Stochastic Constraints: How Feasible Is Feasible?
Winter Simulation Conference(2023)
摘要
Stochastic constraints, which constrain an expectation in the context of simulation optimization, can be hard to conceptualize and harder still to assess. As with a deterministic constraint, a solution is considered either feasible or infeasible with respect to a stochastic constraint. This perspective belies the subjective nature of stochastic constraints, which often arise when attempting to avoid alternative optimization formulations with multiple objectives or an aggregate objective with weights. Moreover, a solution’s feasibility with respect to a stochastic constraint cannot, in general, be ascertained based on only a finite number of simulation replications. We introduce different means of estimating how "close" the expected performance of a given solution is to being feasible with respect to one or more stochastic constraints. We explore how these metrics and their bootstrapped error estimates can be incorporated into plots showing a solver’s progress over time when solving a stochastically constrained problem.
更多查看译文
关键词
Stochastic Constraints,Simulation Optimization,Replicate Simulations,Optimization Problem,Objective Function,Covariance Matrix,Feasible Solution,Components Of Vector,Boundary Region,Random Vector,Vector Function,Objective Function Value,Feasible Set,Normal Density,Progress Curve,Sample Covariance Matrix,Random Element,Value At Risk,Gaussian Random Vector,Conditional Value At Risk,Performance Of Solver,Plug-in Estimator,Adjacent Periods,Left-hand Side,Decision Variables,Mean Vector,End Of The Day,Random Variables,Constraint Violation,Working Hours
AI 理解论文
溯源树
样例
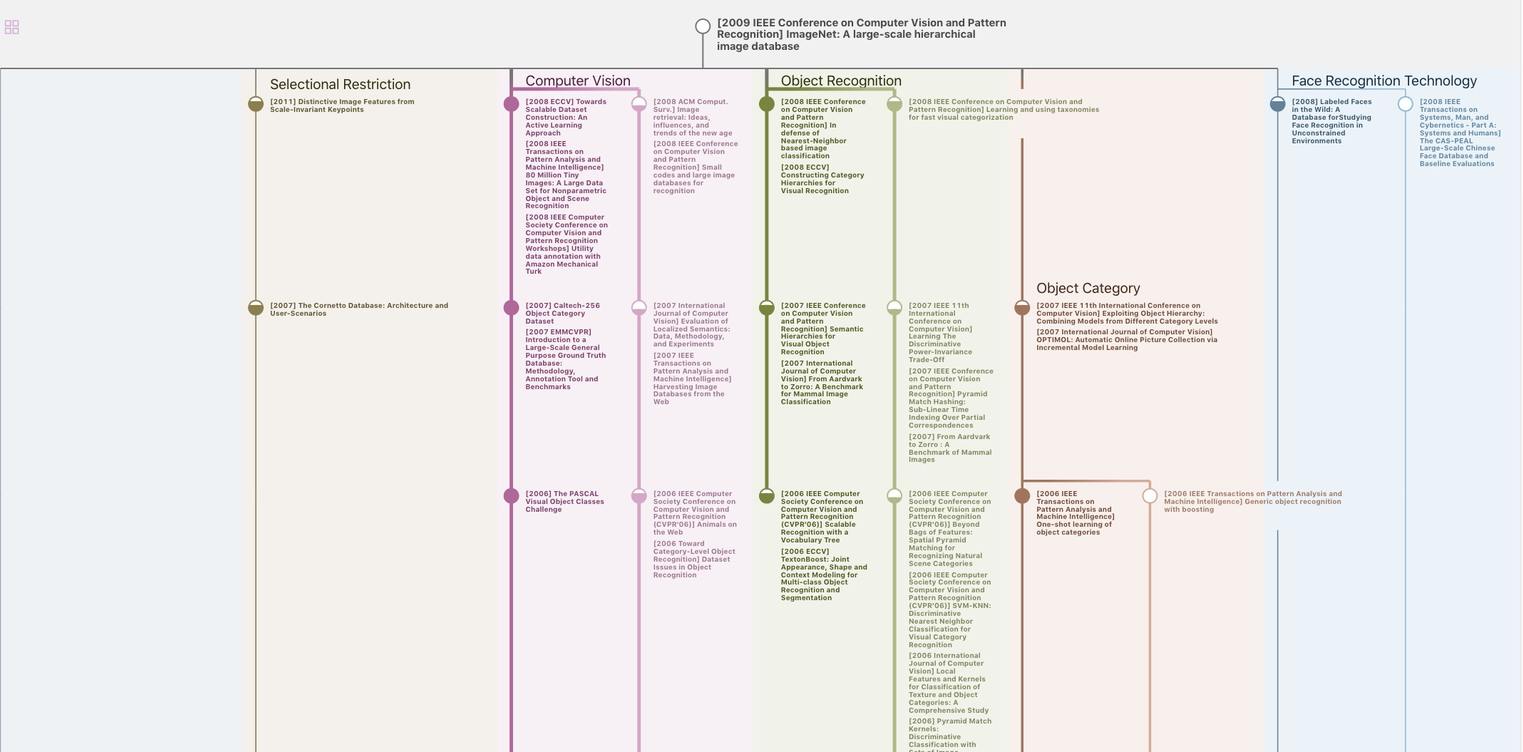
生成溯源树,研究论文发展脉络
Chat Paper
正在生成论文摘要