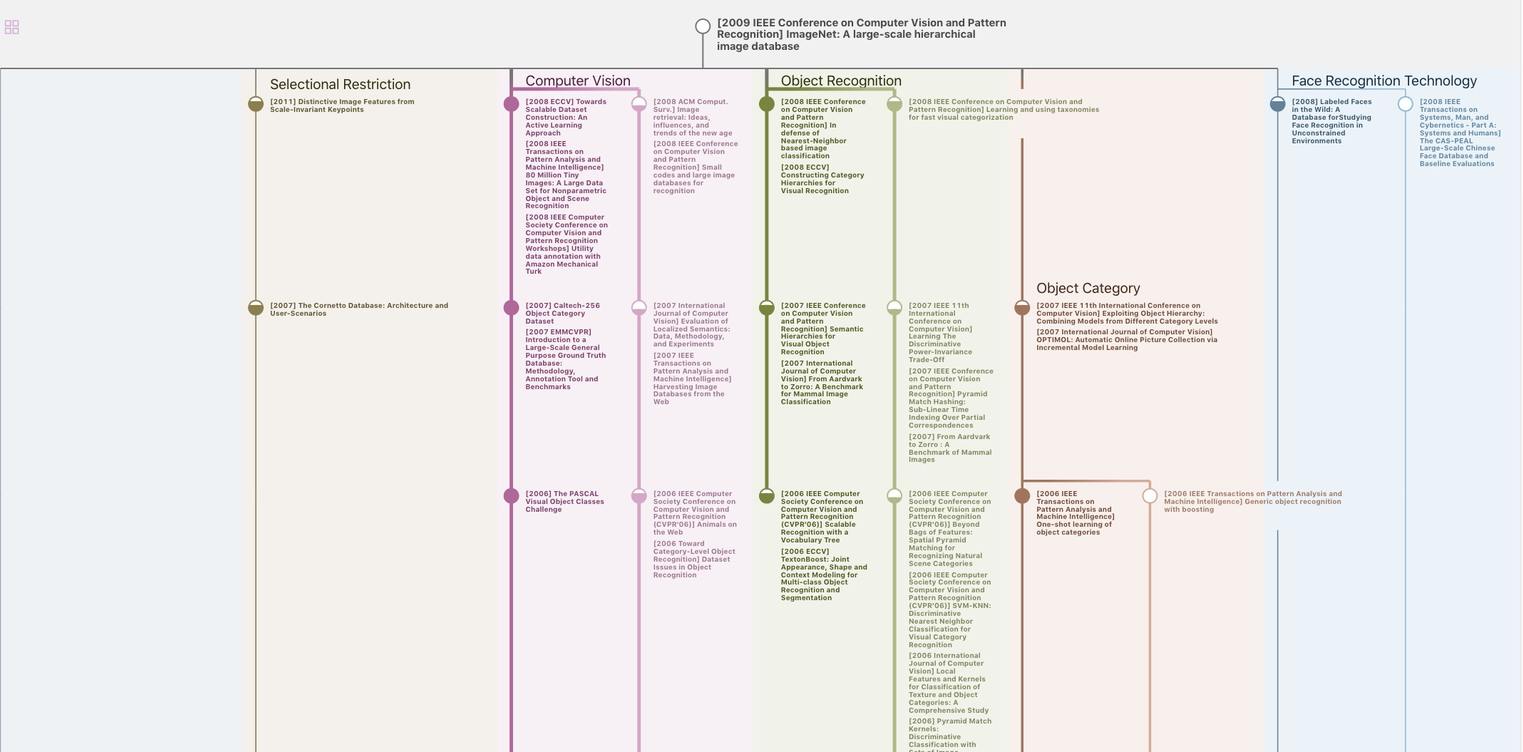
生成溯源树,研究论文发展脉络
Chat Paper
正在生成论文摘要