Cosmological Prediction of the CSST Ultra Deep Field Type Ia Supernova Photometric Survey
Monthly Notices of the Royal Astronomical Society(2024)
摘要
Type Ia supernova (SN Ia) as a standard candle is an ideal tool to measure
cosmic distance and expansion history of the Universe. Here we investigate the
SN Ia photometric measurement in the China Space Station Telescope Ultra Deep
Field (CSST-UDF) survey, and study the constraint power on the cosmological
parameters, such as the equation of state of dark energy. The CSST-UDF survey
is expected to cover a 9 deg^2 sky area in two years with 60 exposures. The
magnitude limit can reach i≃26 AB mag for 5σ point source
detection. We generate light curve mock data for SNe Ia and different types of
core-collapse supernovae (CCSNe), based on the relevant SN SED templates,
natural generation rates, luminosity functions, CSST instrumental design and
survey strategy. After selecting high-quality data and fitting the light
curves, we derive the light curve parameters and identify CCSNe as
contamination, resulting in ∼2200 SNe with a ∼7% CCSN contamination
rate. We adopt a calibration method similar to Chauvenet's criterion, and apply
it to the distance modulus data to further reduce the contamination. We find
that this method is effective and can suppress the contamination fraction to
∼3.5% with 2012 SNe Ia and 73 CCSNe. About 16% of SNe Ia are at z>1 in
the final CSST-UDF SN sample. By checking the cosmological constraints, the
result derived from this calibrated SN sample is in good agreement with that
using the pure SN Ia sample. The constraint accuracies on Ω_ M,
Ω_Λ and w are about 10%∼20%, which is about two times
better than the current SN surveys, and it could be further improved by a
factor of ∼1.4 if including the baryon acoustic oscillation (BAO) data
from the CSST spectroscopic survey. This indicates that CSST can provide
accurate measurements for the cosmic expansion history and the nature of dark
energy.
更多查看译文
AI 理解论文
溯源树
样例
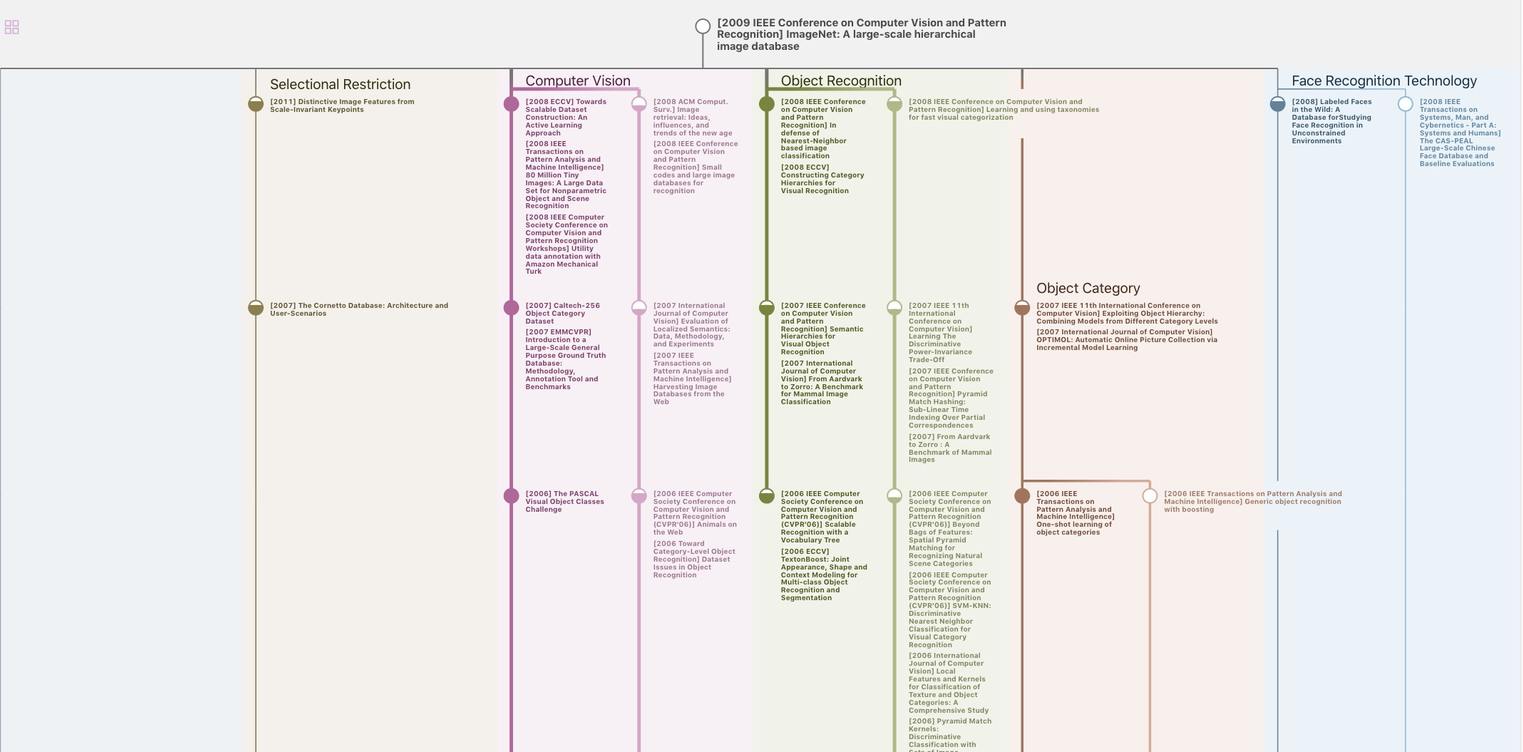
生成溯源树,研究论文发展脉络
Chat Paper
正在生成论文摘要