Uncertainty-Aware Guidance for Target Tracking subject to Intermittent Measurements using Motion Model Learning
CoRR(2024)
摘要
This letter presents a novel guidance law for target tracking applications
where the target motion model is unknown and sensor measurements are
intermittent due to unknown environmental conditions and low measurement update
rate. In this work, the target motion model is represented by a
transformer-based neural network and trained by previous target position
measurements. This neural network (NN)-based motion model serves as the
prediction step in a particle filter for target state estimation and
uncertainty quantification. Then this estimation uncertainty is utilized in the
information-driven guidance law to compute a path for the mobile agent to
travel to a position with maximum expected entropy reduction (EER). The
computation of EER is performed in real-time by approximating the probability
distribution of the state using the particle representation from particle
filter. Simulation and hardware experiments are performed with a quadcopter
agent and TurtleBot target to demonstrate that the presented guidance law
outperforms two other baseline guidance methods.
更多查看译文
AI 理解论文
溯源树
样例
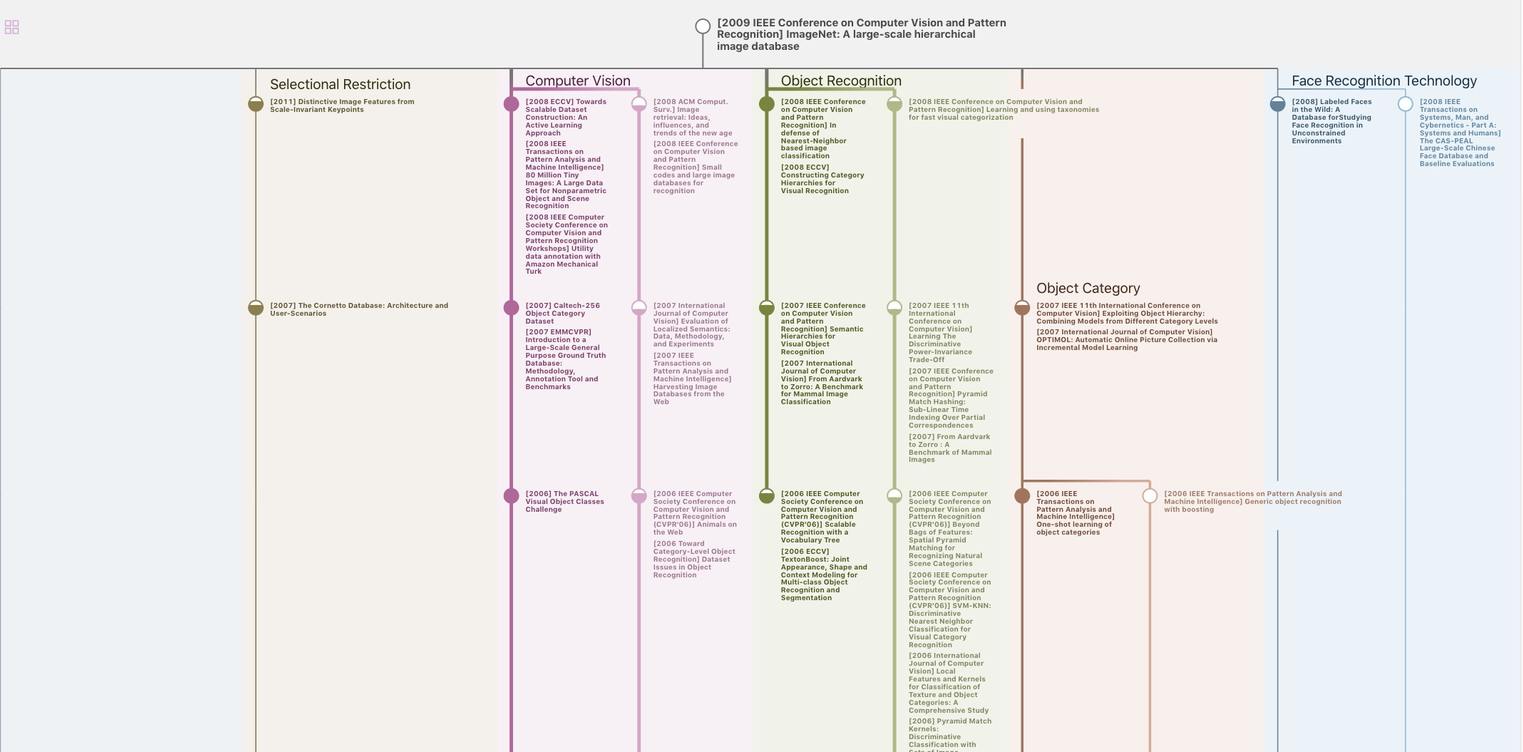
生成溯源树,研究论文发展脉络
Chat Paper
正在生成论文摘要