Revealing genomic heterogeneity and commonality: A penalized integrative analysis approach accounting for the adjacency structure of measurements
GENETIC EPIDEMIOLOGY(2024)
摘要
Advancements in high-throughput genomic technologies have revolutionized the field of disease biomarker identification by providing large-scale genomic data. There is an increasing focus on understanding the relationships among diverse patient groups with distinct disease subtypes and characteristics. Complex diseases exhibit both heterogeneity and shared genomic factors, making it essential to investigate these patterns to accurately detect markers and comprehensively understand the diseases. Integrative analysis has emerged as a promising approach to address this challenge. However, existing studies have been limited by ignoring the adjacency structure of genomic measurements, such as single nucleotide polymorphisms (SNPs) and DNA methylations. In this study, we propose a structured integrative analysis method that incorporates a spline type penalty to accommodate this adjacency structure. We utilize a fused lasso type penalty to identify both heterogeneity and commonality across the groups. Extensive simulations demonstrate its superiority compared to several direct competing methods. The analysis of The Cancer Genome Atlas melanoma data with DNA methylation measurements and GENEVA diabetes data with SNP measurements exhibit that the proposed analysis lead to meaningful findings with better prediction performance and higher selection stability.
更多查看译文
关键词
adjacency structure,genomics,heterogeneity and commonality,integrative analysis
AI 理解论文
溯源树
样例
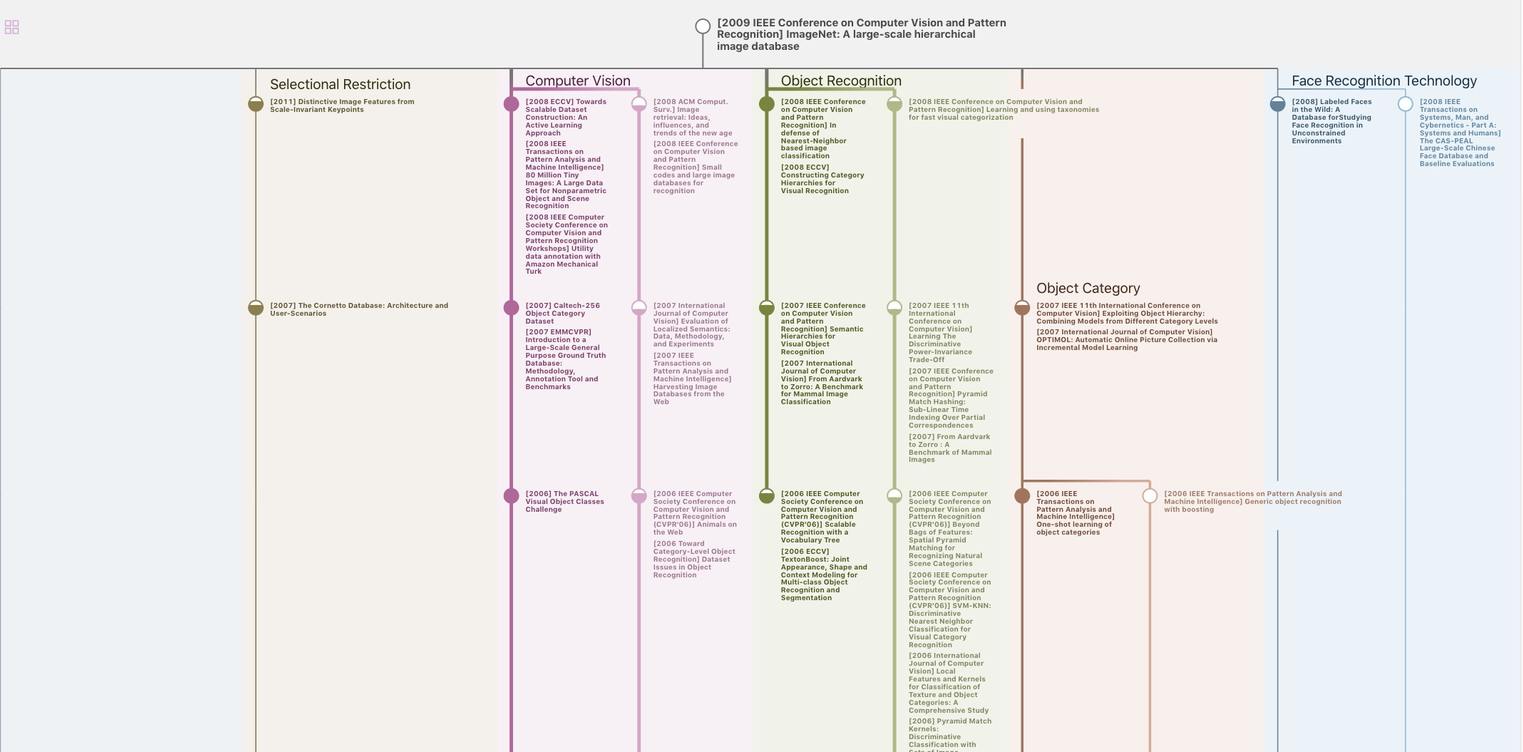
生成溯源树,研究论文发展脉络
Chat Paper
正在生成论文摘要