Revisiting Generative Adversarial Networks for Binary Semantic Segmentation on Imbalanced Datasets
CoRR(2024)
摘要
Anomalous pavement surface conditions detection aims to detect pixels
representing anomalous states, such as cracks, on pavement surface images
automatically by algorithms. Recently, deep learning models have been
intensively applied to related topics with outstanding performance. However,
most existing deep learning-related solutions rarely achieve a stable
performance on diverse datasets. To address this issue, in this work, we
propose a deep learning framework based on conditional Generative Adversarial
Networks for anomalous region detection on pavement images at the pixel level.
In particular, the proposed framework is developed to enhance the generator's
ability to estimate the probability feature map from heterogeneous inputs with
two training stages and multiscale feature representation. Moreover, several
attention mechanisms are incorporated into the proposed framework to mitigate
the performance deterioration of model training on severely imbalanced
datasets. We implement experiments on six accessible pavement datasets.
Extensive qualitative and quantitative experiments demonstrate that the
proposed framework can achieve SOTA results on these datasets efficiently and
robustly.
更多查看译文
AI 理解论文
溯源树
样例
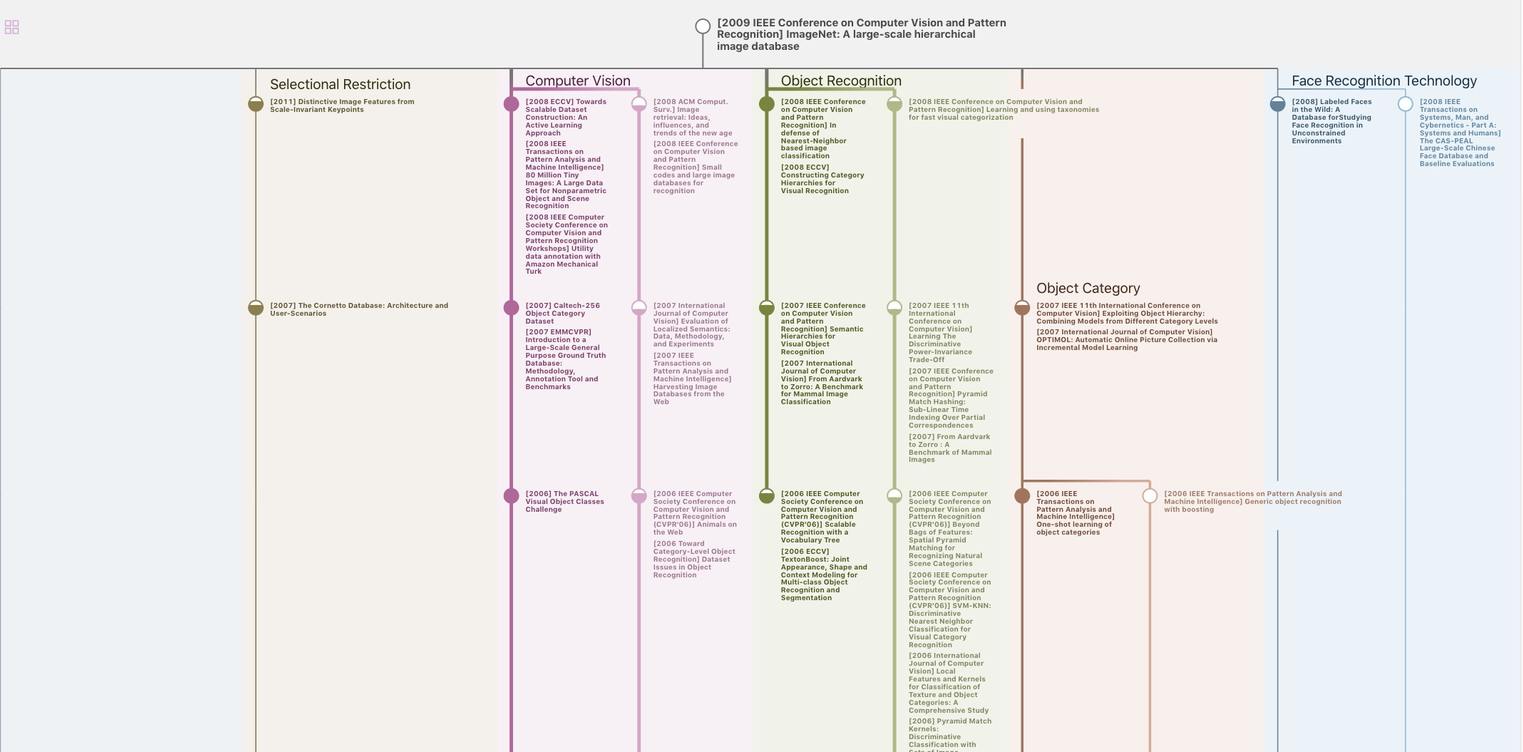
生成溯源树,研究论文发展脉络
Chat Paper
正在生成论文摘要