Object-based video anomaly detection using multi-attention and adaptive velocity attribute representation learning
Multimedia Systems(2024)
摘要
Video anomaly detection is an important topic in multimedia technology. Multiscale features and cross-learning between low-level and high-level features in the existing prediction models for anomaly detection are not exploited sufficiently, resulting in inadequate learning of object appearance and motion representations. In addition, the velocity attribute of the object is not been effectively utilized, resulting in inadequate learning of object motion information. To this end, a novel method for object-based video anomaly detection using multi-attention and adaptive velocity attribute representation learning is proposed. In this method, the MA-Unet (multiple attention Unet) model that incorporates channel attention, multi-scale spatial attention, and cross-semantic attention is constructed to learn object features effectively and capture more effective object appearance and motion information. Furthermore, the mechanism combining adaptive velocity attribute representation learning is proposed for anomaly discrimination, aiming to learn the velocity attributes of the object reasonably and better utilize the motion information of the object. Experiments conducted on the publicly available datasets USCDped2, Avenue, and ShanghaiTech show that our method outperforms state-of-the-art methods, which further validates the effectiveness of our method.
更多查看译文
关键词
Video anomaly detection,Attention mechanism,Adaptive velocity attribute representation learning,Unet
AI 理解论文
溯源树
样例
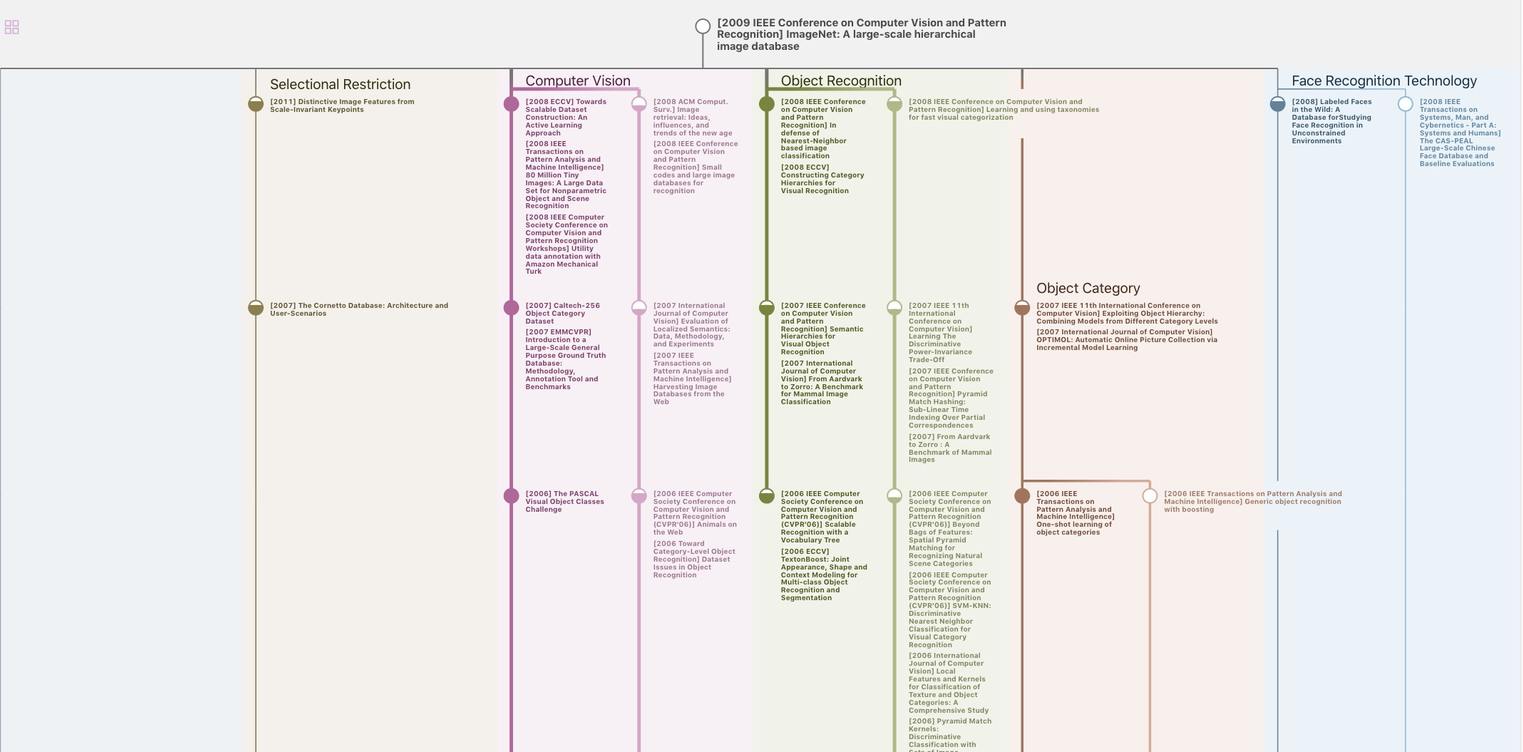
生成溯源树,研究论文发展脉络
Chat Paper
正在生成论文摘要