Eco-driving under localization uncertainty for connected vehicles on Urban roads: Data-driven approach and Experiment verification
CoRR(2024)
摘要
This paper addresses the eco-driving problem for connected vehicles on urban
roads, considering localization uncertainty. Eco-driving is defined as
longitudinal speed planning and control on roads with the presence of a
sequence of traffic lights. We solve the problem by using a data-driven model
predictive control (MPC) strategy. This approach involves learning a cost-to-go
function and constraints from state-input data. The cost-to-go function
represents the remaining energy-to-spend from the given state, and the
constraints ensure that the controlled vehicle passes the upcoming traffic
light timely while obeying traffic laws. The resulting convex optimization
problem has a short horizon and is amenable for real-time implementations. We
demonstrate the effectiveness of our approach through real-world vehicle
experiments. Our method demonstrates 12% improvement in energy efficiency
compared to the traditional approaches, which plan longitudinal speed by
solving a long-horizon optimal control problem and track the planned speed
using another controller, as evidenced by vehicle experiments.
更多查看译文
AI 理解论文
溯源树
样例
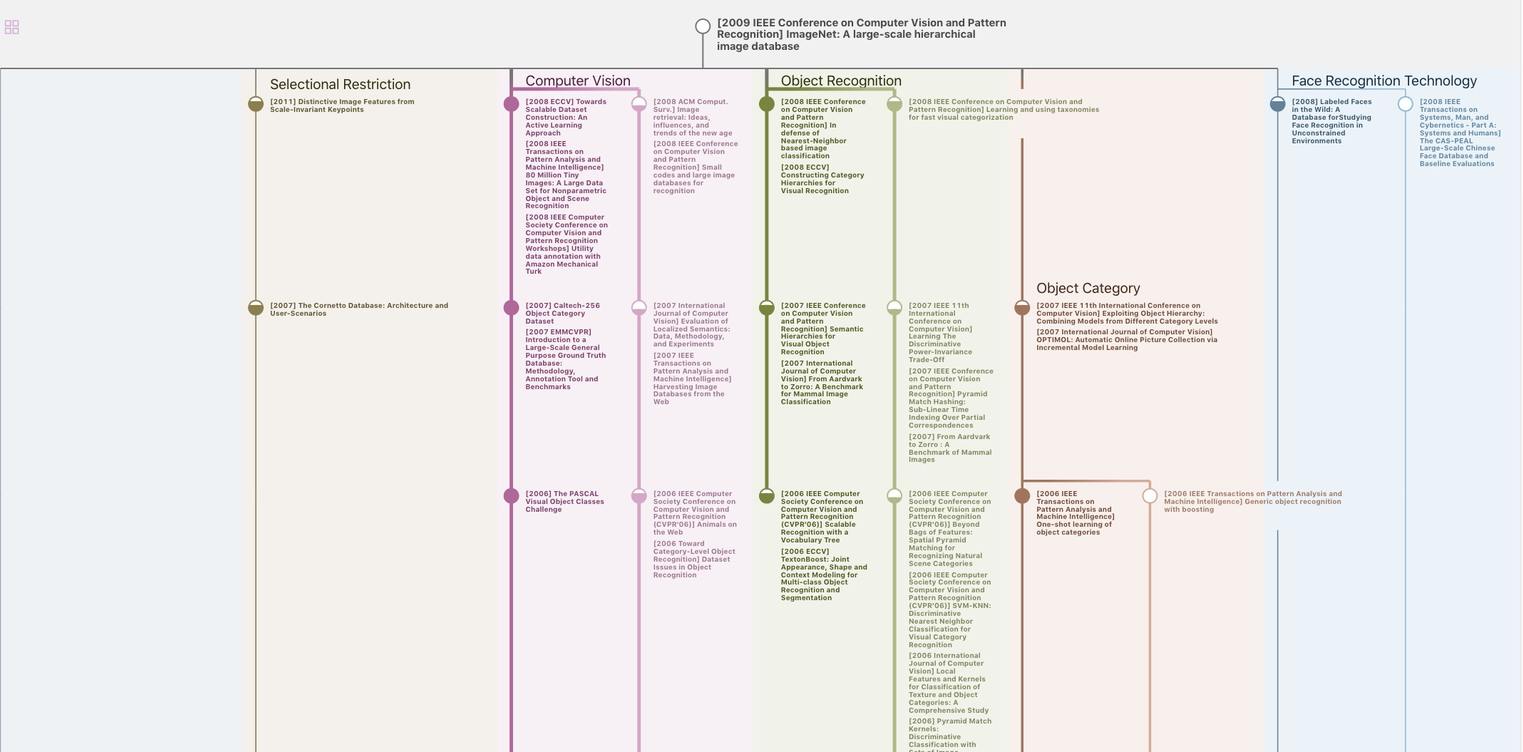
生成溯源树,研究论文发展脉络
Chat Paper
正在生成论文摘要