Disentangled body features for clothing change person re-identification
Multimedia Tools and Applications(2024)
摘要
With the rapid development of computer vision and deep learning technology, person re-identification(ReID) has attracted widespread attention as an important research area. Most current ReID methods primarily focus on short-term re-identification. In the scenario of pedestrian clothing changes, traditional ReID methods face some challenges due to significant changes in pedestrian appearance. Therefore, this paper proposes a clothes-changing person re-identification(CC-ReID) method, namely SViT-ReID, based on a Vision Transformer and incorporating semantic information. This method integrates semantic segmentation maps to more accurately extract features and representations of pedestrian instances in complex scenes, enabling the model to learn some clues unrelated to clothing. Specifically, we extract clothing-unrelated features (such as the face, arms, legs, and feet) from pedestrian parsing tasks’ obtained features. These features are then fused with global features to emphasize the importance of these body features. In addition, the complete semantic features derived from pedestrian parsing are fused with global features. These fused features undergo shuffle and grouping operations to generate local features, which are computed in parallel with global features, thereby enhancing the model’s robustness and accuracy. Experimental evaluations on two real-world benchmarks show the proposed SViT-ReID achieves state-of-the-art performance. Extensive ablation studies and visualizations illustrate the effectiveness of our method. Our method achieves a Top-1 accuracy of 55.2% and 43.4% on the PRCC and LTCC datasets, respectively.
更多查看译文
关键词
Person re-identification,Clothes-changing scenarios,Vision transformer,Semantic segmentation,Disentangled features
AI 理解论文
溯源树
样例
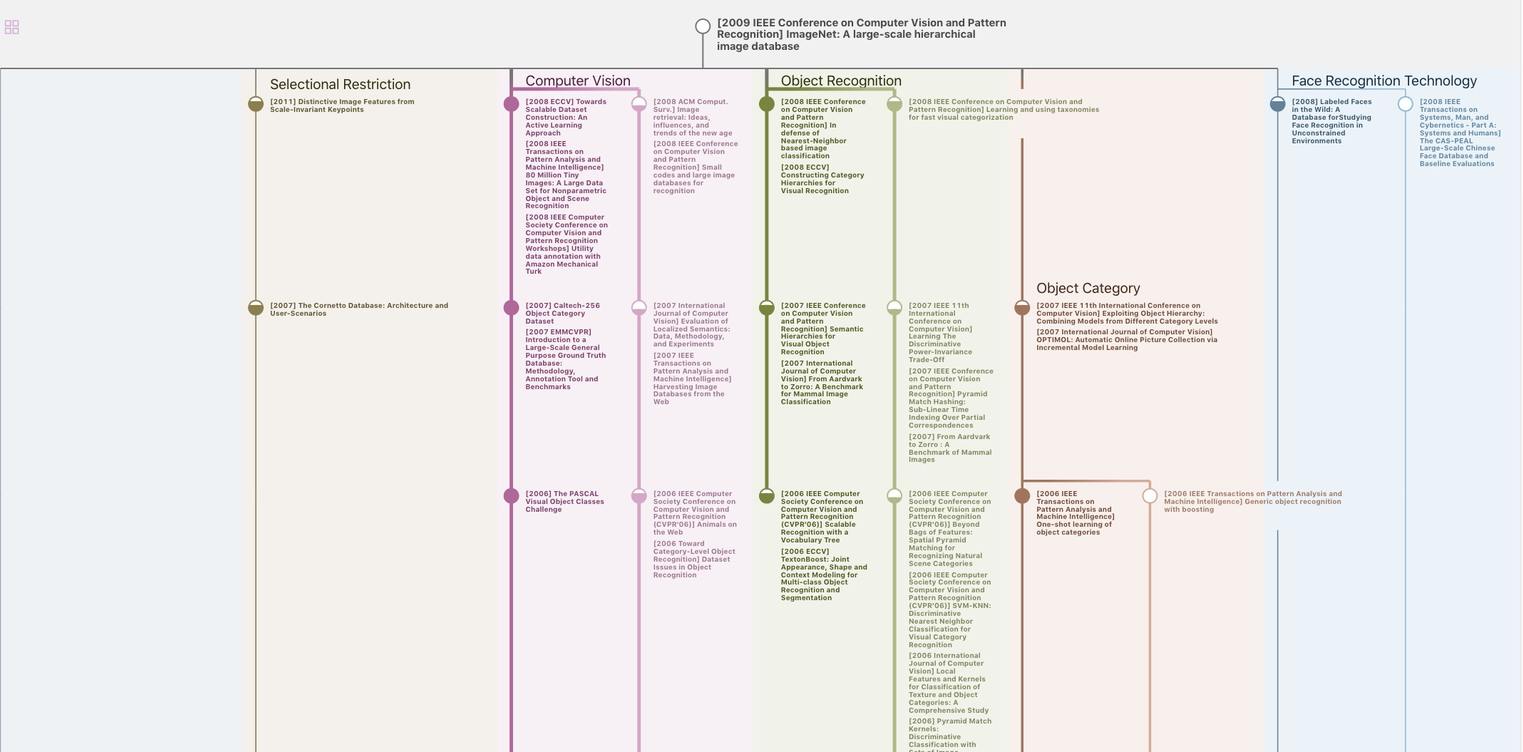
生成溯源树,研究论文发展脉络
Chat Paper
正在生成论文摘要