BotSSCL: Social Bot Detection with Self-Supervised Contrastive Learning
CoRR(2024)
摘要
The detection of automated accounts, also known as "social bots", has been an
increasingly important concern for online social networks (OSNs). While several
methods have been proposed for detecting social bots, significant research gaps
remain. First, current models exhibit limitations in detecting sophisticated
bots that aim to mimic genuine OSN users. Second, these methods often rely on
simplistic profile features, which are susceptible to manipulation. In addition
to their vulnerability to adversarial manipulations, these models lack
generalizability, resulting in subpar performance when trained on one dataset
and tested on another.
To address these challenges, we propose a novel framework for social Bot
detection with Self-Supervised Contrastive Learning (BotSSCL). Our framework
leverages contrastive learning to distinguish between social bots and humans in
the embedding space to improve linear separability. The high-level
representations derived by BotSSCL enhance its resilience to variations in data
distribution and ensure generalizability. We evaluate BotSSCL's robustness
against adversarial attempts to manipulate bot accounts to evade detection.
Experiments on two datasets featuring sophisticated bots demonstrate that
BotSSCL outperforms other supervised, unsupervised, and self-supervised
baseline methods. We achieve approx. 6
than SOTA on both datasets. In addition, BotSSCL also achieves 67
trained on one dataset and tested with another, demonstrating its
generalizability. Lastly, BotSSCL increases adversarial complexity and only
allows 4
更多查看译文
AI 理解论文
溯源树
样例
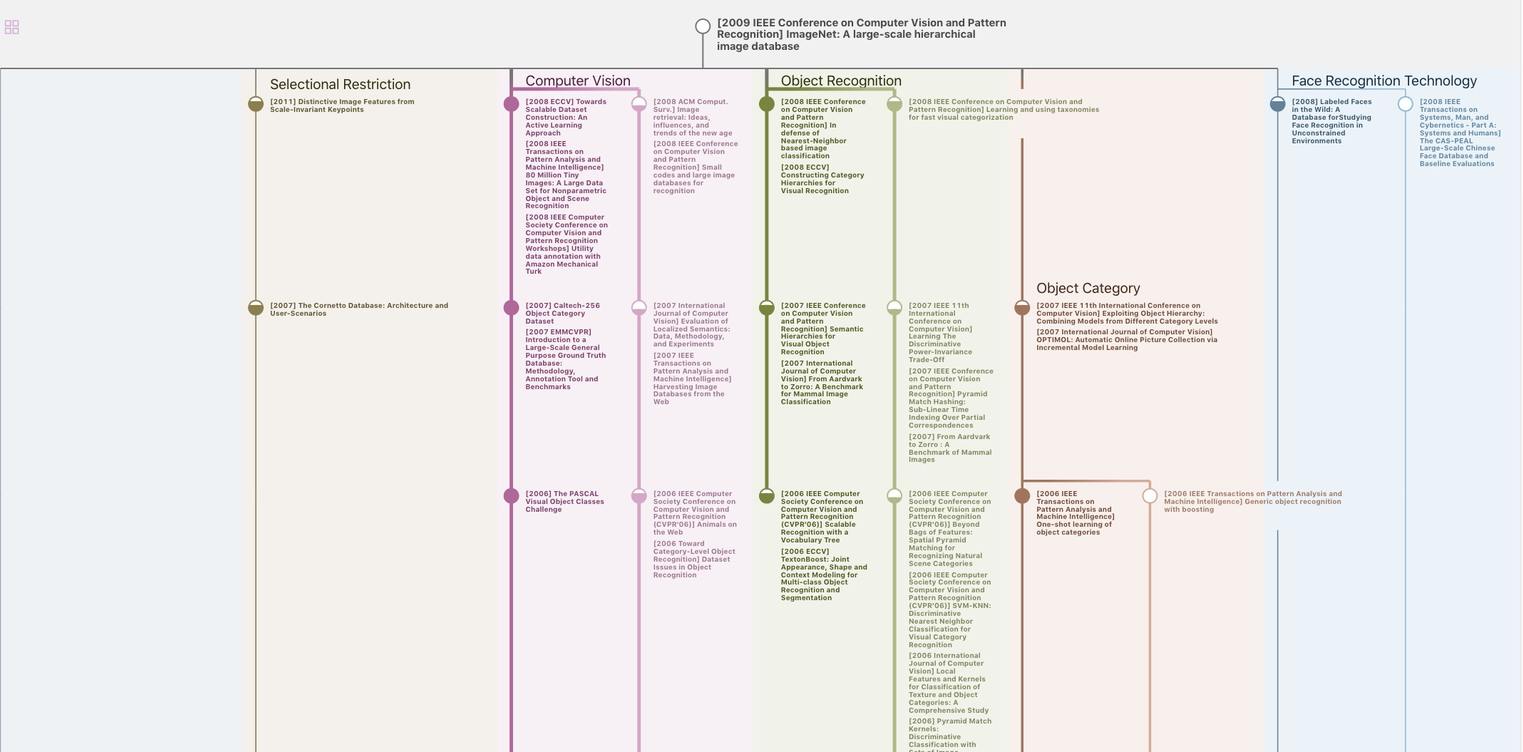
生成溯源树,研究论文发展脉络
Chat Paper
正在生成论文摘要