Dive into the Chasm: Probing the Gap between In- and Cross-Topic Generalization
Conference of the European Chapter of the Association for Computational Linguistics(2024)
摘要
Pre-trained language models (LMs) perform well in In-Topic setups, where
training and testing data come from the same topics. However, they face
challenges in Cross-Topic scenarios where testing data is derived from distinct
topics – such as Gun Control. This study analyzes various LMs with three
probing-based experiments to shed light on the reasons behind the In- vs.
Cross-Topic generalization gap. Thereby, we demonstrate, for the first time,
that generalization gaps and the robustness of the embedding space vary
significantly across LMs. Additionally, we assess larger LMs and underscore the
relevance of our analysis for recent models. Overall, diverse pre-training
objectives, architectural regularization, or data deduplication contribute to
more robust LMs and diminish generalization gaps. Our research contributes to a
deeper understanding and comparison of language models across different
generalization scenarios.
更多查看译文
AI 理解论文
溯源树
样例
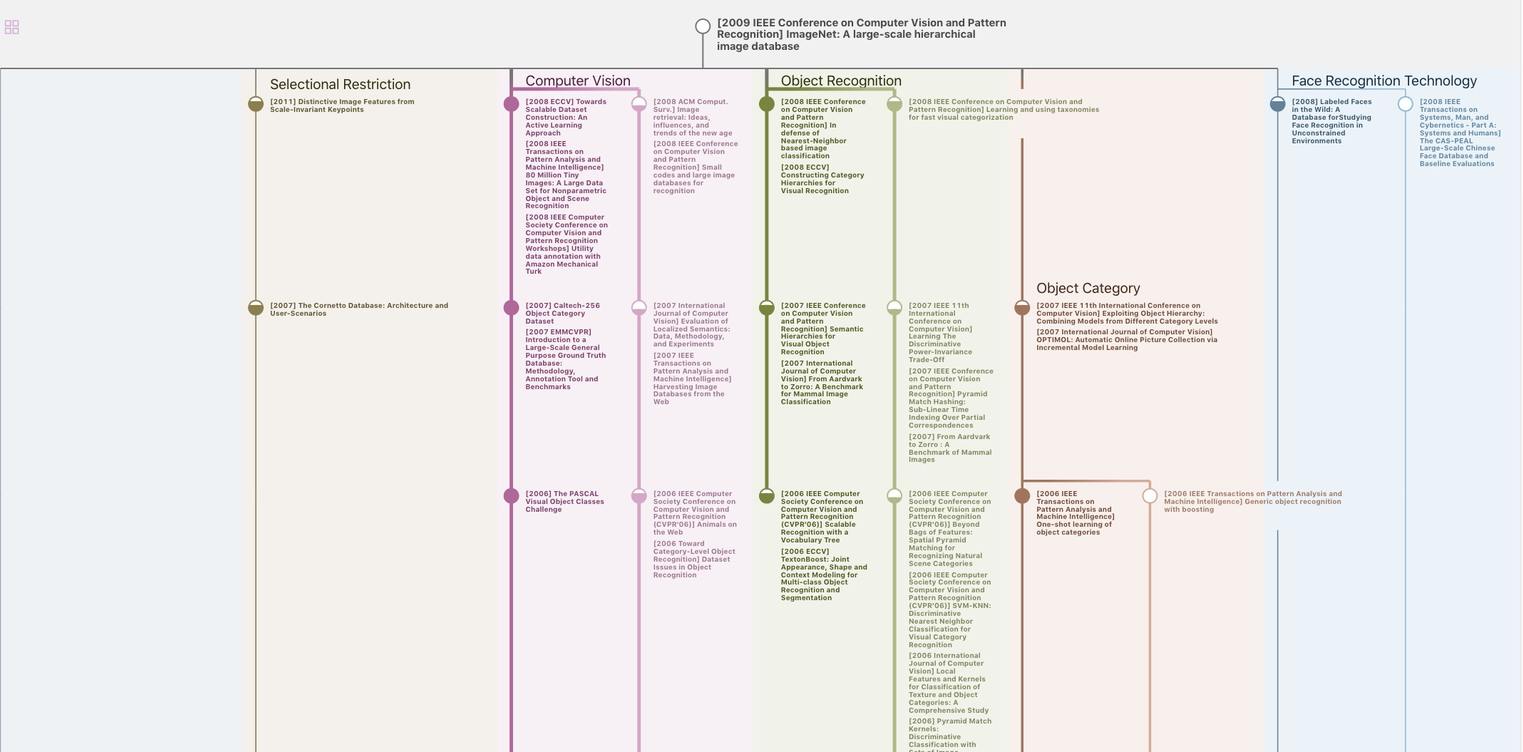
生成溯源树,研究论文发展脉络
Chat Paper
正在生成论文摘要