CF-GCN: Graph Convolutional Network for Change Detection in Remote Sensing Images.
IEEE Transactions on Geoscience and Remote Sensing(2024)
摘要
The remote sensing image change detection methods based on deep learning have made great progress. However, many convolutional neural network (CNN)-based methods persistently face challenges in connecting long-range semantic concepts because of their limited receptive fields. Recently, some methods that combine transformers effectively extract global information by modeling the context in the temporal and spatial domains have been proposed to solve the problem, but they still suffer from both the incorrect identification of “non-semantic changes” and the incomplete and irregular boundary extraction due to the deterioration of local feature details. In response to these inquiries, we propose a novel network, coordinate space and feature interaction-graph convolutional network (CF-GCN), based on graph convolutional structures for change detection. Specifically, in the encoder and decoder of the network, different projection strategies are employed to construct a coordinate space graph convolution network (GCN_C) and feature interaction graph convolution network (GCN_F). The boundary perception module (BPM) extracts spatial boundary features of shallow layers and enhances boundary perception ability during graph-based information propagation, effectively suppressing the tendency of image boundary information to gradually smooth out. At the same time, the knowledge review module (KRM) is utilized to form knowledge complementarity between key layers of the network, effectively mitigating the propagation of erroneous knowledge in the deep network. On the LEVIR-CD dataset, the intersection over union (IoU) score of CF-GCN is 83.41%, which is 0.35% and 0.39% higher than ChangeStar and DMINet, respectively. On the WHU-CD dataset, the
${F}1$
and IoU are as high as 91.83% and 84.90%, which are significantly better than other state-of-the-art (SOTA) networks. The experimental results show that, in addition to CNN and Transformer, the graph-convolution structure approach is expected to be another major research direction for performing fully supervised change detection. Our code and pretrained models will be available at
https://github.com/liucongcharles/CF-GCN
.
更多查看译文
关键词
Boundary perception,change detection,graph convolutional network (GCN),remote sensing images
AI 理解论文
溯源树
样例
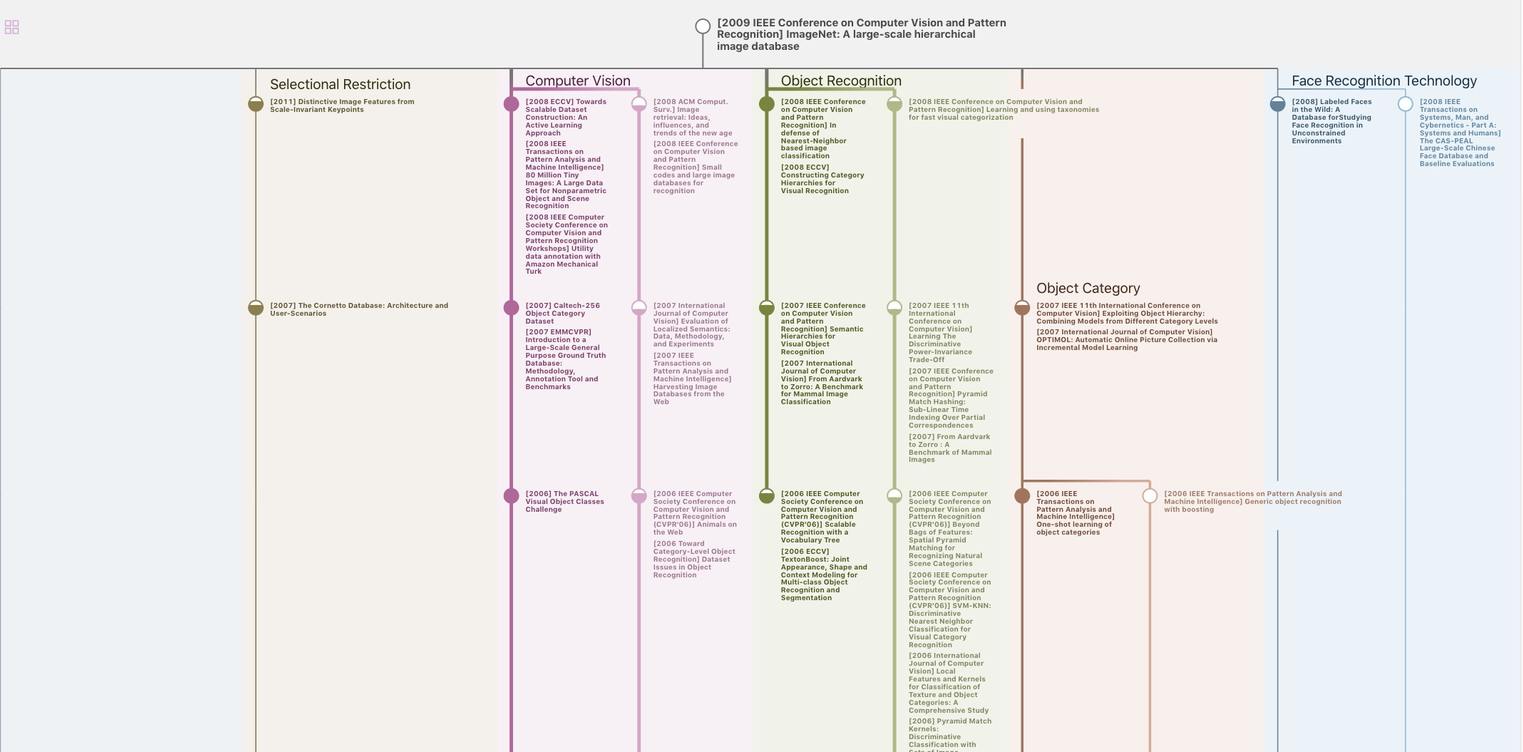
生成溯源树,研究论文发展脉络
Chat Paper
正在生成论文摘要