A-REA: Relation-Aware Graph Convolutional Network Entity Alignment Via a Differential Adjacency Matrix
2023 Asia-Pacific Conference on Image Processing, Electronics and Computers (IPEC)(2023)
摘要
Entity Alignment is the task of seeking entities with the same semantics from distinct knowledge graphs (KGs). Researchers have recently proposed Graph Convolutional Network (GCN) based approaches to achieve this task. Most existing GCN-based methods embed all the relations into an identical value, making the underlying loss on the diversity of the relationship. Relation semantics of entities and relation structure have benefitted the entity alignment task in the monolingual scenarios. This paper proposes a Relation-Aware Graph Convolutional Network with a Differential Adjacency Matrix (A-REA), which exploits the relation-specific semantics and structural information of entities in the KGs to promote the entity representations. The experiments on DBP-15K and DWY-100K datasets, including three cross-lingual subsets and two cross-knowledge graph sub-datasets, reveal that our approach leads to advance in entity alignment task in both the cross-lingual and cross-knowledge KGs. Discriminating the relation shows the effective signal for entity alignment, especially for the schema heterogeneity of different KGs.
更多查看译文
关键词
Entity Alignment,Knowledge Graph,Graph Convolutional Network,Relational Semantics
AI 理解论文
溯源树
样例
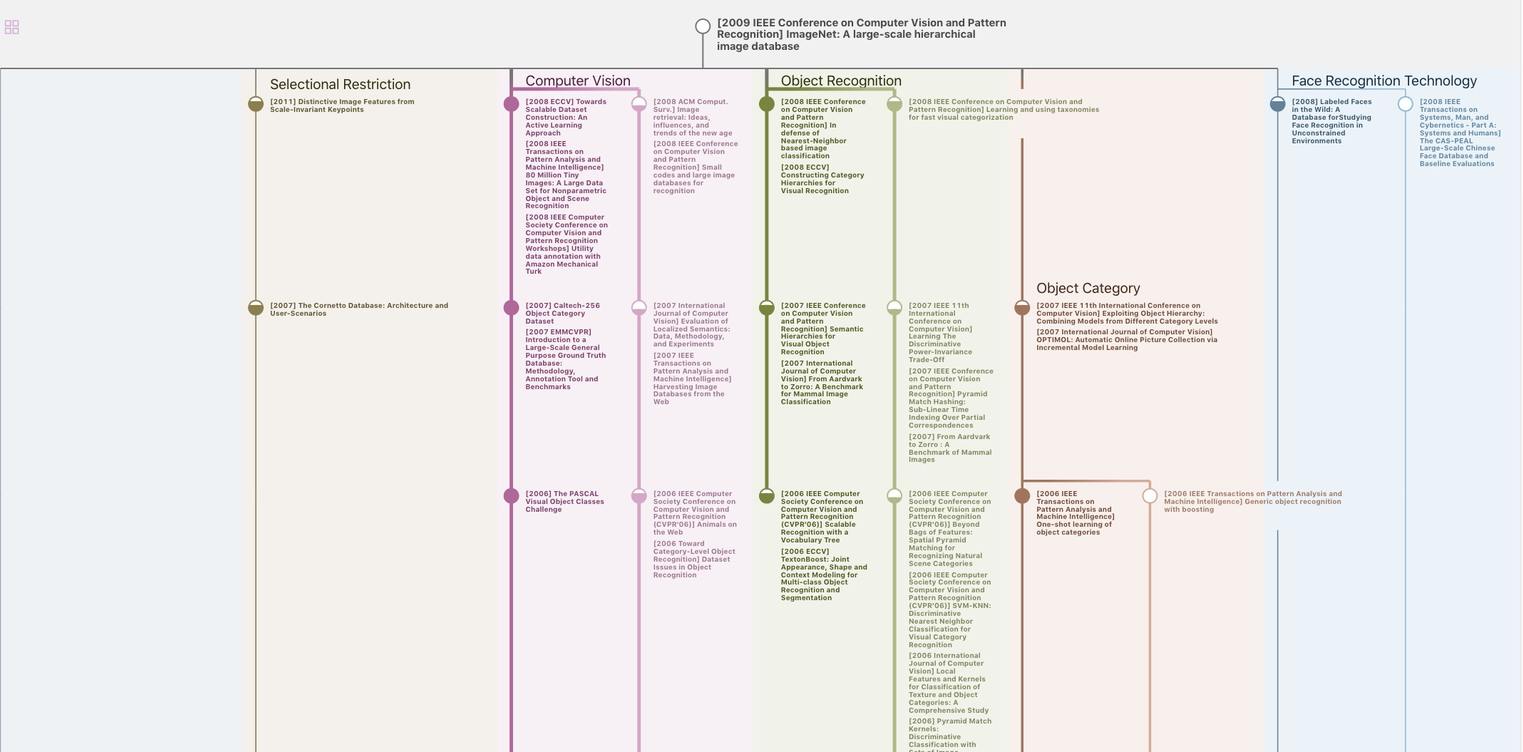
生成溯源树,研究论文发展脉络
Chat Paper
正在生成论文摘要