Bidformer: A Transformer-Based Model via Bidirectional Sparse Self-Attention Mechanism for Long Sequence Time-Series Forecasting.
2023 IEEE International Conference on Systems, Man, and Cybernetics (SMC)(2023)
摘要
Long Sequence Time-Series Forecasting (LSTF) is an important and challenging research with broad applications. Recent studies have shown that Transformer-based models can be effective in solving correlation problems in time-series data, but they also introduce issues of quadratic time and memory complexity, which make them unsuitable for LSTF problems. As a response, we investigate the impact of the long-tail distribution of attention scores on prediction accuracy and propose a Bis-Attention mechanism based on the mean measurement to bi-directionally sparse the self-attention matrix as a way to enhance the differentiation of attention scores and to reduce the complexity of the Transformer-based models from
$O(L^{2})$
to
$O((logL)^{2})$
. Moreover, we reduce memory consumption and optimize the model architecture through the use of a shared-QK method. The effectiveness of the proposed method is verified by theoretical analysis and visualisation. Extensive experiments on three benchmarks demonstrate that our method achieves better performance compared to other state-of-the-art methods, including an average reduction of 19.2% in MSE and 12% in MAE compared to Informer.
更多查看译文
关键词
Self-attention Mechanism,Time Series Data,Time Complexity,Space Complexity,Memory Consumption,Long-tailed Distribution,Time And Space,Deep Neural Network,Power Calculation,Recurrent Neural Network,Attention Mechanism,Kernel Function,Kullback-Leibler,Taylor Series,Dot Product,Memory Usage,Sparse Measurements,Average Mean Absolute Error,RNN-based Models
AI 理解论文
溯源树
样例
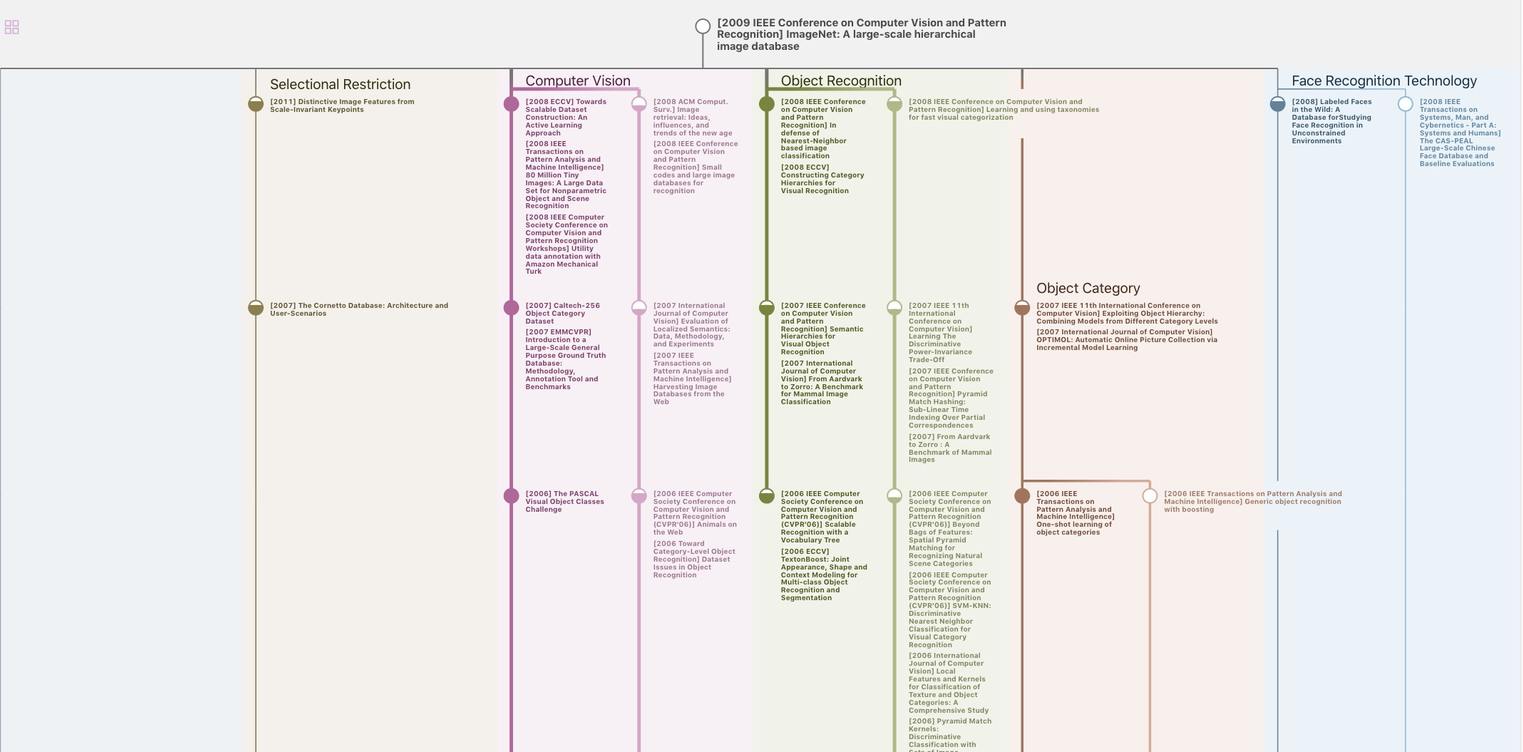
生成溯源树,研究论文发展脉络
Chat Paper
正在生成论文摘要