Closed-Loop In-Memory Computing for Energy-Efficient Matrix Eigendecomposition.
MetroXRAINE(2023)
摘要
To overcome the fundamental limitations of von Neumann-based computing system, in-memory computing (IMC) has been proposed as a potential candidate for next-generation computational architectures. In IMC, computation is performed in situ within the memory elements, thus eliminating the energy and latency overheads associated with continuous data transfer. IMC may be a key enabler for next-generation personal biometric monitoring systems by allowing low-power acceleration of machine learning (ML) techniques, among which principal component analysis (PCA) is extensively employed for classification and clustering with diagnostic purposes. Here, we show a novel closed-loop IMC circuit capable of performing matrix eigendecomposition, a core operation in PCA, describing the circuit's principle of operation and characterizing its performance in terms of speed and accuracy. As a real-world application, we perform in-memory classification on the Cleveland Heart Disease dataset, comparing the circuit performance with a commercial graphic processing unit. Simulation results show × 10
4
improvements in energy efficiency, with additional advantages in terms of throughput and area consumption, strengthening IMC's position as a promising candidate for next-generation low-power diagnostic devices.
更多查看译文
关键词
In-memory computing,resistive random access memory,hardware accelerator,principal component analysis
AI 理解论文
溯源树
样例
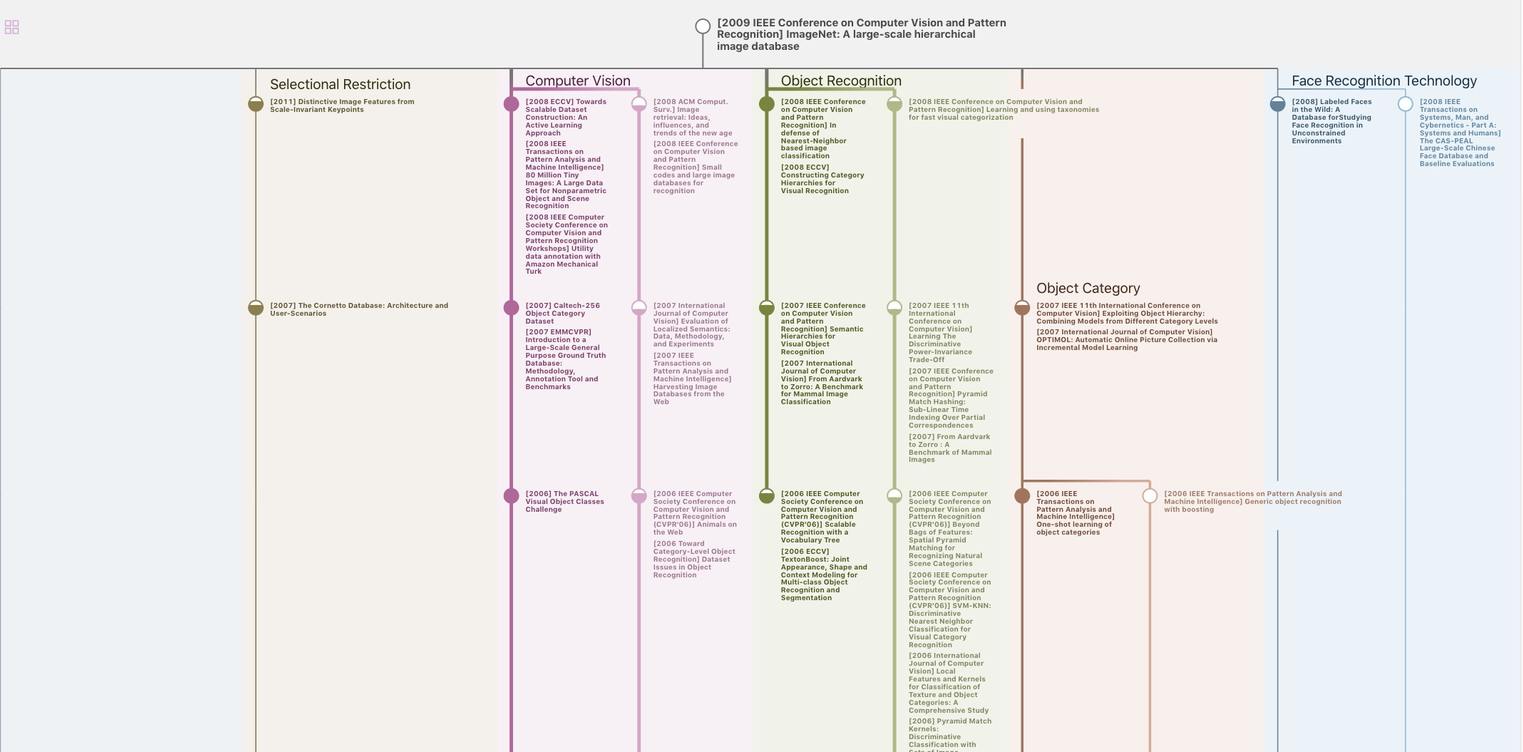
生成溯源树,研究论文发展脉络
Chat Paper
正在生成论文摘要