Variation-Guided Condition Generation for Diffusion Inversion in Few-Shot Image Classification
2023 International Conference on New Trends in Computational Intelligence (NTCI)(2023)
摘要
Few-shot learning (FSL) learns to recognize objects based on very limited examples of each category. An intuitive approach for FSL is to generate additional samples for few-shot categories. This is typically achieved by transferring general knowledge from the “many-shot” (base) categories. However, most existing methods do not fully exploit the intra-class variations within these categories, leading to limited diversity and quality among the generated samples. In this paper, we proposed a variation-guided condition generation network for diffusion Inversion in FSL, which not only learns to decompose embeddings obtained by the inversion into class-related and variation-related information to utilize abundant variations in the base dataset, but also incorporates the knowledge embedded in the general pre-trained Diffusion model into generated images. The proposed method consists of three steps. First, a conditional mapping network is trained by reversing each training image into the output space of the text encoder to obtain a set of embeddings as unique conditional vectors for each image. These vectors are then decomposed into class-related and variation-related conditions. The model learns to generate new conditional vectors by combining class-related and variation-related conditions from different categories. Finally, in the testing phase, the vectors are perturbed and denoised by Stable Diffusion to generate additional images for few-shot classes and help build few-shot classifiers. Experiments conducted on three popular few-shot datasets demonstrate that the proposed method can generate diverse and discriminative examples and significantly improve classification accuracy.
更多查看译文
关键词
image classification,image generation,few-shot learning,data augmentation
AI 理解论文
溯源树
样例
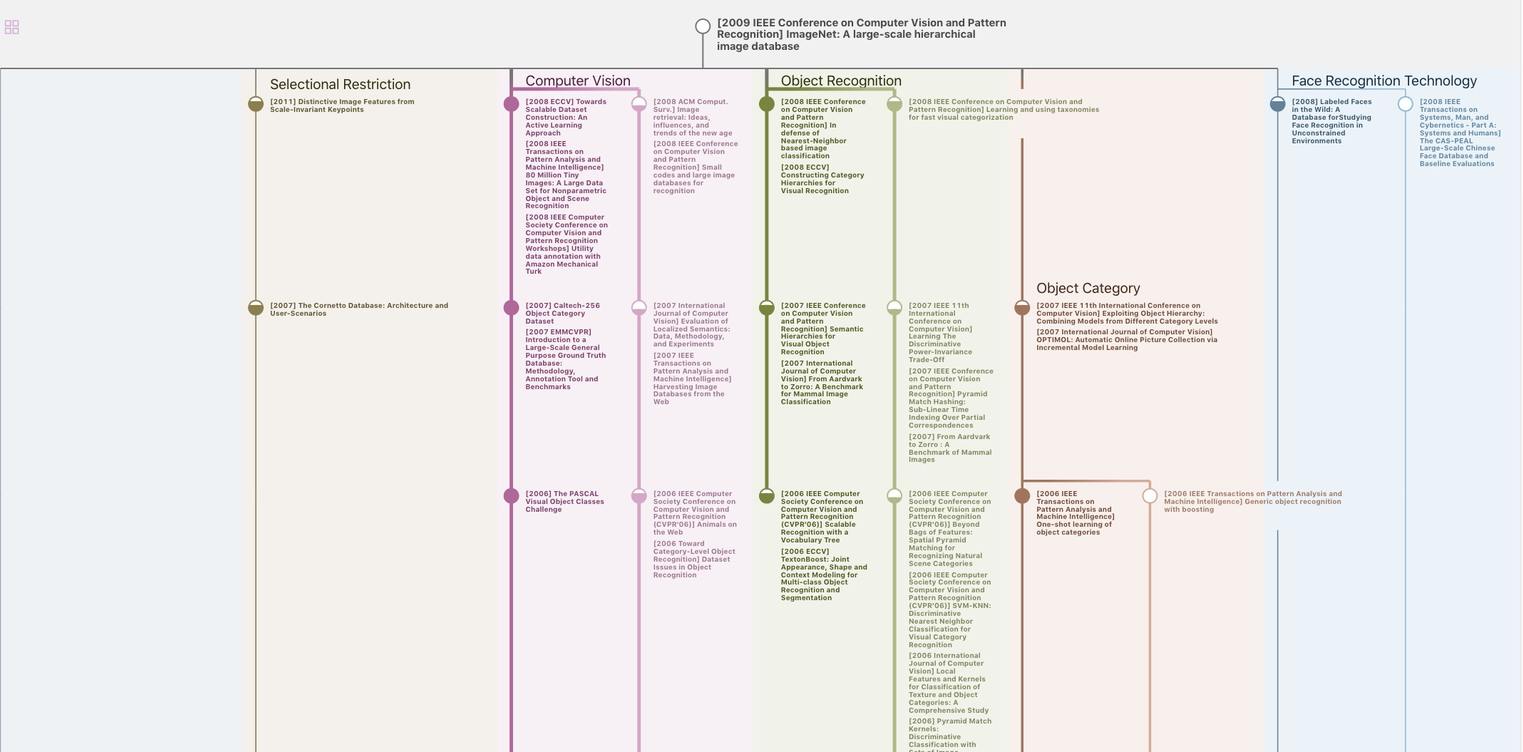
生成溯源树,研究论文发展脉络
Chat Paper
正在生成论文摘要